Five-institution study of automated classification of pathological slowing from adult scalp electroencephalograms
arXiv (Cornell University)(2020)
摘要
Pathological slowing in the electroencephalogram (EEG) is widely investigated for the diagnosis of neurological disorders. Currently, the gold standard for slowing detection is the visual inspection of the EEG by experts. However, visual inspection is time-consuming and subjective. Moreover, there is shortage of EEG experts worldwide. To address those issues, we propose three automated approaches to detect slowing in EEG: unsupervised learning system (ULS), supervised shallow learning system (SSLS), and supervised deep learning system (SDLS). These systems are evaluated on single-channel segments (channel-level), multi-channel segments (segment-level), and entire EEGs (EEG-level). The ULS performs prediction via spectrum features. By contrast, the SSLS and SDLS detect slowing at individual channels via a channel-level slowing detector, and leverage the channel-level detections for detections on the level of segments and full EEGs. We evaluate the systems through Leave-One-Subject-Out (LOSO) cross-validation (CV) and Leave-One-Institution-Out (LOIO) CV on four datasets from the US, Singapore, and India. The SDLS achieved the best overall results: LOIO CV mean balanced accuracy (BAC) of 71.9%, 75.5%, and 82.0% at channel-, segment- and EEG-level, and LOSO CV mean BAC of 73.6%, 77.2%, and 81.8% at channel-, segment-, and EEG-level. The channel- and segment-level performance is comparable to the intra-rater agreement (IRA) of an expert of 72.4% and 82%. The SDLS can process a 30-minutes EEG in 4 seconds, and may be deployed to assist clinicians in interpreting EEGs.
更多查看译文
关键词
pathological slowing,five-institution
AI 理解论文
溯源树
样例
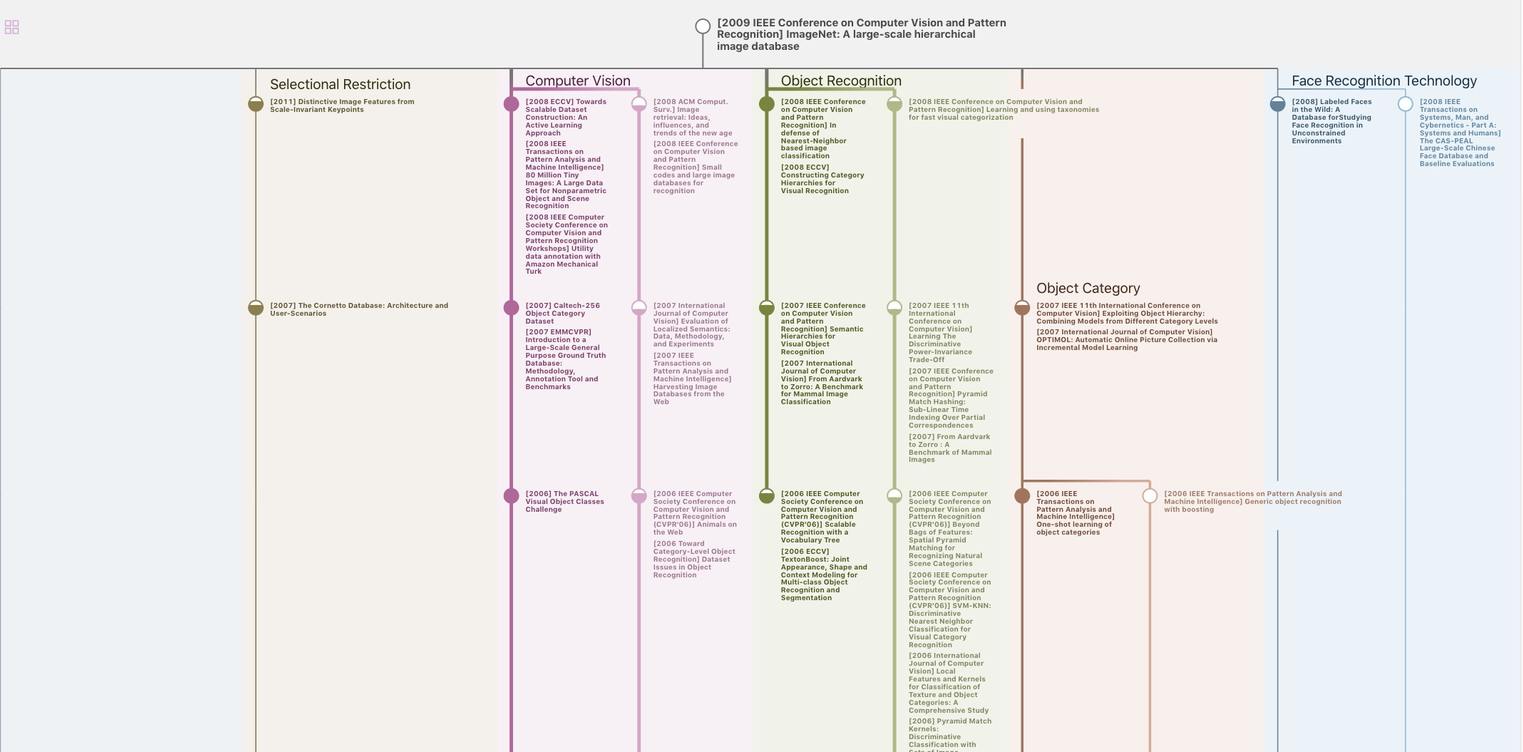
生成溯源树,研究论文发展脉络
Chat Paper
正在生成论文摘要