Combining GMM-Based Hidden Markov Random Field and Bag-of-Words Trained Classifier for Lung Cancer Detection Using Pap-Stained Microscopic Images
ADVANCED COMPUTATIONAL AND COMMUNICATION PARADIGMS, VOL 2(2018)
摘要
Lung cancer is a malignant tumour having uncontrolled lung cell growth. Papanicolaou (Pap)-stained cell cytology from Fine Needle Aspiration Cytology (FNAC) is the most followed approach for lung cancer diagnosis. However, the manual assessment of cytopathology slides under light microscopy is time consuming and suffers from feature ambiguities including inter-observer variability. Here, an automated computer vision approach is presented for identifying and classifying cancerous cell nuclei from pap-stained microscopic image of lung FNAC sample. The proposed methodology adopted Gaussian mixture model-based hidden Markov random field technique to segment cell nucleus. Later, bag-of-visual words model was used for nucleus classification, where scale-invariant feature transform feature were extracted from segmented nucleus for training a random forest classifier model. The adopted nucleus segmentation-cum-classification model was able to precisely segment the nucleus and classify them in two class, viz. Small Cell Lung Cancer (SCLC) and Non-small Cell Lung Cancer (NSCLC). The segmentation process achieves a sensitivity of 98.88% and specificity of 97.93%. And also, the nucleus classification model was able to perform with a sensitivity of 97.31%, specificity of 99.54%, and accuracy of 98.78%.
更多查看译文
关键词
Gaussian mixture model,Bag of words,Supervised classification,Nucleus segmentation,Lung cancer
AI 理解论文
溯源树
样例
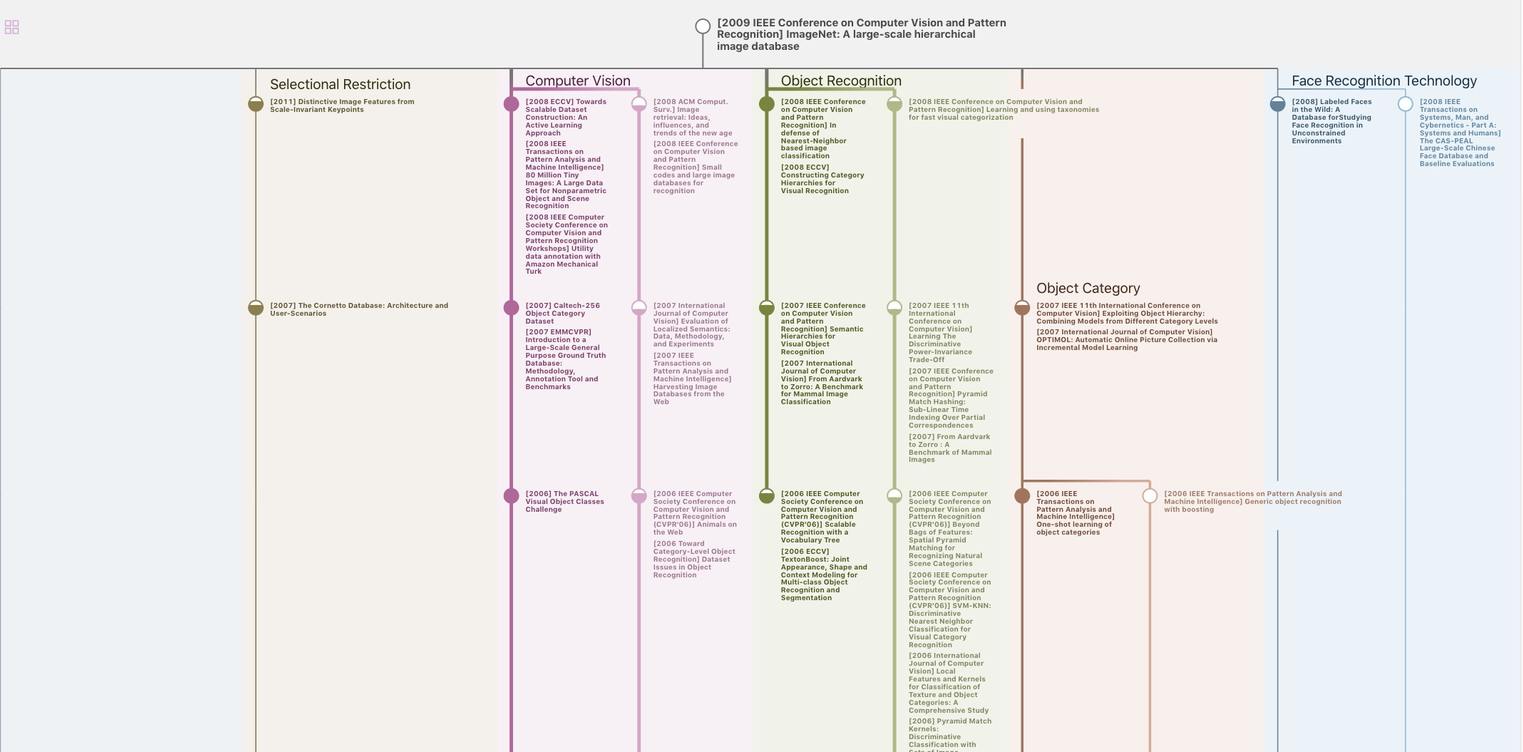
生成溯源树,研究论文发展脉络
Chat Paper
正在生成论文摘要