Adapting To Noise In Speech Obfuscation By Audio Profiling Using Generative Models For Passive Health Monitoring
42ND ANNUAL INTERNATIONAL CONFERENCES OF THE IEEE ENGINEERING IN MEDICINE AND BIOLOGY SOCIETY: ENABLING INNOVATIVE TECHNOLOGIES FOR GLOBAL HEALTHCARE EMBC'20(2020)
摘要
Passive health monitoring has been introduced as a solution for continuous diagnosis and tracking of subjects' condition with minimal effort. This is partially achieved by the technology of passive audio recording although it poses major audio privacy issues for subjects. Existing methods are limited to controlled recording environments and their prediction is significantly influenced by background noises. Meanwhile, they are too compute-intensive to be continuously running on smart phones. In this paper, we implement an efficient and robust audio privacy preserving method that profiles the background audio to focus only on audio activities detected during recording for performance improvement, and to adapt to the noise for more accurate speech segmentation. We analyze the performance of our method using audio data collected by a smart watch in lab noisy settings. Our obfuscation results show a low false positive rate of 20% with a 92% true positive rate by adapting to the recording noise level. We also reduced model memory footprint and execution time of the method on a smart phone by 75% and 62% to enable continuous speech obfuscation.
更多查看译文
关键词
Communications Media,Noise,Smartphone,Speech,Speech Perception
AI 理解论文
溯源树
样例
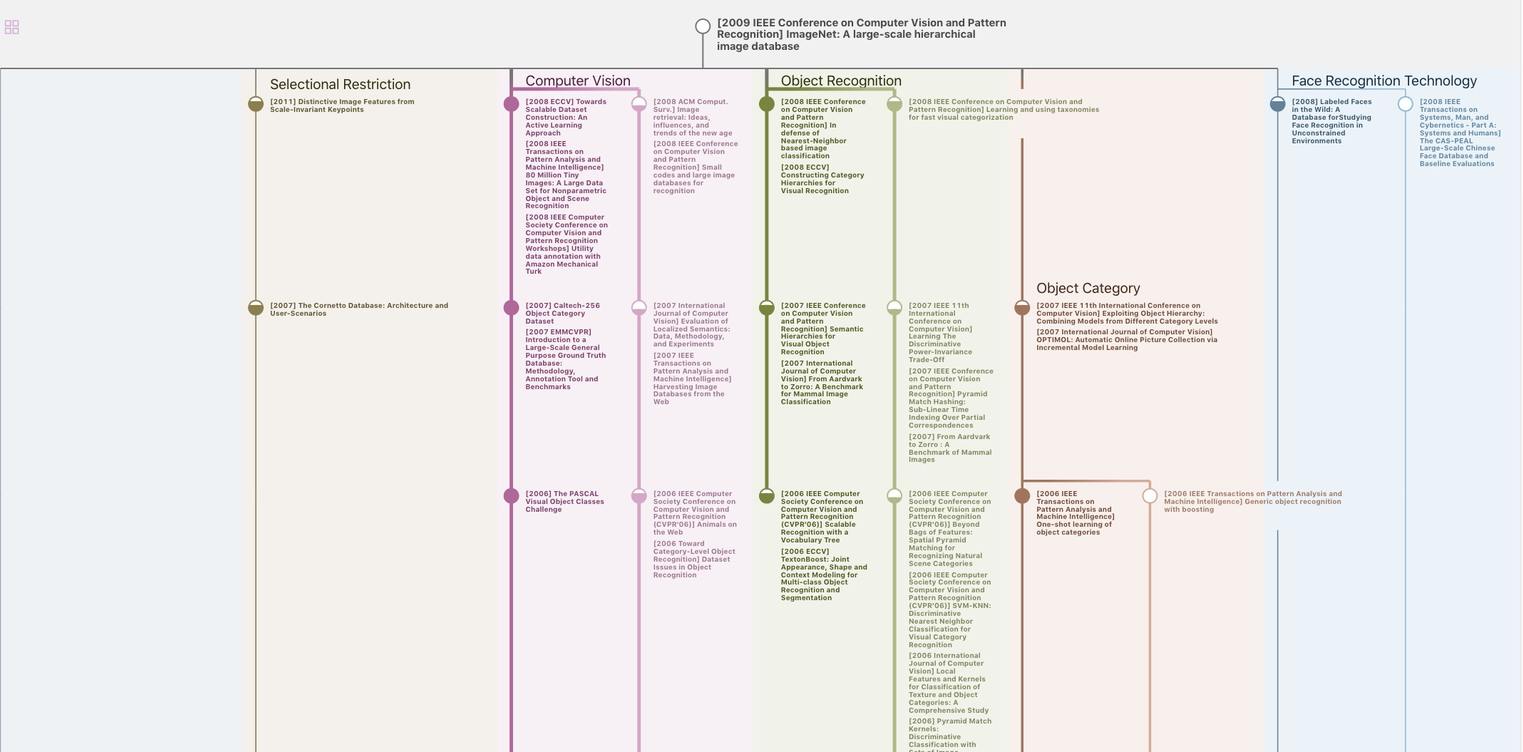
生成溯源树,研究论文发展脉络
Chat Paper
正在生成论文摘要