Low-Rank Subspace Representation from Optimal Coded-Aperture for Unsupervised Classification of Hypersepctral Imagery
ICIP(2020)
摘要
This paper aims at developing a clustering approach with spectral images directly from the compressive measurements of coded aperture snapshot spectral imager (CASSI). Assuming that compressed measurements often lie approximately in low dimensional subspaces corresponding to multiple classes, state of the art methods generally obtains optimal solution for each step separately but cannot guarantee that it will achieve the globally optimal clustering results. In this paper, a low-rank subspace representation (LRSR) algorithm is proposed to perform clustering on the compressed measurements. In addition, a subspace structured norm is added into the objective of low-rank representation problem exploiting the fact that each point in a union of subspaces can be expressed as a sparse linear combination of all other points and that the matrix of the points within each subspace is low rank. Simulation with real dataset illustrates the accuracy of the proposed spectral image clustering approach.
更多查看译文
关键词
CASSI,low-rank subspace representation (LRSR),spectral image clustering
AI 理解论文
溯源树
样例
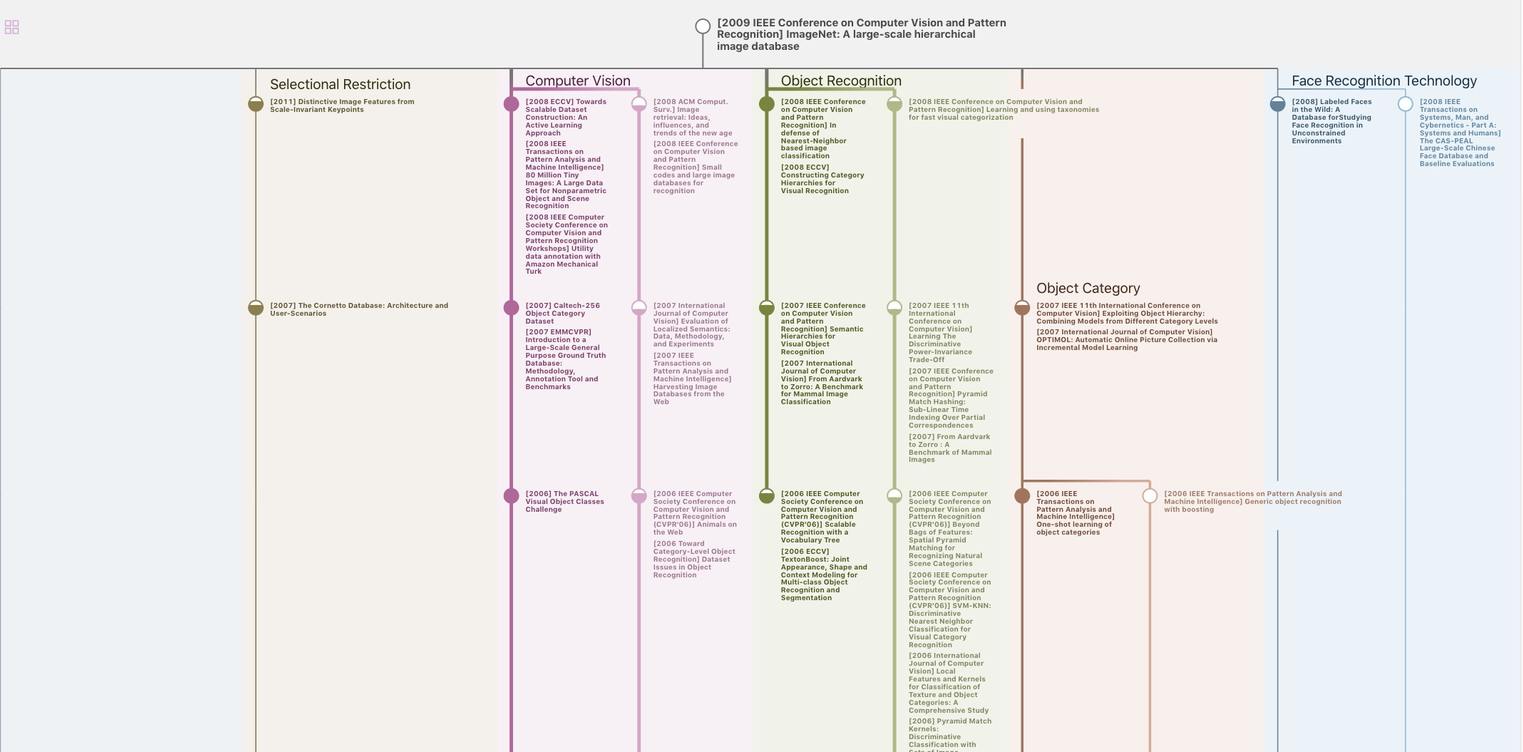
生成溯源树,研究论文发展脉络
Chat Paper
正在生成论文摘要