Direct Signal Separation via Extraction of Local Frequencies With Adaptive Time-Varying Parameters
IEEE TRANSACTIONS ON SIGNAL PROCESSING(2022)
摘要
Real-world phenomena that can be formulated as signals are often affected by a number of factors and appear as multi-component modes. To understand and process such phenomena, "divide-and-conquer" is probably the most common strategy to address the problem. In other words, the captured signal is decomposed into signal components for each individual component to be processed. Unfortunately, for signals that are superimposition of non-stationary amplitude-frequency modulated (AM-FM) components, the "divide-and-conquer" strategy is bound to fail, since there is no way to be sure that the decomposed components take on the AM-FM formulations which are necessary for the extraction of their instantaneous frequencies (IFs) and amplitudes (IAs). In this paper, we propose an adaptive signal separation operation (ASSO) for effective and accurate separation of a single-channel blind-source multi-component signal, via introducing a time-varying parameter that adapts locally to IFs and using linear chirp (linear frequency modulation) signals to approximate components at each time instant. We derive more accurate component recovery formulae based on the linear chirp signal local approximation. In addition, a recovery scheme, together with a ridge detection method, is also proposed to extract the signal components one by one, and the time-varying parameter is updated for each component. The proposed method is suitable for engineering implementation, being capable of separating complicated signals into their components or sub-signals and reconstructing the signal trend directly. Numerical experiments on synthetic and real-world signals are presented to demonstrate our improvement over the previous attempts.
更多查看译文
关键词
Source separation, Chirp, Time-frequency analysis, Market research, Signal processing algorithms, Continuous wavelet transforms, Spectrogram, Time-frequency analysis, Adaptive signal separation operator, Linear chirp local approximation, Instantaneous frequency estimation, Multi-component signal separation
AI 理解论文
溯源树
样例
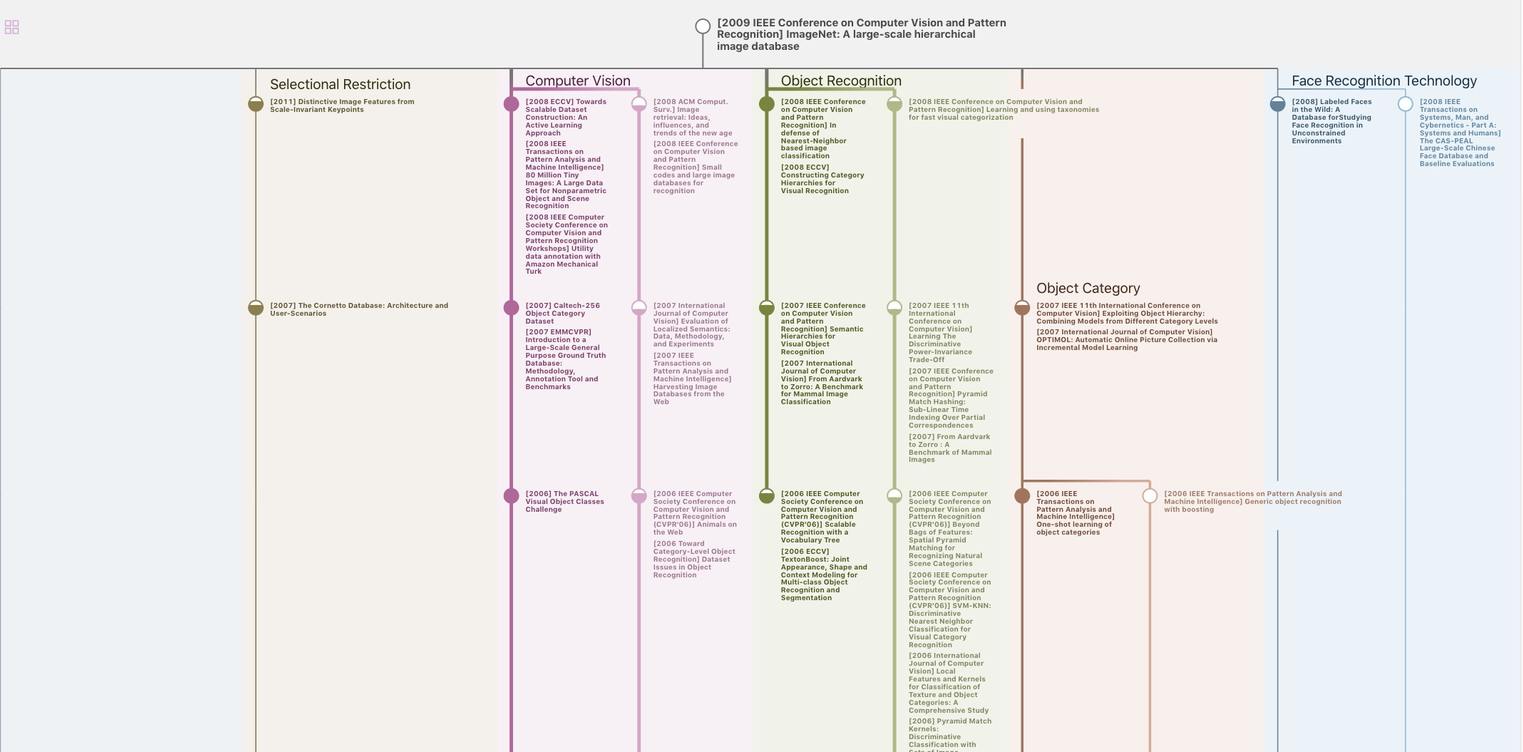
生成溯源树,研究论文发展脉络
Chat Paper
正在生成论文摘要