Fault Diagnosis of Bearings with Variable Rotational Speeds Using Convolutional Neural Networks
ADVANCES IN COMPUTER COMMUNICATION AND COMPUTATIONAL SCIENCES, VOL 1(2019)
摘要
Feature extraction is of great significance in the traditional bearing fault diagnosis methods which detect incipient defects by identifying peaks at characteristic defect frequencies in the envelope power spectrum or by developing discriminative models for features extracted from the signals through time and frequency domain analysis. This issue not only requires expert knowledge to design discriminative features but also requires appropriate feature selection algorithms to eliminate irrelevant and redundant features that degrade the performance of the methods. In this paper, we present a convolutional neural networks (CNNs) based method to automatically derive optimal features which not require any feature extraction or feature selection. The proposed method identifies bearing defects efficiently by processing feature extraction and classification into a single learning machine and automatically extracts optimal features from these energy distribution maps to diagnose various single and compound bearing defects and yields better diagnostic performance compared to state-of-the-art AE-based methods.
更多查看译文
关键词
Convolutional neural networks,Stochastic diagonal,Levenberg-Marquardt algorithm,Bearing fault diagnosis
AI 理解论文
溯源树
样例
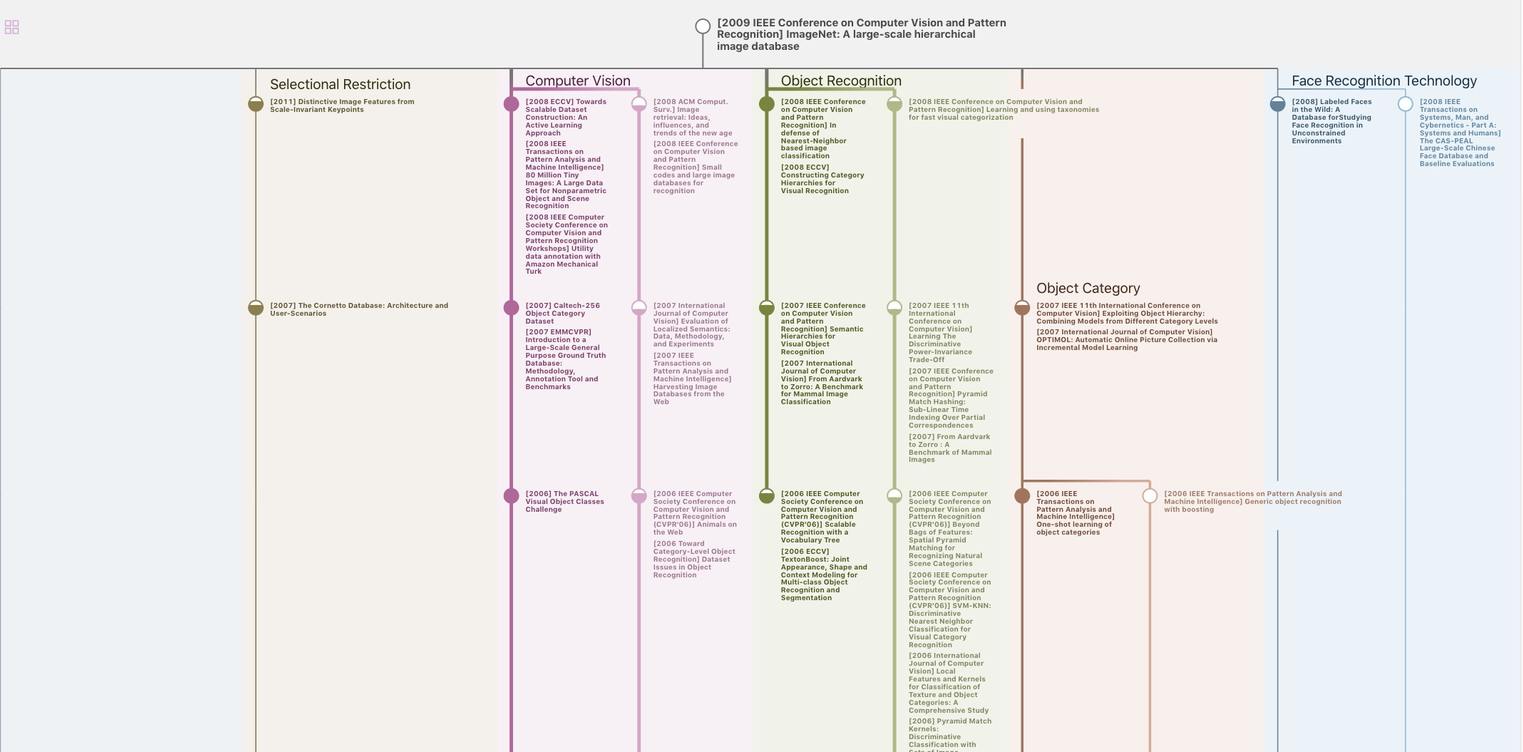
生成溯源树,研究论文发展脉络
Chat Paper
正在生成论文摘要