Insider Collusion Attack on Distributed Machine Learning System and its Solutions - A Case of SVM
ASIA CCS '20: The 15th ACM Asia Conference on Computer and Communications Security Taipei Taiwan October, 2020(2020)
摘要
Among several known data breaching problems, insider collusion attacks have been one of the fastest-growing threats. However, there are very few insider-related researches involving the distribution of kernel-based data mining, such as distributed Support Vector Machine (SVM). Prior works often fall short by addressing a multifactorial model that is more limited in scope and implementation than addressing "insiders within an organization" colluding with outsiders. In this talk, I will introduce a novel insider collusion attack, in which a faulty system allows collusion to go unnoticed when an insider shares data with an outsider, who can then recover the original data from message transmissions (intermediary kernel values) among organizations. This attack requires only accessibility to a few data entries within the organizations rather than requiring the encrypted administrative privileges typically found in the distribution of data mining scenarios. I will also provide the analysis about the minimum amount of insider data necessary to launch the insider attack. Finally, I will discuss several proposed privacy-preserving schemes, like Differential Privacy, Homomorphic Proxy Re-Encryption, and etc., to counter the described attack.
更多查看译文
AI 理解论文
溯源树
样例
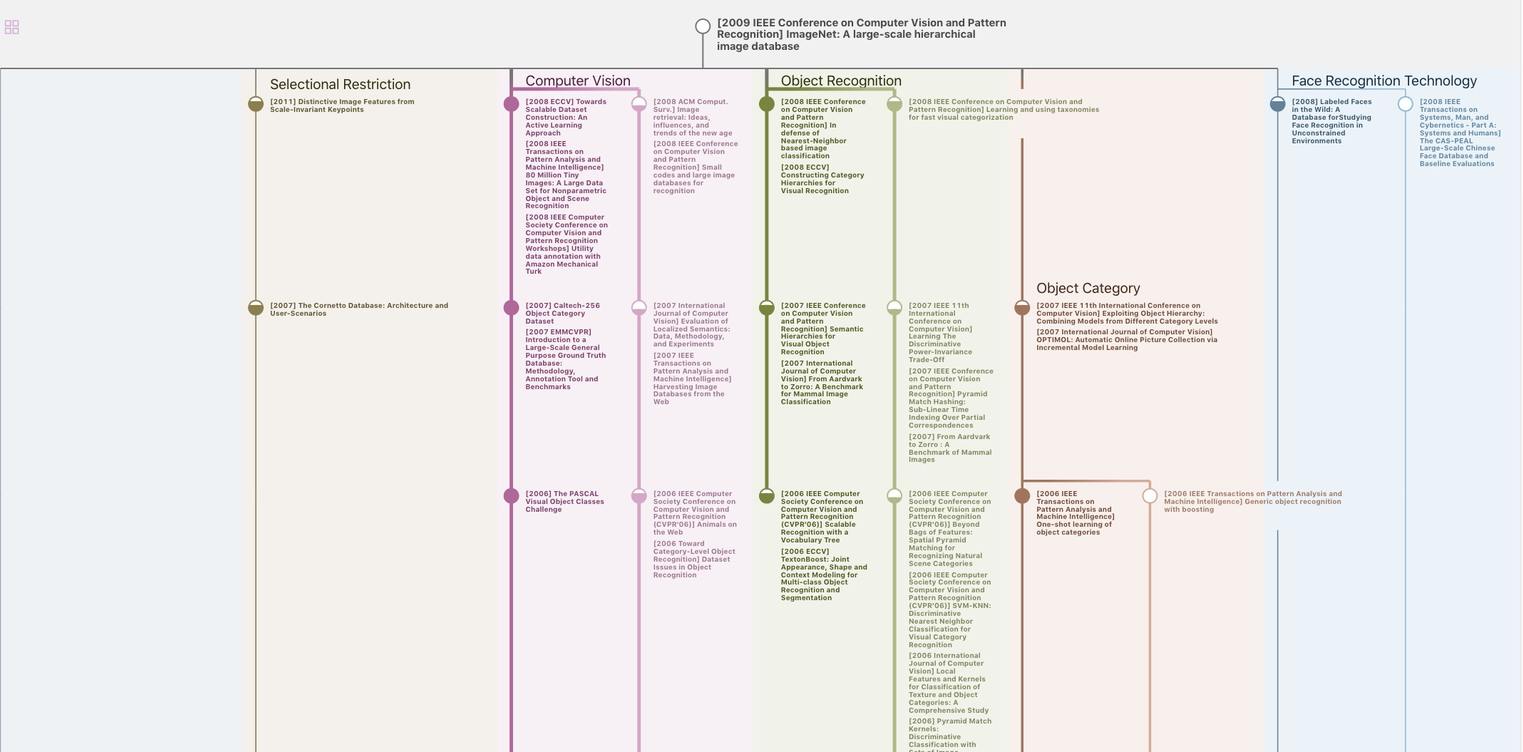
生成溯源树,研究论文发展脉络
Chat Paper
正在生成论文摘要