Learning Time Varying Risk Preferences from Investment Portfolios using Inverse Optimization with Applications on Mutual Funds
arxiv(2020)
摘要
The fundamental principle in Modern Portfolio Theory (MPT) is based on the quantification of the portfolio's risk related to performance. Although MPT has made huge impacts on the investment world and prompted the success and prevalence of passive investing, it still has shortcomings in real-world applications. One of the main challenges is that the level of risk an investor can endure, known as \emph{risk-preference}, is a subjective choice that is tightly related to psychology and behavioral science in decision making. This paper presents a novel approach of measuring risk preference from existing portfolios using inverse optimization on the mean-variance portfolio allocation framework. Our approach allows the learner to continuously estimate real-time risk preferences using concurrent observed portfolios and market price data. We demonstrate our methods on real market data that consists of 20 years of asset pricing and 10 years of mutual fund portfolio holdings. Moreover, the quantified risk preference parameters are validated with two well-known risk measurements currently applied in the field. The proposed methods could lead to practical and fruitful innovations in automated/personalized portfolio management, such as Robo-advising, to augment financial advisors' decision intelligence in a long-term investment horizon.
更多查看译文
关键词
inverse optimization,investment portfolios,risk preferences,mutual funds
AI 理解论文
溯源树
样例
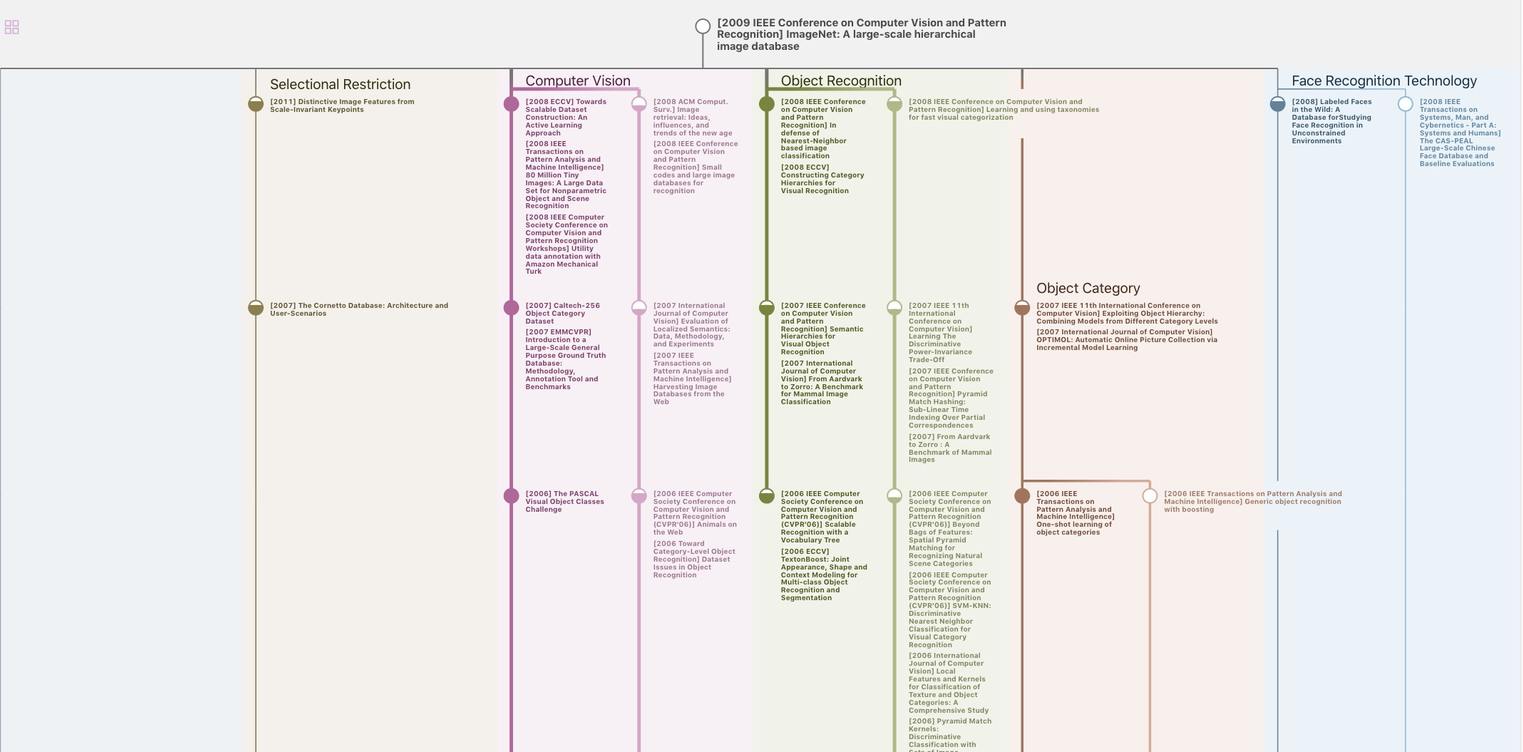
生成溯源树,研究论文发展脉络
Chat Paper
正在生成论文摘要