Neurodynamical Classifiers with Low Model Complexity.
Neural networks(2020)
摘要
The recently proposed Minimal Complexity Machine (MCM) finds a hyperplane classifier by minimizing an upper bound on the Vapnik–Chervonenkis (VC) dimension. The VC dimension measures the capacity or model complexity of a learning machine. Vapnik’s risk formula indicates that models with smaller VC dimension are expected to show improved generalization. On many benchmark datasets, the MCM generalizes better than SVMs and uses far fewer support vectors than the number used by SVMs. In this paper, we describe a neural network that converges to the MCM solution. We employ the MCM neurodynamical system as the final layer of a neural network architecture. Our approach also optimizes the weights of all layers in order to minimize the objective, which is a combination of a bound on the VC dimension and the classification error. We illustrate the use of this model for robust binary and multi-class classification. Numerical experiments on benchmark datasets from the UCI repository show that the proposed approach is scalable and accurate, and learns models with improved accuracies and fewer support vectors.
更多查看译文
关键词
Linear programming,Neural network,VC dimension,Minimal Complexity Machine,Classification
AI 理解论文
溯源树
样例
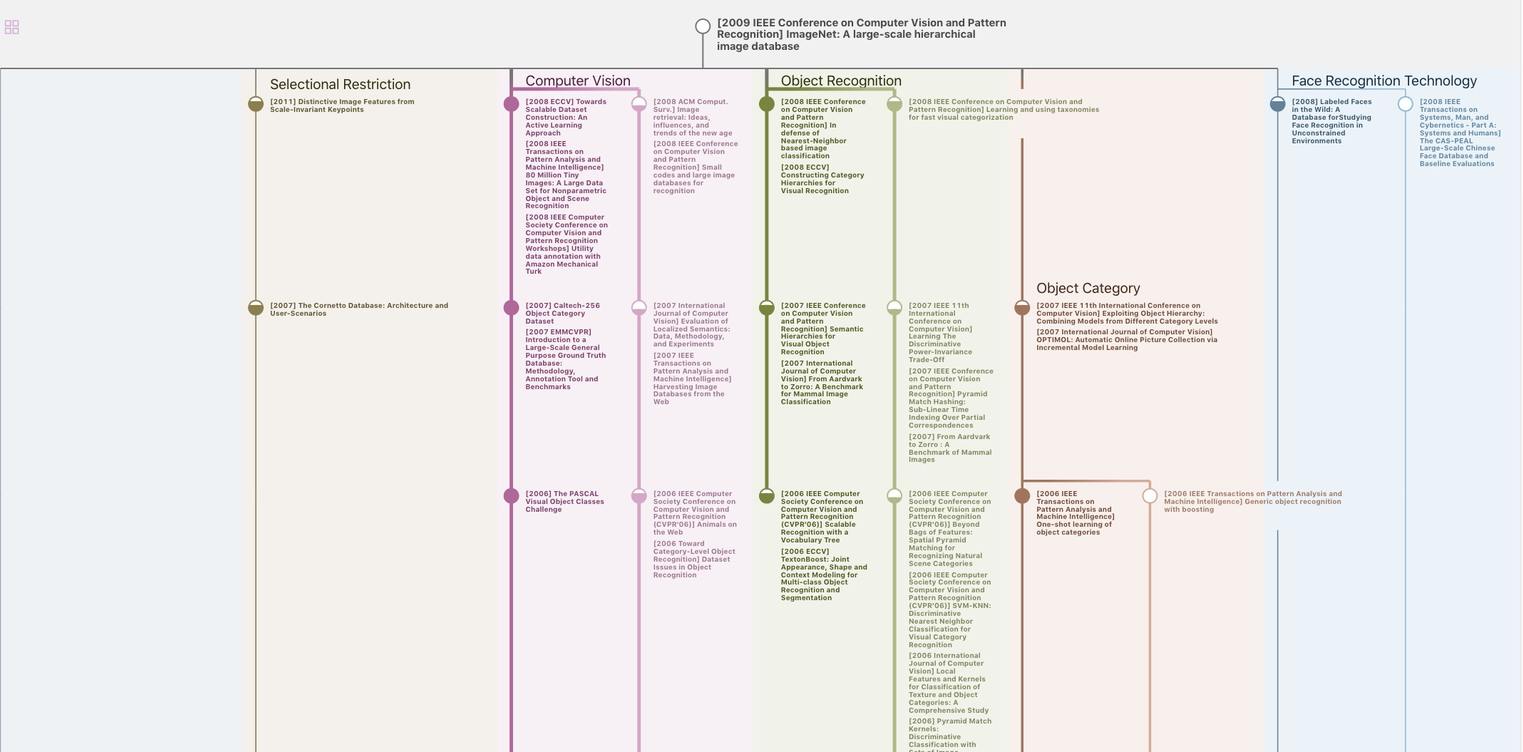
生成溯源树,研究论文发展脉络
Chat Paper
正在生成论文摘要