Transients generate memory and break hyperbolicity in stochastic enzymatic networks
JOURNAL OF CHEMICAL PHYSICS(2021)
摘要
The hyperbolic dependence of catalytic rate on substrate concentration is a classical result in enzyme kinetics, quantified by the celebrated Michaelis-Menten equation. The ubiquity of this relation in diverse chemical and biological contexts has recently been rationalized by a graph-theoretic analysis of deterministic reaction networks. Experiments, however, have revealed that "molecular noise"-intrinsic stochasticity at the molecular scale-leads to significant deviations from classical results and to unexpected effects like "molecular memory," i.e., the breakdown of statistical independence between turnover events. Here, we show, through a new method of analysis, that memory and non-hyperbolicity have a common source in an initial, and observably long, transient peculiar to stochastic reaction networks of multiple enzymes. Networks of single enzymes do not admit such transients. The transient yields, asymptotically, to a steady-state in which memory vanishes and hyperbolicity is recovered. We propose new statistical measures, defined in terms of turnover times, to distinguish between the transient and steady-states and apply these to experimental data from a landmark experiment that first observed molecular memory in a single enzyme with multiple binding sites. Our study shows that catalysis at the molecular level with more than one enzyme always contains a non-classical regime and provides insight on how the classical limit is attained.
更多查看译文
关键词
memory,hyperbolicity,networks
AI 理解论文
溯源树
样例
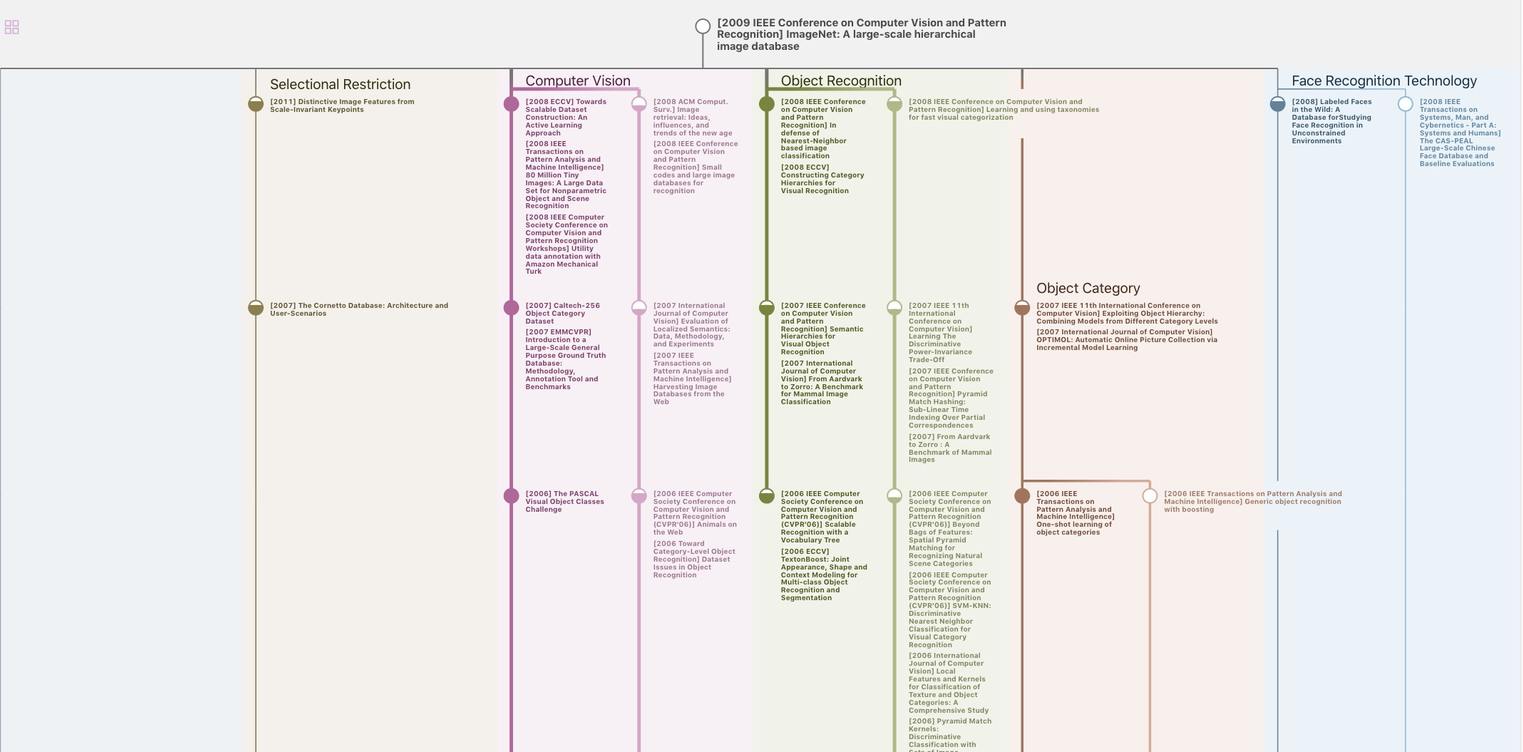
生成溯源树,研究论文发展脉络
Chat Paper
正在生成论文摘要