A spectral clustering with self-weighted multiple kernel learning method for single-cell RNA-seq data
BRIEFINGS IN BIOINFORMATICS(2021)
摘要
Single-cell RNA-sequencing (scRNA-seq) data widely exist in bioinformatics. It is crucial to devise a distance metric for scRNA-seq data. Almost all existing clustering methods based on spectral clustering algorithms work in three separate steps: similarity graph construction; continuous labels learning; discretization of the learned labels by k-means clustering. However, this common practice has potential flaws that may lead to severe information loss and degradation of performance. Furthermore, the performance of a kernel method is largely determined by the selected kernel; a self-weighted multiple kernel learning model can help choose the most suitable kernel for scRNA-seq data. To this end, we propose to automatically learn similarity information from data. We present a new clustering method in the form of a multiple kernel combination that can directly discover groupings in scRNA-seq data. The main proposition is that automatically learned similarity information from scRNA-seq data is used to transform the candidate solution into a new solution that better approximates the discrete one. The proposed model can be efficiently solved by the standard support vector machine (SVM) solvers. Experiments on benchmark scRNA-Seq data validate the superior performance of the proposed model. Spectral clustering with multiple kernels is implemented in Matlab, licensed under Massachusetts Institute of Technology (MIT) and freely available from the Github website, https://github.com/Cuteu/SMSC/.
更多查看译文
关键词
scRNA-Seq, spectral clustering, multiple kernel learning, self-weighted, cell clustering
AI 理解论文
溯源树
样例
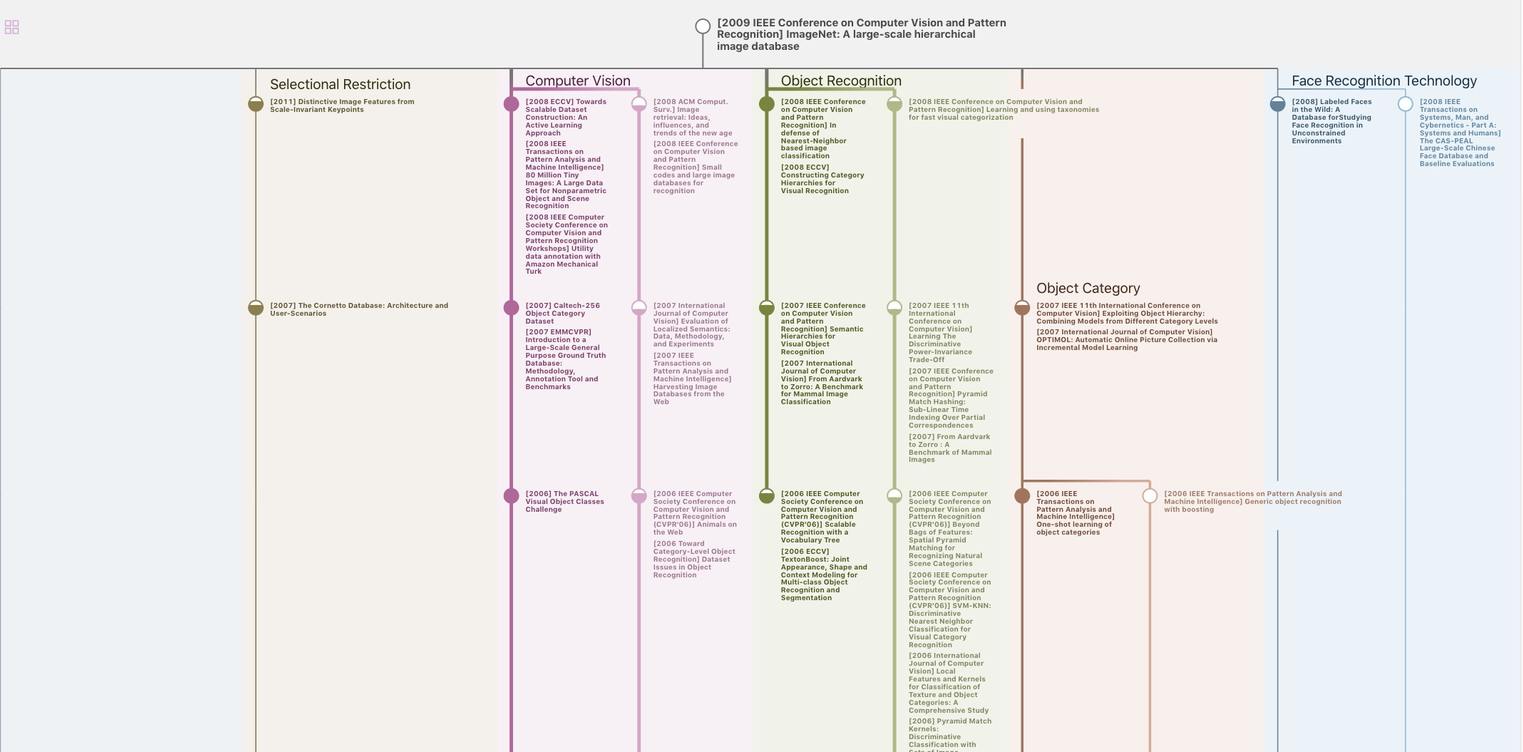
生成溯源树,研究论文发展脉络
Chat Paper
正在生成论文摘要