Client Selection in Federated Learning: Convergence Analysis and Power-of-Choice Selection Strategies
arxiv(2020)
摘要
Federated learning is a distributed optimization paradigm that enables a large number of resource-limited client nodes to cooperatively train a model without data sharing. Several works have analyzed the convergence of federated learning by accounting of data heterogeneity, communication and computation limitations, and partial client participation. However, they assume unbiased client participation, where clients are selected at random or in proportion of their data sizes. In this paper, we present the first convergence analysis of federated optimization for biased client selection strategies, and quantify how the selection bias affects convergence speed. We reveal that biasing client selection towards clients with higher local loss achieves faster error convergence. Using this insight, we propose Power-of-Choice, a communication- and computation-efficient client selection framework that can flexibly span the trade-off between convergence speed and solution bias. Our experiments demonstrate that Power-of-Choice strategies converge up to 3 $\times$ faster and give $10$% higher test accuracy than the baseline random selection.
更多查看译文
关键词
federated learning,selection,power-of-choice
AI 理解论文
溯源树
样例
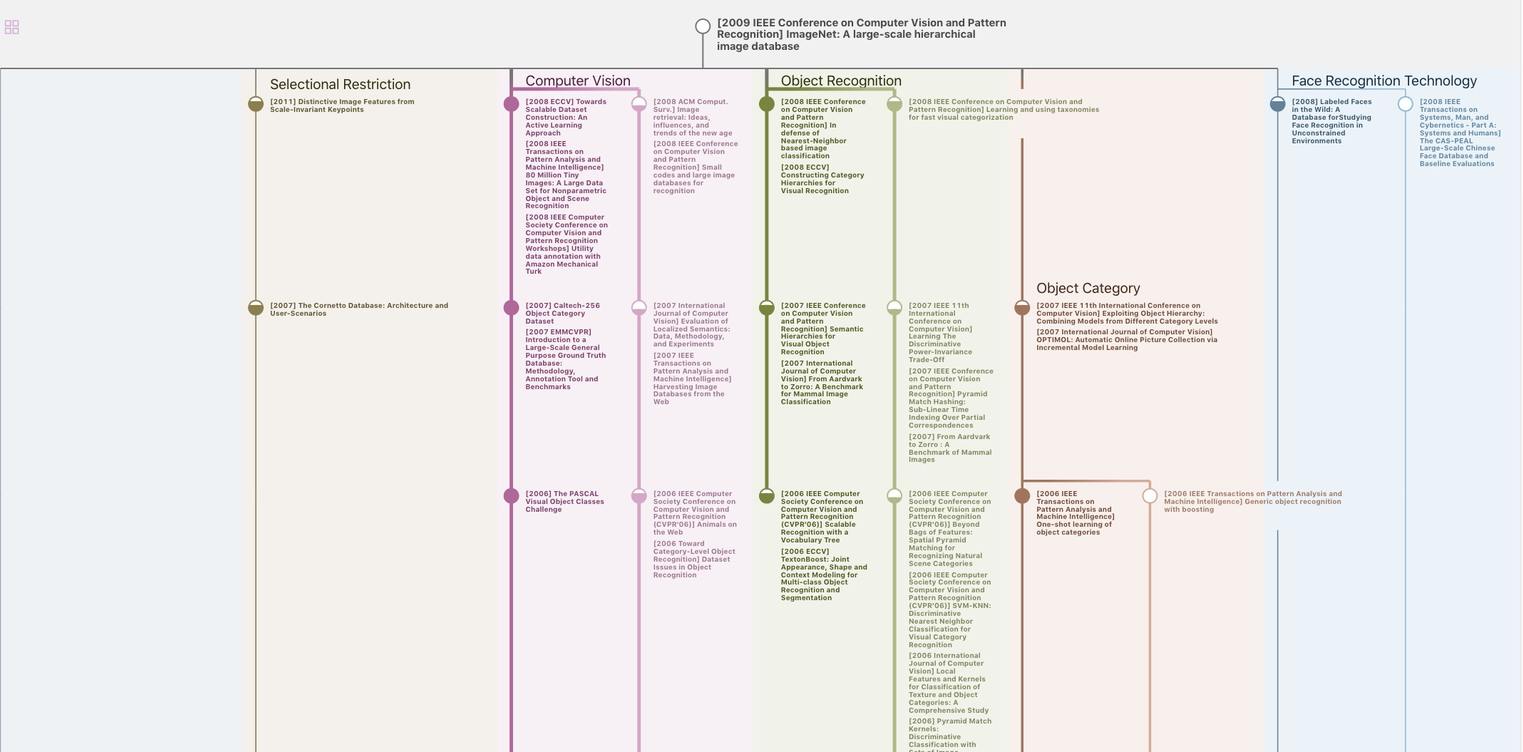
生成溯源树,研究论文发展脉络
Chat Paper
正在生成论文摘要