Online Linear Optimization with Many Hints
NIPS 2020(2020)
摘要
We study an online linear optimization (OLO) problem in which the learner is provided access to $K$ "hint" vectors in each round prior to making a decision. In this setting, we devise an algorithm that obtains logarithmic regret whenever there exists a convex combination of the $K$ hints that has positive correlation with the cost vectors. This significantly extends prior work that considered only the case $K=1$. To accomplish this, we develop a way to combine many arbitrary OLO algorithms to obtain regret only a logarithmically worse factor than the minimum regret of the original algorithms in hindsight; this result is of independent interest.
更多查看译文
关键词
online linear optimization
AI 理解论文
溯源树
样例
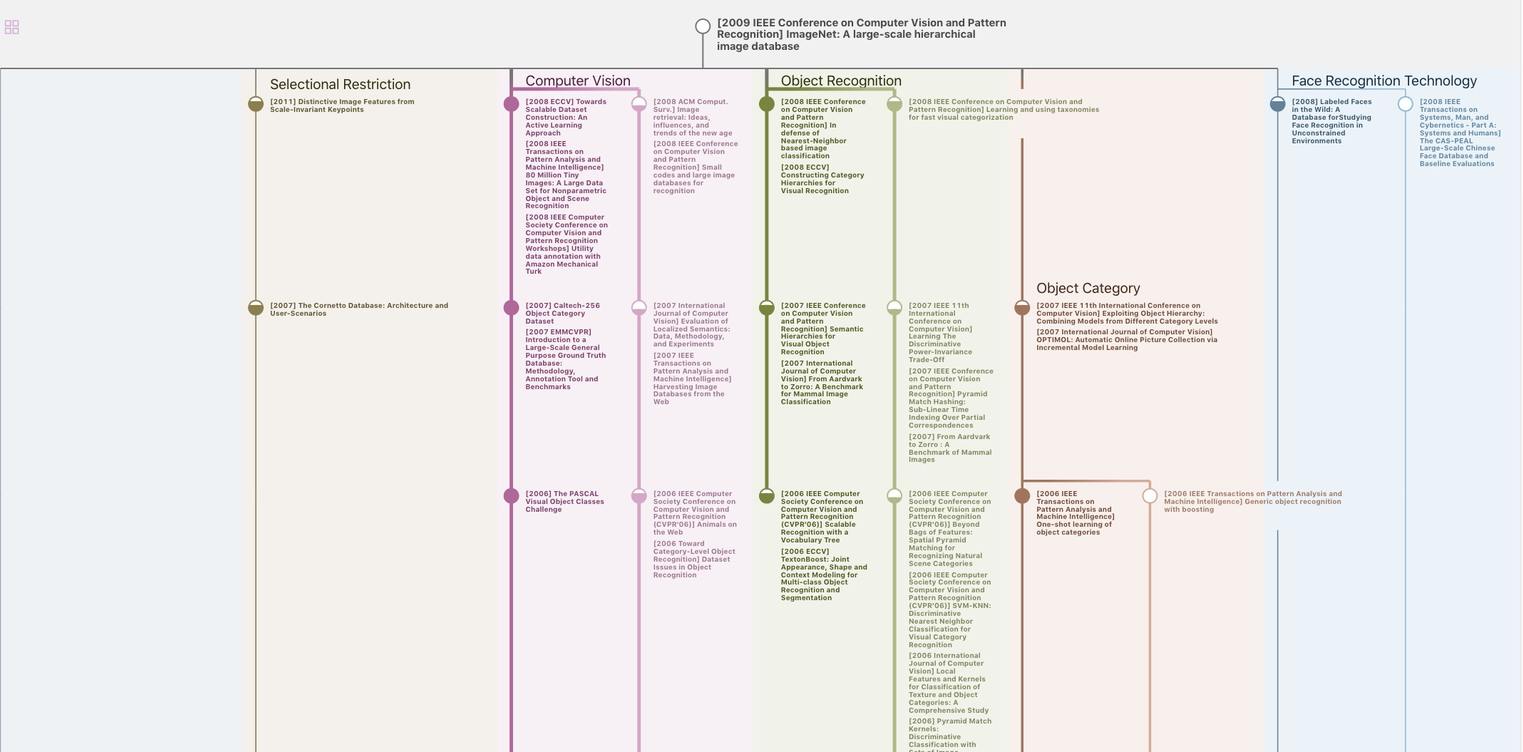
生成溯源树,研究论文发展脉络
Chat Paper
正在生成论文摘要