Convergence of gradient-based block coordinate descent algorithms for nonorthogonal joint approximate diagonalization of matrices
SIAM JOURNAL ON MATRIX ANALYSIS AND APPLICATIONS(2023)
摘要
We propose a gradient-based block coordinate descent (BCD-G) framework to solve the joint approximate diagonalization of matrices defined on the product of the complex Stiefel manifold and the special linear group. Instead of the cyclic fashion, we choose a block optimization based on the Riemannian gradient. To update the first block variable in the complex Stiefel manifold, we use the well-known line search descent method. To update the second block variable in the special linear group, based on four different kinds of elementary transformations, we construct three classes, GLU, GQU and GU, and then get three BCD-G algorithms, BCD-GLU, BCD-GQU and BCD -GU. We establish the global convergence and weak convergence of these three algorithms using the \Lojasiewicz gradient inequality under the assumption that the iterates are bounded. We also propose a gradient-based Jacobi-type framework to solve the joint approximate diagonalization of matrices defined on the special linear group. As in the BCD-G case, using the GLU and GQU classes of elementary transformations, we focus on the Jacobi-GLU and Jacobi-GQU algorithms and establish their global convergence and weak convergence as well. All the algorithms and convergence results described in this paper also apply to the real case.
更多查看译文
关键词
blind source separation, joint approximate diagonalization of matrices, block coordinate descent, Jacobi-G algorithm, convergence analysis, manifold optimization
AI 理解论文
溯源树
样例
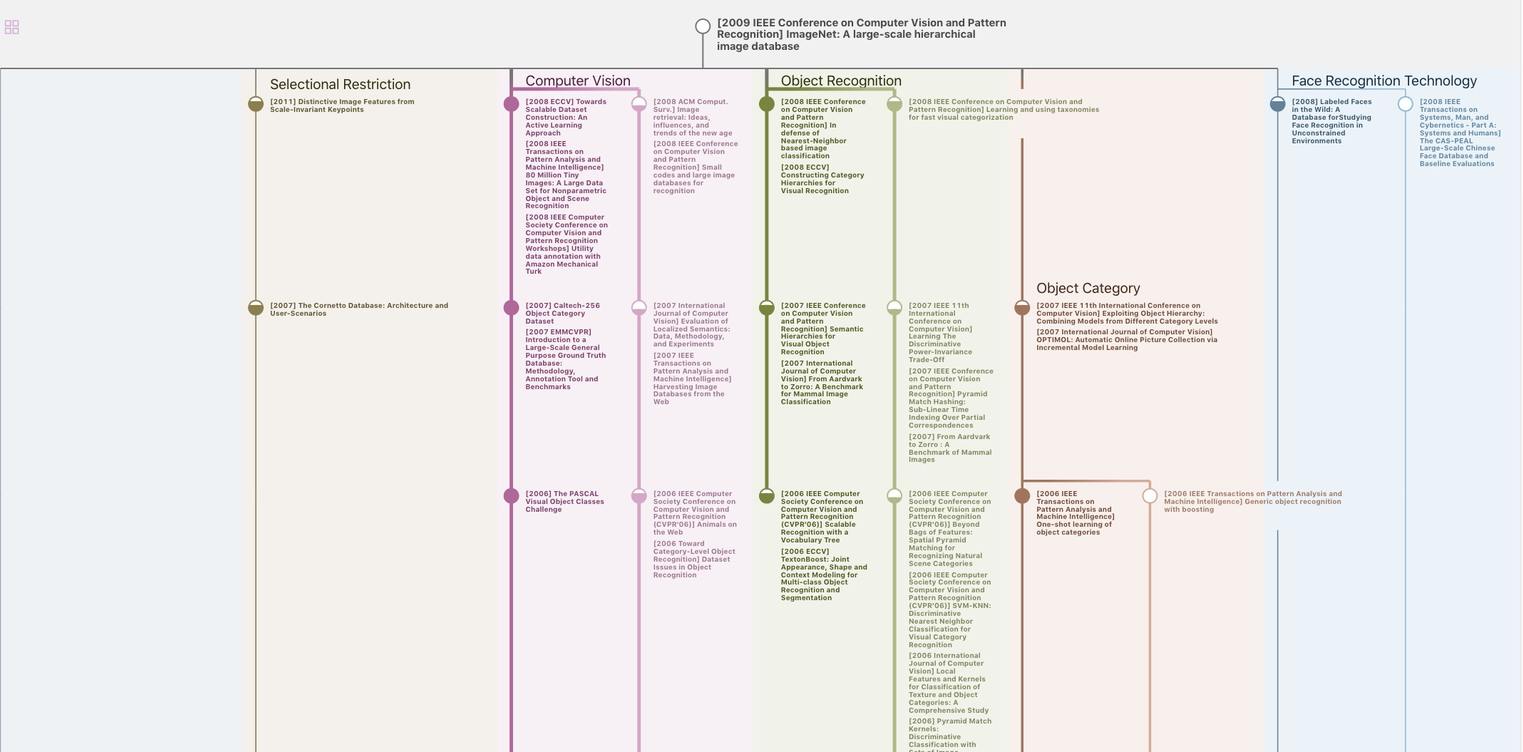
生成溯源树,研究论文发展脉络
Chat Paper
正在生成论文摘要