The All Babies Count Initiative: Impact Of A Health System Improvement Approach On Neonatal Care And Outcomes In Rwanda
GLOBAL HEALTH-SCIENCE AND PRACTICE(2020)
摘要
Introduction: Poor-quality care contributes to a significant portion of neonatal deaths globally. The All Babies Count (ABC) initiative was an 18-month district-wide approach designed to improve clinical and system performance across 2 rural Rwandan districts.Methods: This pre-post intervention study measured change in maternal and newborn health (MNH) quality of care and neonatal mortality. Data from the facility and community health management information system and newly introduced indicators were extracted from facility registers. Medians and interquartile ranges were calculated for the health facility to assess changes over time, and a mixed-effects logistic regression model was created for neonatal mortality. A difference-in-differences analysis was conducted to compare the change in district neonatal mortality with the rest of rural Rwanda.Results: Improvements were seen in multiple measures of facility readiness and MNH quality of care, including antenatal care coverage, preterm labor management, and postnatal care quality. District hospital case fatality decreased, with a statistically significant reduction in district neonatal mortality (odds ratio [OR]=0.54; 95% confidence interval [CI]=0.36, 0.83) and among preterm/low birth weight neonates (OR=0.47; 95% CI=0.25, 0.90). Neonatal mortality was reduced from 30.1 to 19.6 deaths/1,000 live births in the intervention districts and remained relatively stable in the rest of rural Rwanda (difference in differences similar to 12.9).Conclusion: The ABC initiative contributed to improved MNH quality of care and outcomes in rural Rwanda. A combined clinical and health system improvement approach could be an effective strategy to improve quality and reduce neonatal mortality.
更多查看译文
AI 理解论文
溯源树
样例
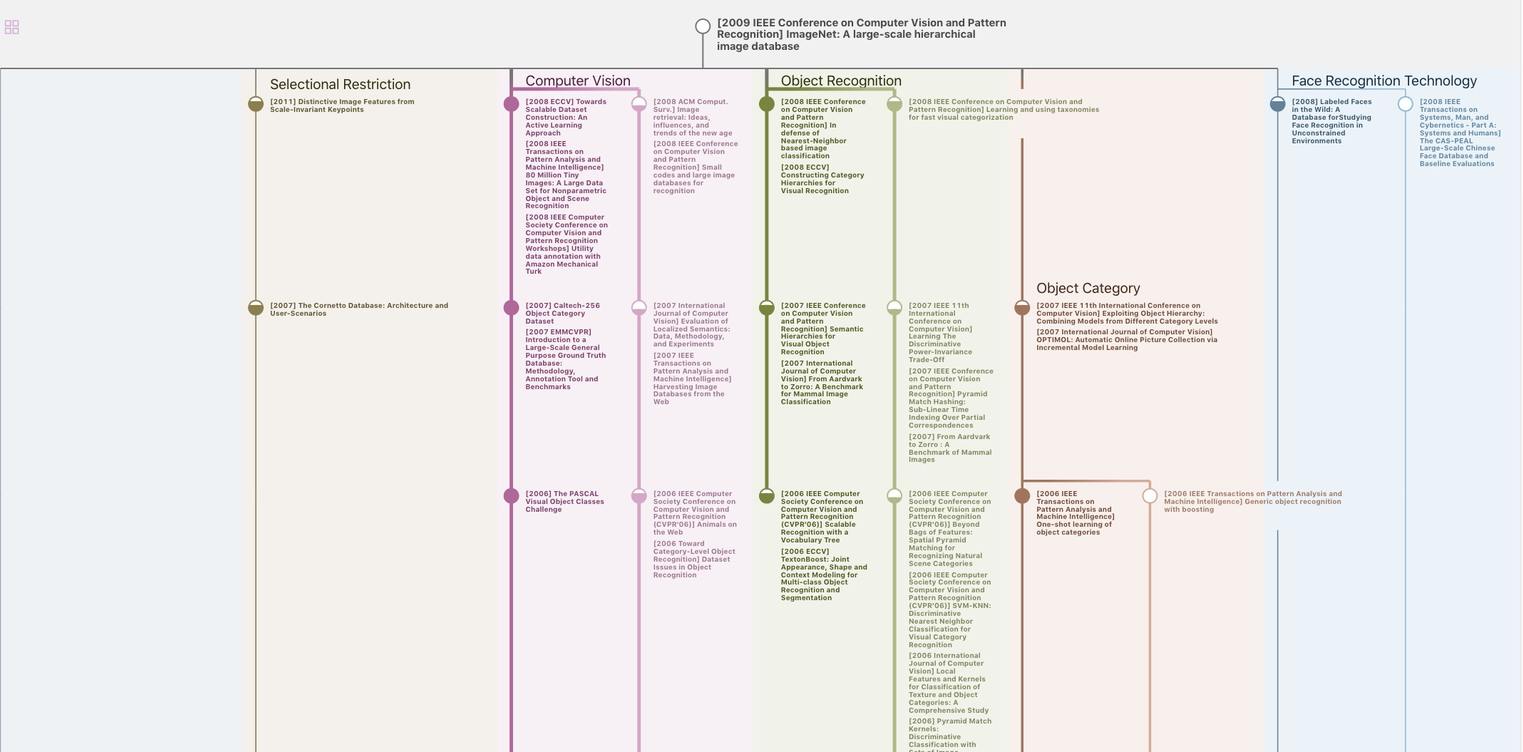
生成溯源树,研究论文发展脉络
Chat Paper
正在生成论文摘要