A Sharp Analysis of Model-based Reinforcement Learning with Self-Play
INTERNATIONAL CONFERENCE ON MACHINE LEARNING, VOL 139(2021)
摘要
Model-based algorithms-algorithms that explore the environment through building and utilizing an estimated model-are widely used in reinforcement learning practice and theoretically shown to achieve optimal sample efficiency for single-agent reinforcement learning in Markov Decision Processes (MDPs). However, for multi-agent reinforcement learning in Markov games, the current best known sample complexity for model-based algorithms is rather suboptimal and compares unfavorably against recent model-free approaches. In this paper, we present a sharp analysis of model-based self-play algorithms for multi-agent Markov games. We design an algorithm Optimistic Nash Value Iteration (Nash-VI) for two-player zero-sum Markov games that is able to output an epsilon-approximate Nash policy in (O) over tilde (H(3)SAB/epsilon(2)) episodes of game playing, where S is the number of states, A, B are the number of actions for the two players respectively, and H is the horizon length. This significantly improves over the best known model-based guarantee of (O) over tilde (H(4)S(2)AB/epsilon(2)), and is the first that matches the information-theoretic lower bound Omega((HS)-S-3 (A + B)/epsilon(2)) except for a min {A, B} factor. In addition, our guarantee compares favorably against the best known model-free algorithm if min {A, B} = o(H-3), and outputs a single Markov policy while existing sample-efficient model-free algorithms output a nested mixture of Markov policies that is in general non-Markov and rather inconvenient to store and execute. We further adapt our analysis to designing a provably efficient task-agnostic algorithm for zero-sum Markov games, and designing the first line of provably sample-efficient algorithms for multiplayer general-sum Markov games.
更多查看译文
关键词
reinforcement learning,model-based,self-play
AI 理解论文
溯源树
样例
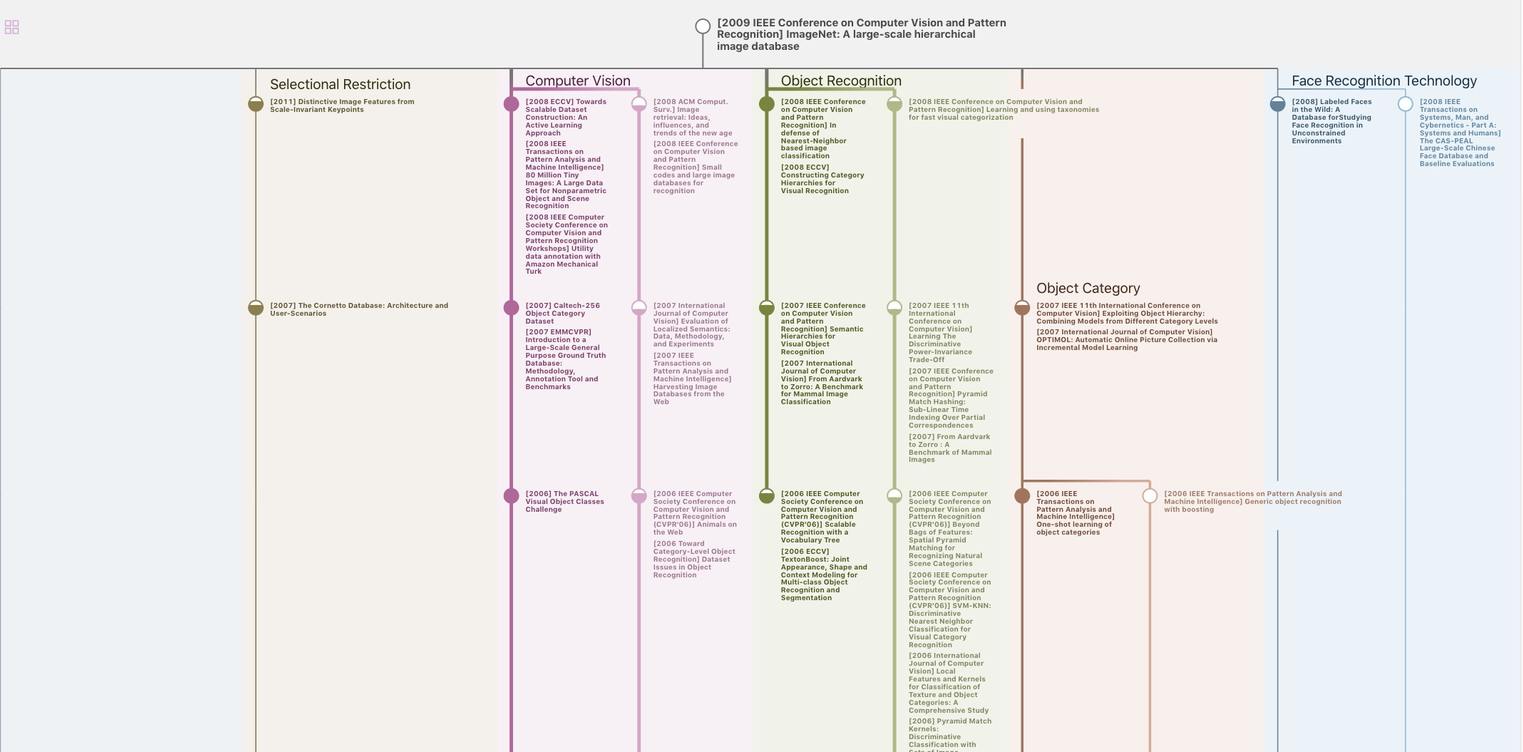
生成溯源树,研究论文发展脉络
Chat Paper
正在生成论文摘要