Utilizing Transfer Learning and a Customized Loss Function for Optic Disc Segmentation from Retinal Images.
ACCV (5)(2020)
摘要
Accurate segmentation of the optic disc from a retinal image is vital to extracting retinal features that may be highly correlated with retinal conditions such as glaucoma. In this paper, we propose a deep-learning based approach capable of segmenting the optic disc given a high-precision retinal fundus image. Our approach utilizes a UNET-based model with a VGG16 encoder trained on the ImageNet dataset. This study can be distinguished from other studies in the customization made for the VGG16 model, the diversity of the datasets adopted, the duration of disc segmentation, the loss function utilized, and the number of parameters required to train our model. Our approach was tested on seven publicly available datasets augmented by a dataset from a private clinic that was annotated by two Doctors of Optometry through a web portal built for this purpose. We achieved an accuracy of 99.78\% and a Dice coefficient of 94.73\% for a disc segmentation from a retinal image in 0.03 seconds. The results obtained from comprehensive experiments demonstrate the robustness of our approach to disc segmentation of retinal images obtained from different sources.
更多查看译文
关键词
optic disc segmentation,transfer learning,customized loss function
AI 理解论文
溯源树
样例
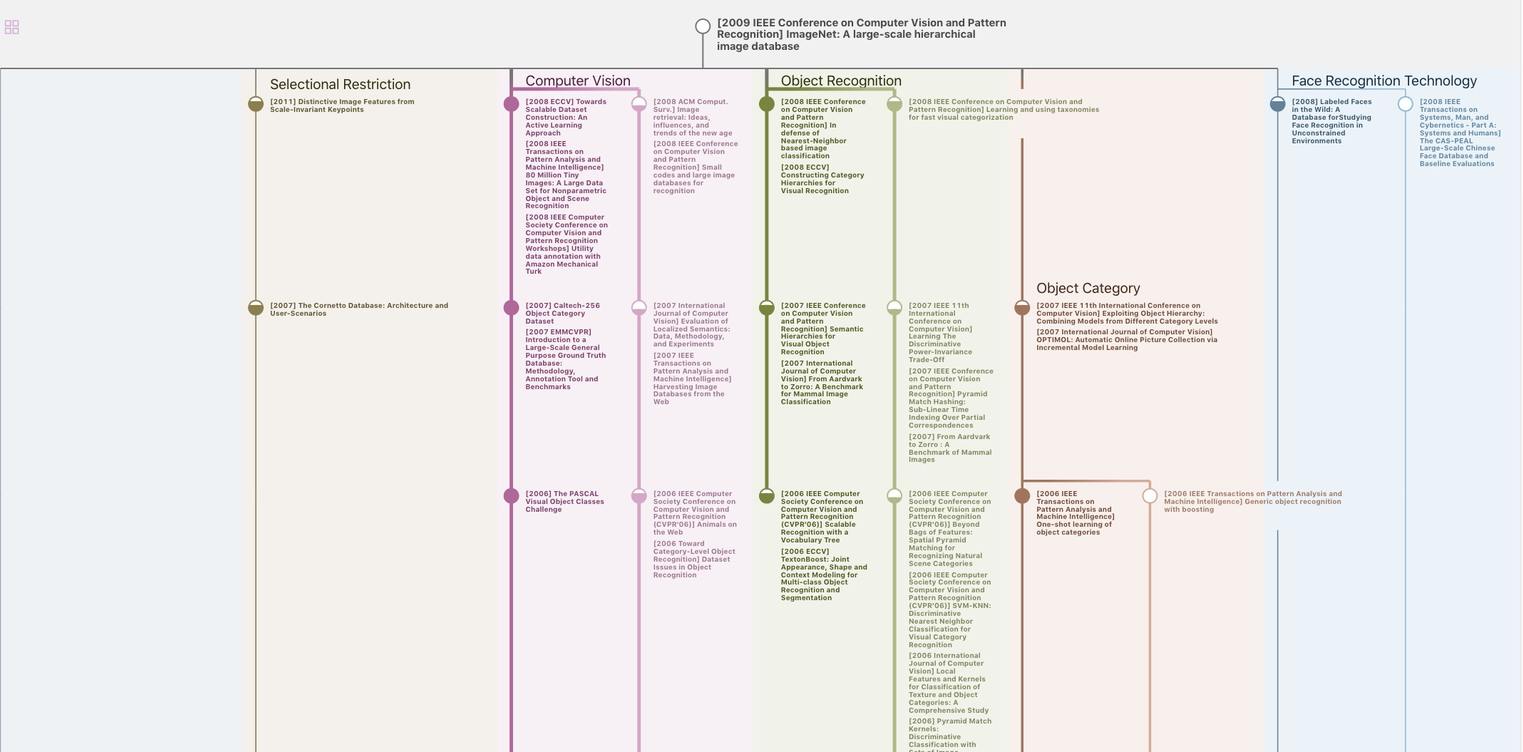
生成溯源树,研究论文发展脉络
Chat Paper
正在生成论文摘要