ResDUnet: Residual Dilated UNet for Left Ventricle Segmentation from Echocardiographic Images
42ND ANNUAL INTERNATIONAL CONFERENCES OF THE IEEE ENGINEERING IN MEDICINE AND BIOLOGY SOCIETY: ENABLING INNOVATIVE TECHNOLOGIES FOR GLOBAL HEALTHCARE EMBC'20(2020)
摘要
Echocardiography is the modality of choice for the assessment of left ventricle function. Left ventricle is responsible for pumping blood rich in oxygen to all body parts. Segmentation of this chamber from echocardiographic images is a challenging task, due to the ambiguous boundary and inhomogeneous intensity distribution. In this paper we propose a novel deep learning model named ResDUnet. The model is based on U-net incorporated with dilated convolution, where residual blocks are employed instead of the basic U-net units to ease the training process. Each block is enriched with squeeze and excitation unit for channel-wise attention and adaptive feature re-calibration. To tackle the problem of left ventricle shape and size variability, we chose to enrich the process of feature concatenation in U-net by integrating feature maps generated by cascaded dilation. Cascaded dilation broadens the receptive field size in comparison with traditional convolution, which allows the generation of multi-scale information which in turn results in a more robust segmentation. Performance measures were evaluated on a publicly available dataset of 500 patients with large variability in terms of quality and patients pathology. The proposed model shows a dice similarity increase of 8.4% when compared to deeplabv3 and 1.2% when compared to the basic U-net architecture. Experimental results demonstrate the potential use in clinical domain.
更多查看译文
关键词
Echocardiography,Heart Ventricles,Humans,Specimen Handling
AI 理解论文
溯源树
样例
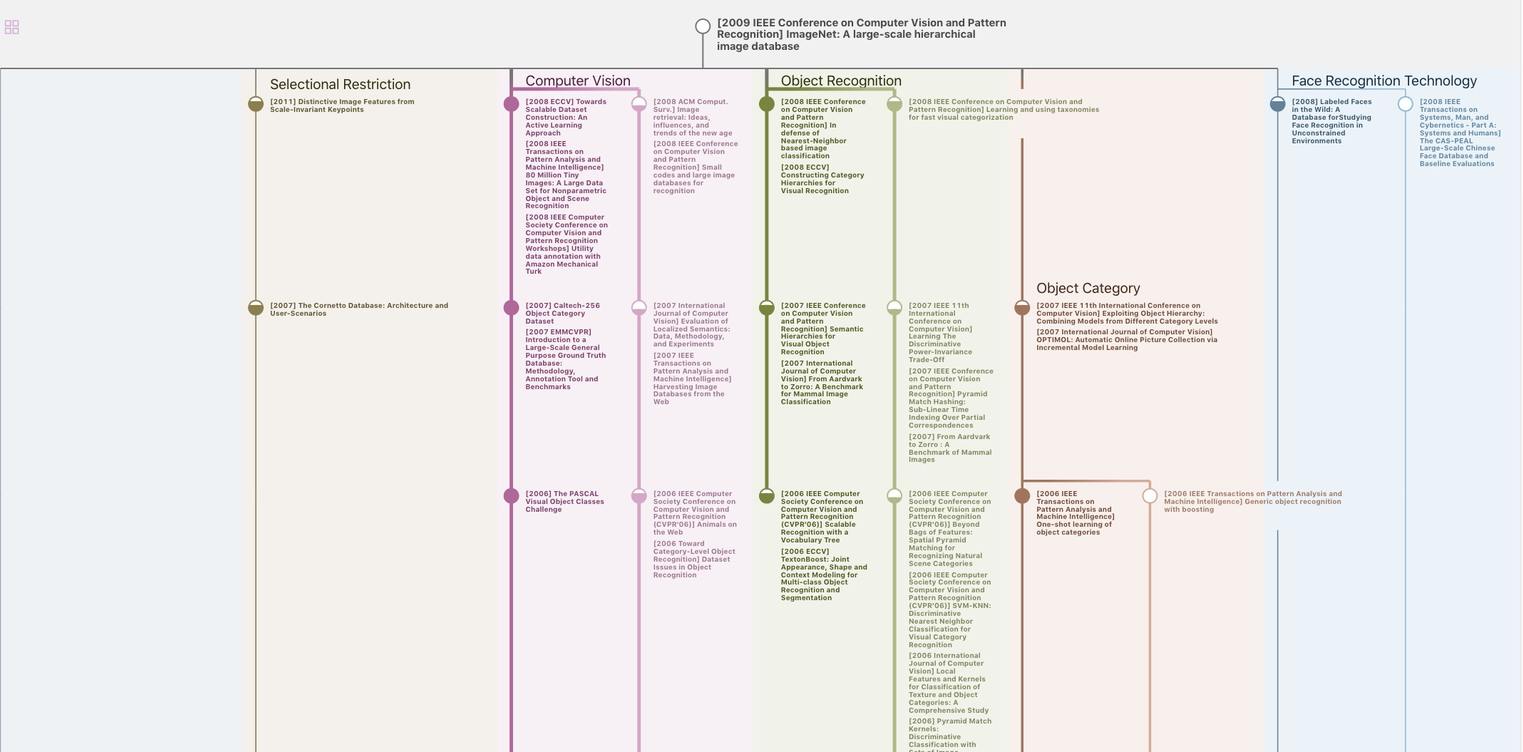
生成溯源树,研究论文发展脉络
Chat Paper
正在生成论文摘要