A Hybrid Intrusion Detection with Decision Tree for Feature Selection
arXiv (Cornell University)(2020)
摘要
Due to the size and nature of intrusion detection datasets, intrusion detection systems (IDS) typically take high computational complexity to examine features of data and identify intrusive patterns. Data preprocessing techniques such as feature selection can be used to reduce such complexity by eliminating irrelevant and redundant features in the dataset. The objective of this study is to analyze the efficiency and effectiveness of some feature selection approaches namely, wrapper-based and filter-based modeling approaches. To achieve that, a hybrid of feature selection algorithm in combination with wrapper and filter selection processes is designed. We propose a wrapper-based hybrid intrusion detection modeling with a decision tree algorithm to guide the selection process. Five machine learning algorithms are used on the wrapper and filter-based feature selection methods to build IDS models using the UNSW-NB15 dataset. The three filter-based methods namely, information gain, gain ratio, and relief are used for comparison to determine the efficiency and effectiveness of the proposed approach. Furthermore, a fair comparison with other state-of-the-art intrusion detection approaches is also performed. The experimental results show that our approach is quite effective in comparison to state-of-the-art works, however, it takes high computational time in comparison to the filter-based methods whilst achieves similar results. Our work also revealed unobserved issues about the conformity of the UNSW-NB15 dataset.
更多查看译文
关键词
hybrid intrusion detection,feature selection,decision tree
AI 理解论文
溯源树
样例
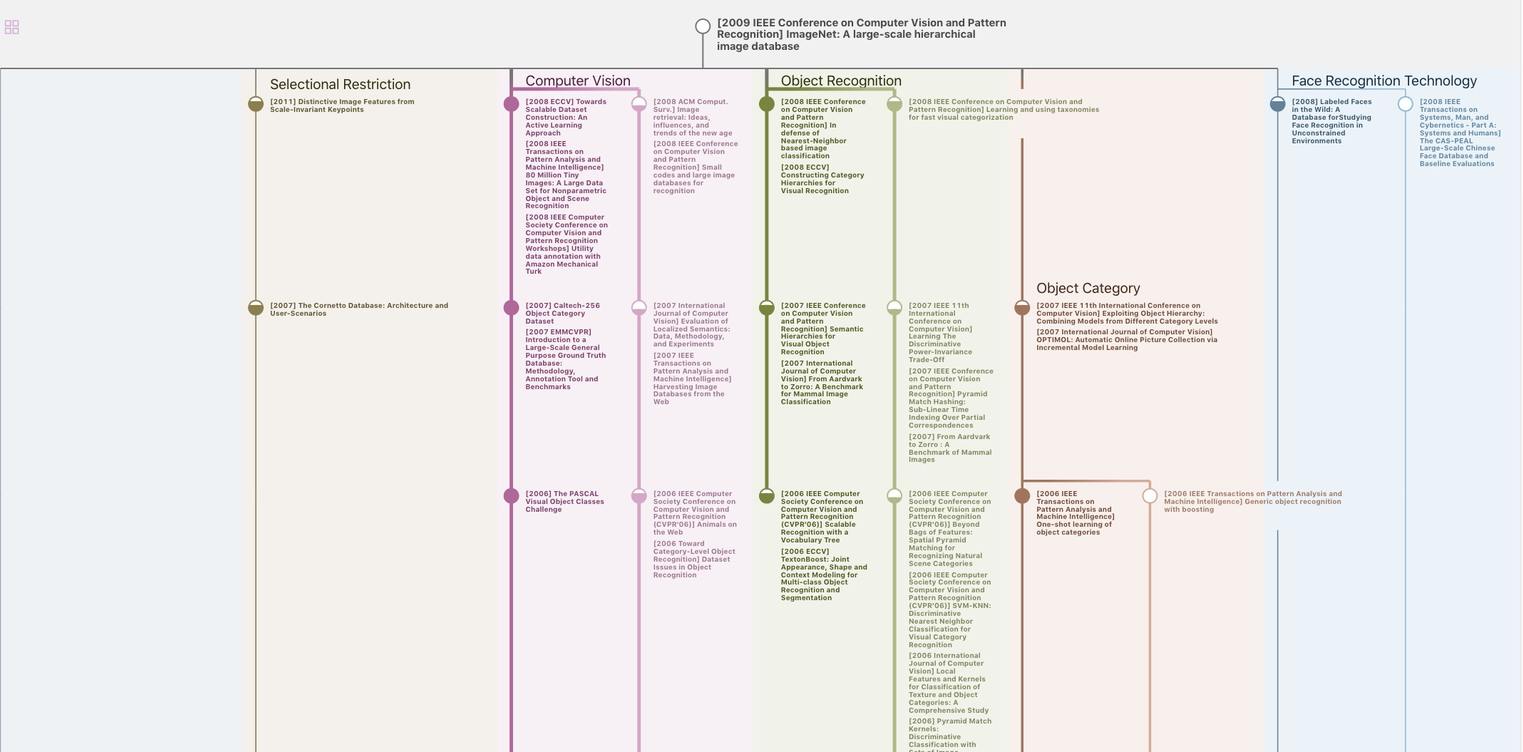
生成溯源树,研究论文发展脉络
Chat Paper
正在生成论文摘要