Decoding Movement Direction From Cortical Microelectrode Recordings Using An Lstm-Based Neural Network
42ND ANNUAL INTERNATIONAL CONFERENCES OF THE IEEE ENGINEERING IN MEDICINE AND BIOLOGY SOCIETY: ENABLING INNOVATIVE TECHNOLOGIES FOR GLOBAL HEALTHCARE EMBC'20(2020)
摘要
Brain-machine interfaces (BMIs) allow individuals to communicate with computers using neural signals, and Kalman Filter (KF) are prevailingly used to decode movement directions from these neural signals. In this paper, we implemented a multi-layer long short-term memory (LSTM)-based artificial neural network (ANN) for decoding BMI neural signals. We collected motor cortical neural signals from a non-human primate (NHP), implanted with microelectrode array (MEA) while performing a directional joystick task. Next, we compared the LSTM model in decoding the joystick trajectories from the neural signals against the prevailing KF model. The results showed that the LSTM model yielded significantly improved decoding accuracy measured by mean correlation coefficient (0.84, p < 10(-7)) than the KF model (0.72). In addition, using a principal component analysis (PCA)-based dimensionality reduction technique yielded slightly deteriorated accuracies for both the LSTM (0.80) and KF (0.70) models, but greatly reduced the computational complexity. The results showed that the LSTM decoding model holds promise to improve decoding in BMIs for paralyzed humans.
更多查看译文
关键词
brain-computer interface, BCI, brain-machine interface, BMI, asynchronous decoding, recurrent neural network, LSTM, principal component analysis, PCA
AI 理解论文
溯源树
样例
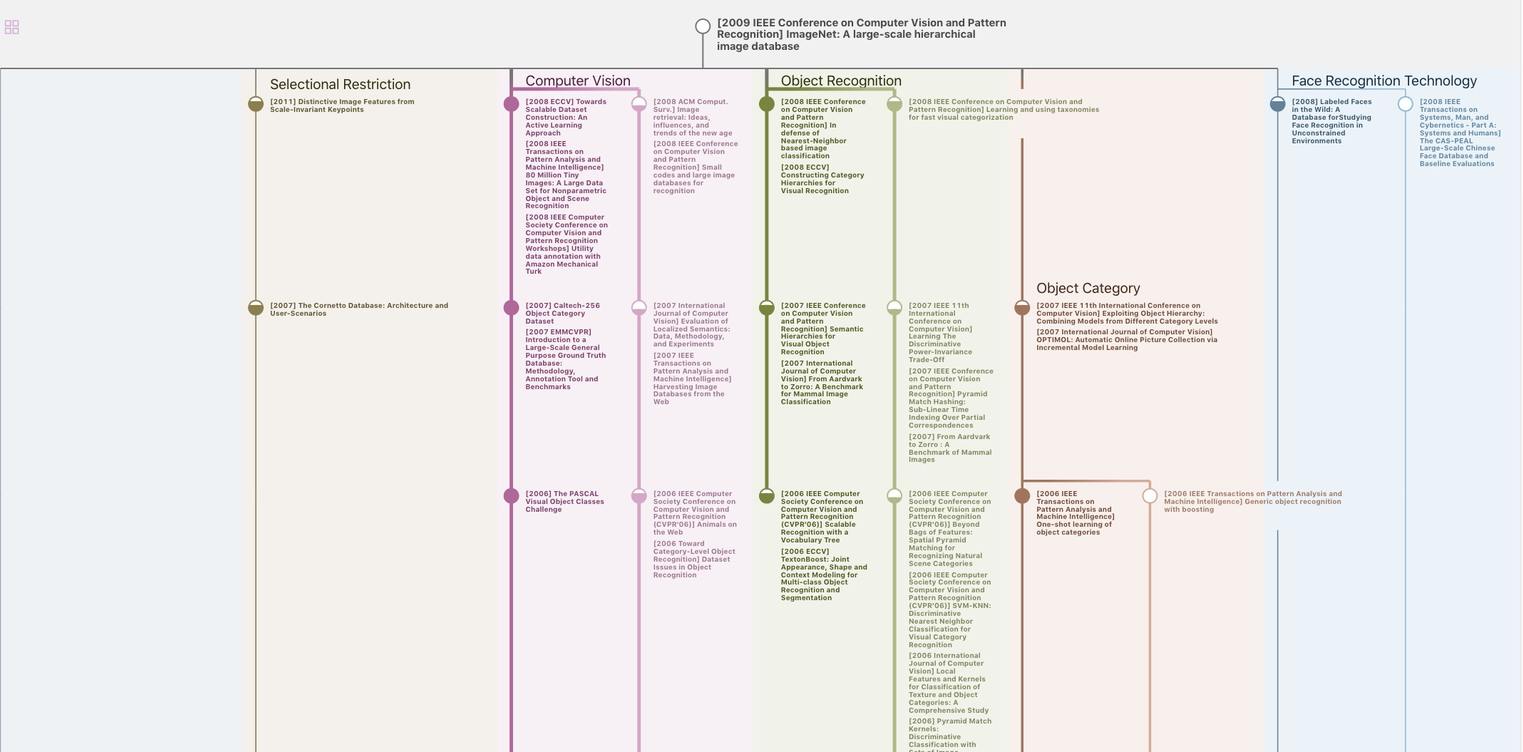
生成溯源树,研究论文发展脉络
Chat Paper
正在生成论文摘要