$\epsilon$-net Induced Lazy Witness Complexes on Graphs
arxiv(2020)
摘要
Computation of persistent homology of simplicial representations such as the Rips and the C\v{e}ch complexes do not efficiently scale to large point clouds. It is, therefore, meaningful to devise approximate representations and evaluate the trade-off between their efficiency and effectiveness. The lazy witness complex economically defines such a representation using only a few selected points, called landmarks. Topological data analysis traditionally considers a point cloud in a Euclidean space. In many situations, however, data is available in the form of a weighted graph. A graph along with the geodesic distance defines a metric space. This metric space of a graph is amenable to topological data analysis. We discuss the computation of persistent homologies on a weighted graph. We present a lazy witness complex approach leveraging the notion of $\epsilon$-net that we adapt to weighted graphs and their geodesic distance to select landmarks. We show that the value of the $\epsilon$ parameter of the $\epsilon$-net provides control on the trade-off between choice and number of landmarks and the quality of the approximate simplicial representation. We present three algorithms for constructing an $\epsilon$-net of a graph. We comparatively and empirically evaluate the efficiency and effectiveness of the choice of landmarks that they induce for the topological data analysis of different real-world graphs.
更多查看译文
关键词
complexes,graphs
AI 理解论文
溯源树
样例
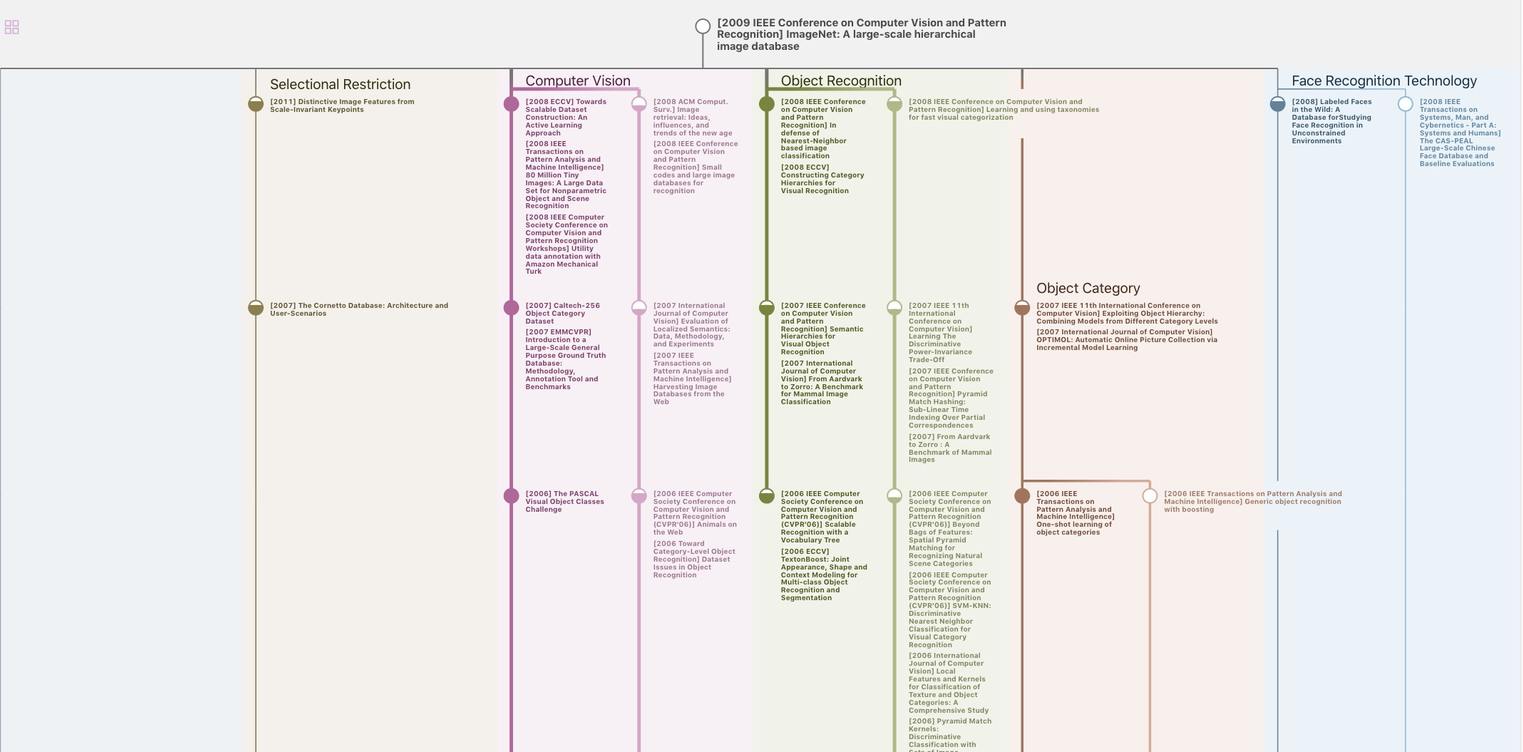
生成溯源树,研究论文发展脉络
Chat Paper
正在生成论文摘要