A Machine-Learning Tool Concurrently Models Single Omics and Phenome Data for Functional Subtyping and Personalized Cancer Medicine.
CANCERS(2020)
摘要
Simple Summary: Tumours are heterogeneous that reflect variable patient prognosis and treatment responses (phenotypes). Since these variable phenotypes are outcomes of genomics, it is essential to integrate genome and phenome jointly. In this study, we report the development and application of a new machine learning tool (Phenotypic Mapping; PhenMap) to identify unsupervised clinically-relevant (functional) subtypes and biomarkers by simultaneously integrating clinical phenotypes and single omics data, mainly, transcriptome. This integrative analysis provides the opportunity to simultaneously identify robust and context-specific subtypes and associated phenotypes (that biologically explain each subtype) without statistically losing information. We demonstrate its utility using breast cancer cell lines and patient samples to identify functional subtypes associated with specific drug responses (including CD4/6 inhibitor) and prognosis. These subtypes may potentially predict clinical outcomes with further validation. This tool can be applied to other omics data such as methylomics, genomics and radiomics along with any phenotypic data. One of the major challenges in defining clinically-relevant and less heterogeneous tumor subtypes is assigning biological and/or clinical interpretations to etiological (intrinsic) subtypes. Conventional clustering/subtyping approaches often fail to define such subtypes, as they involve several discrete steps. Here we demonstrate a unique machine-learning method, phenotype mapping (PhenMap), which jointly integrates single omics data with phenotypic information using three published breast cancer datasets (n = 2045). The PhenMap framework uses a modified factor analysis method that is governed by a key assumption that, features from different omics data types are correlated due to specific "hidden/mapping" variables (context-specific mapping variables (CMV)). These variables can be simultaneously modeled with phenotypic data as covariates to yield functional subtypes and their associated features (e.g., genes) and phenotypes. In one example, we demonstrate the identification and validation of six novel "functional" (discrete) subtypes with differential responses to a cyclin-dependent kinase (CDK)4/6 inhibitor and etoposide by jointly integrating transcriptome profiles with four different drug response data from 37 breast cancer cell lines. These robust subtypes are also present in patient breast tumors with different prognosis. In another example, we modeled patient gene expression profiles and clinical covariates together to identify continuous subtypes with clinical/biological implications. Overall, this genome-phenome machine-learning integration tool, PhenMap identifies functional and phenotype-integrated discrete or continuous subtypes with clinical translational potential.
更多查看译文
关键词
machine learning,breast cancer,continuous subtypes,functional subtypes,CDK inhibitor,etoposide,subtyping,genome-phenome integration,phenotypes,dimension reduction methods
AI 理解论文
溯源树
样例
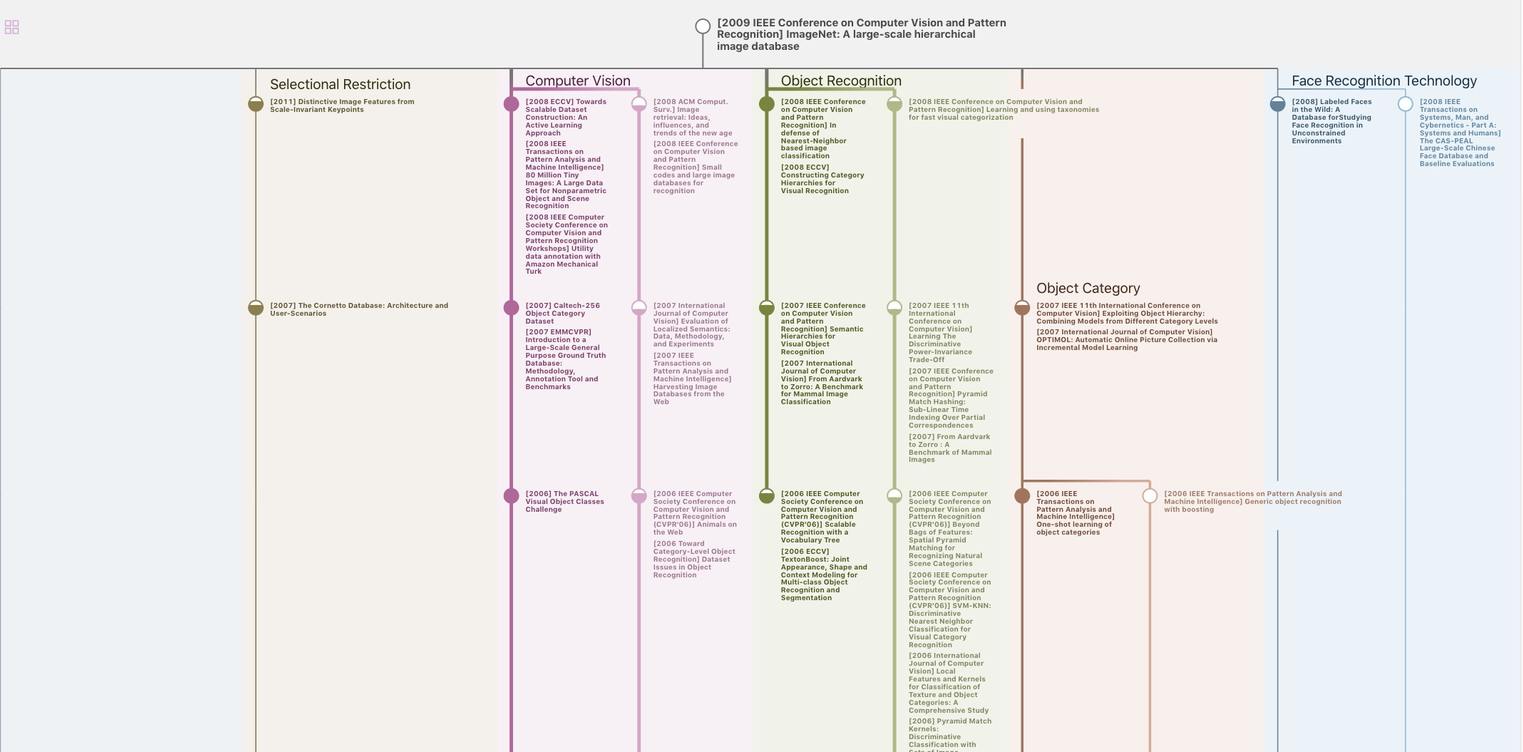
生成溯源树,研究论文发展脉络
Chat Paper
正在生成论文摘要