GraPPa: Grammar-Augmented Pre-Training for Table Semantic Parsing
ICLR(2021)
Abstract
We present GraPPa, an effective pre-training approach for table semantic parsing that learns a compositional inductive bias in the joint representations of textual and tabular data. We construct synthetic question-SQL pairs over high-quality tables via a synchronous context-free grammar (SCFG). We pre-train our model on the synthetic data to inject important structural properties commonly found in semantic parsing into the pre-training language model. To maintain the model\u0027s ability to represent real-world data, we also include masked language modeling (MLM) on several existing table-related datasets to regularize our pre-training process. Our proposed pre-training strategy is much data-efficient. When incorporated with strong base semantic parsers, GraPPa achieves new state-of-the-art results on four popular fully supervised and weakly supervised table semantic parsing tasks.
MoreTranslated text
Key words
semantic parsing,table,grammar-augmented,pre-training
AI Read Science
Must-Reading Tree
Example
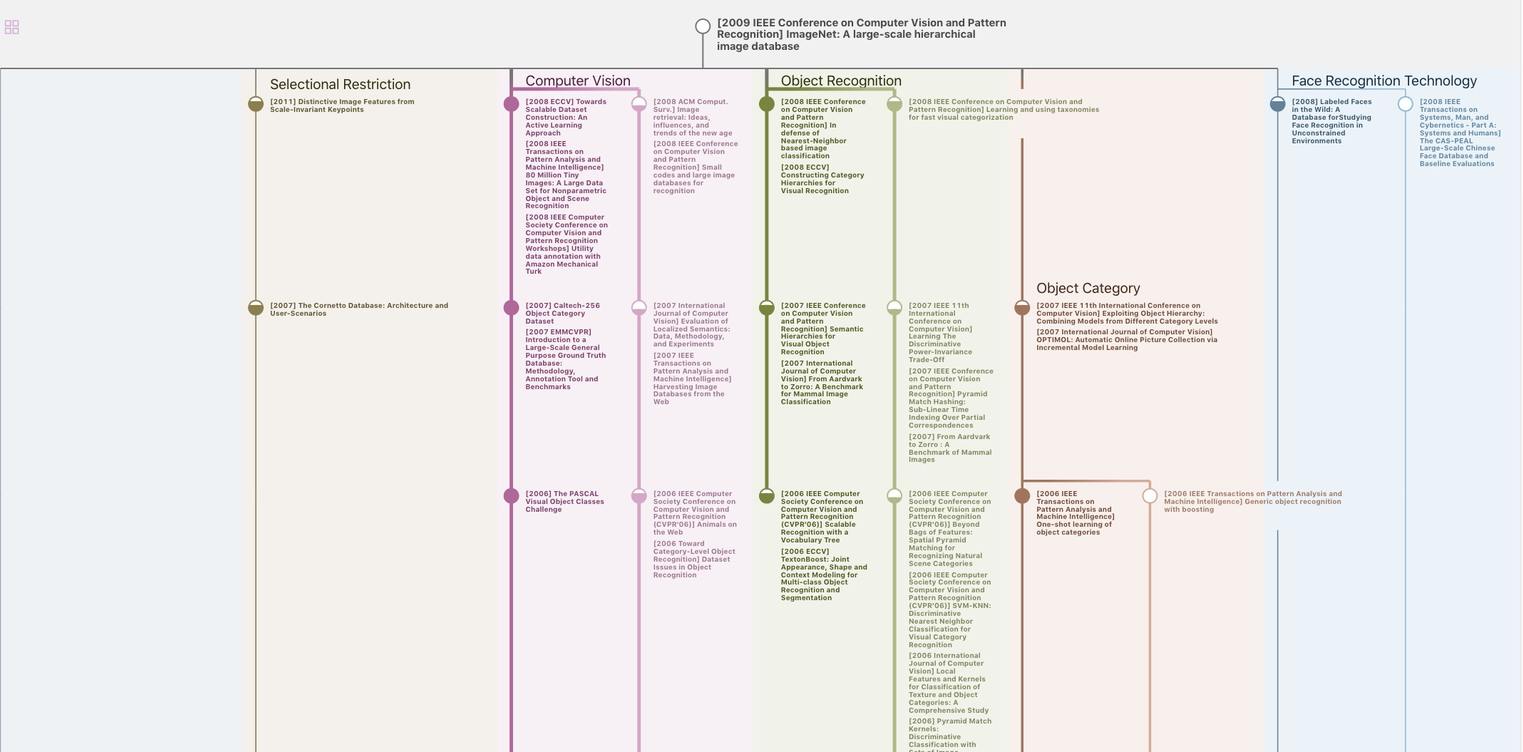
Generate MRT to find the research sequence of this paper
Chat Paper
Summary is being generated by the instructions you defined