Model-Agnostic Round-Optimal Federated Learning via Knowledge Transfer
arxiv(2020)
摘要
Federated learning enables multiple parties to collaboratively learn a model without exchanging their local data. Currently, federated averaging (FedAvg) is the most widely used federated learning algorithm. However, FedAvg or its variants have obvious shortcomings. It can only be used to learn differentiable models and needs many communication rounds to converge. In this paper, we propose a novel federated learning algorithm FedKT that needs only a single communication round (i.e., round-optimal). With applying the knowledge transfer approach, our algorithm can be applied to any classification model. Moreover, we develop the differentially private versions of FedKT and theoretically analyze the privacy loss. The experiments show that our method can achieve close or better accuracy compared with the other state-of-the-art federated learning algorithms.
更多查看译文
关键词
knowledge transfer,learning,model-agnostic,round-optimal
AI 理解论文
溯源树
样例
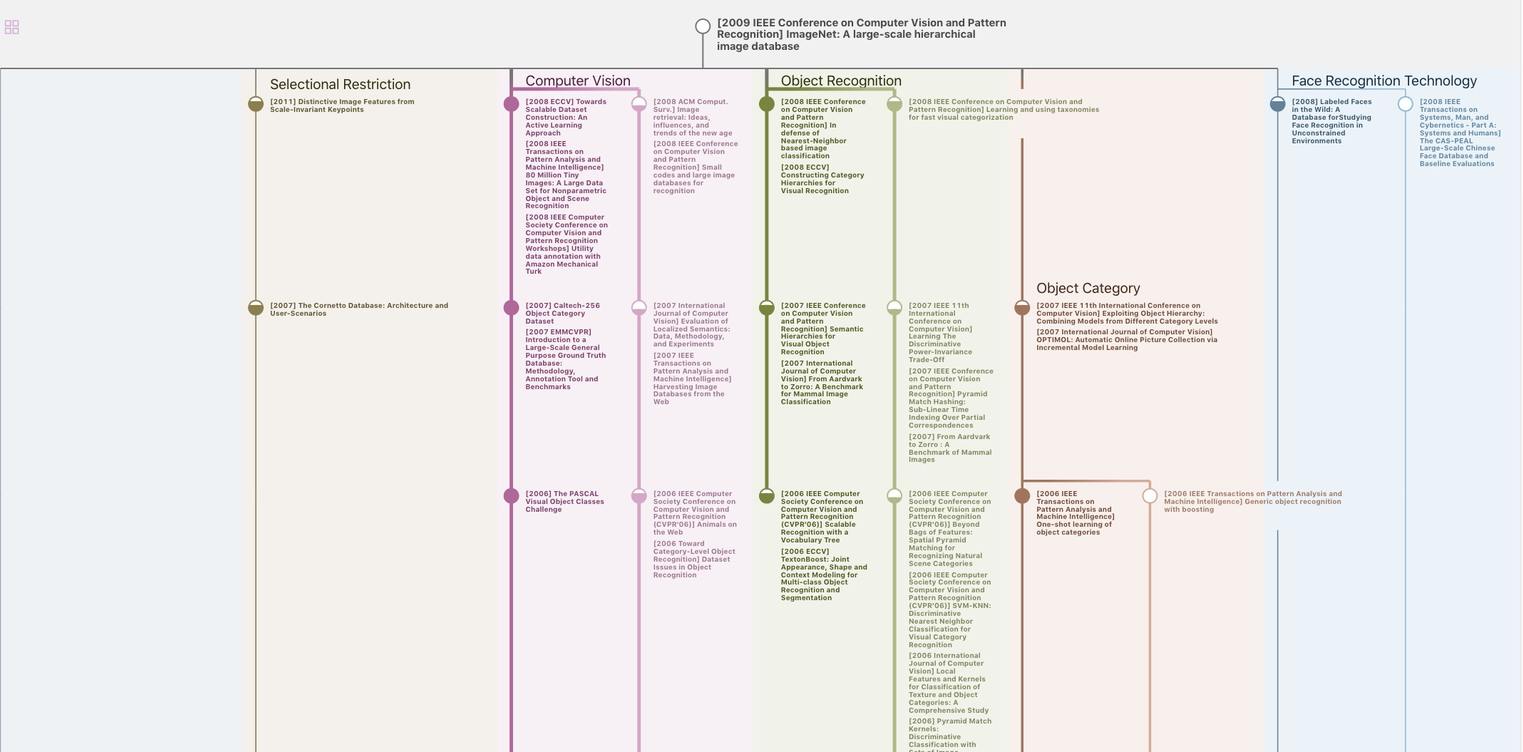
生成溯源树,研究论文发展脉络
Chat Paper
正在生成论文摘要