Extracting Membrane Borders in IVUS Images Using a Multi-Scale Feature Aggregated U-Net
42ND ANNUAL INTERNATIONAL CONFERENCES OF THE IEEE ENGINEERING IN MEDICINE AND BIOLOGY SOCIETY: ENABLING INNOVATIVE TECHNOLOGIES FOR GLOBAL HEALTHCARE EMBC'20(2020)
摘要
Automatic extraction of the lumen-intima border (LIB) and the media-adventitia border (MAB) in intravascular ultrasound (IVUS) images is of high clinical interest. Despite the superior performance achieved by deep neural networks (DNNs) on various medical image segmentation tasks, there are few applications to IVUS images. The complicated pathological presentation and the lack of enough annotation in IVUS datasets make the learning process challenging. Several existing networks designed for IVUS segmentation train two groups of weights to detect the MAB and LIB separately. In this paper, we propose a multi- scale feature aggregated U-Net (MFAU-Net) to extract two membrane borders simultaneously. The MFAU-Net integrates multi-scale inputs, the deep supervision, and a bi-directional convolutional long short-term memory (BConvLSTM) unit. It is designed to sufficiently learn features from complicated IVUS images through a small number of training samples. Trained and tested on the publicly available IVUS datasets, the MFAU-Net achieves both 0.90 Jaccard measure (JM) for the MAB and LIB detection on 20 MHz dataset. The corresponding metrics on 40 MHz dataset are 0.85 and 0.84 JM respectively. Comparative evaluations with state-of-the-art published results demonstrate the competitiveness of the proposed MFAU-Net.
更多查看译文
关键词
Intravascular ultrasound,border detection,deep neural networks,ConvLSTM
AI 理解论文
溯源树
样例
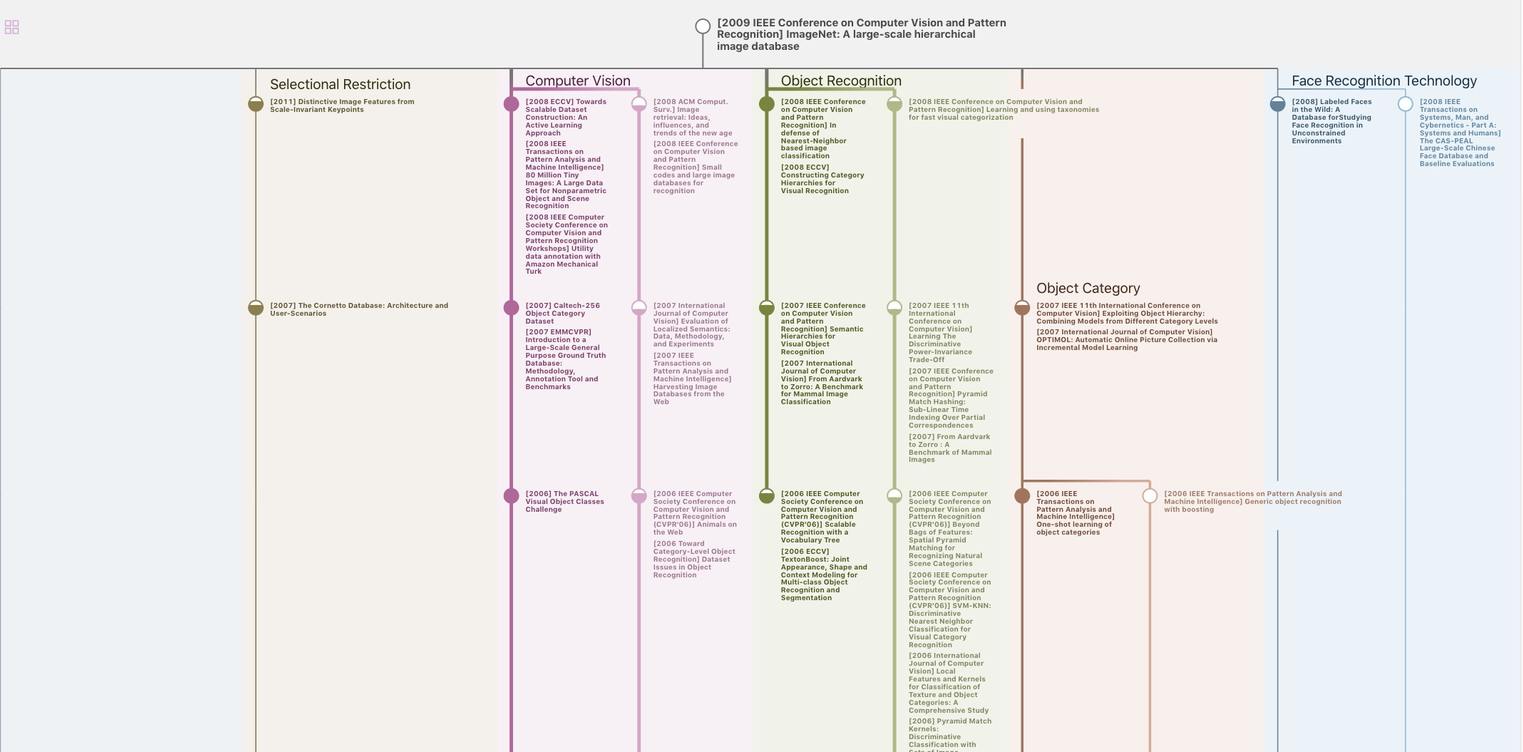
生成溯源树,研究论文发展脉络
Chat Paper
正在生成论文摘要