Multi-layer Coordination for High-Performance Energy-Efficient Federated Learning
2020 IEEE/ACM 28th International Symposium on Quality of Service (IWQoS)(2020)
摘要
Federated Learning is designed for multiple mobile devices to collaboratively train an artificial intelligence model while preserving data privacy. Instead of collecting the raw training data from mobile devices to the cloud, Federated Learning coordinates a group of devices to train a shared model in a distributed manner with the training data located on the devices. However, in order to effectively deploy Federated Learning on resource-constrained mobile devices, several critical issues including convergence rate, scalability and energy efficiency should be well addressed. In this paper, we propose MCFL, a multi-layer online coordination framework for high-performance energy efficient federated learning. MCFL consists of two layers: a macro-layer on the central server and a micro-layer on each participating device. In each training round, the macro coordinator performs two tasks, namely, selecting the right devices to participate, and estimating a time limit, such that the overall training time is significantly reduced while still guaranteeing the model accuracy. Unlike existing systems, MCFL removes the restriction that participating devices must be connected to power sources, thus allowing more timely and ubiquitous training. This clearly requires on-device training to be highly energy-efficient. To this end, the micro coordinator determines optimal schedules for hardware resources in order to meet the time limit set by the macro coordinator with the least amount of energy consumption. Tested on real devices as well as simulation testbed, MCFL has shown to be able to effectively balance the convergence rate, model accuracy and energy efficiency. Compared with existing systems, MCFL can achieve a speedup up to 8.66× and reduce energy consumption by up to 76.5% during the training process.
更多查看译文
AI 理解论文
溯源树
样例
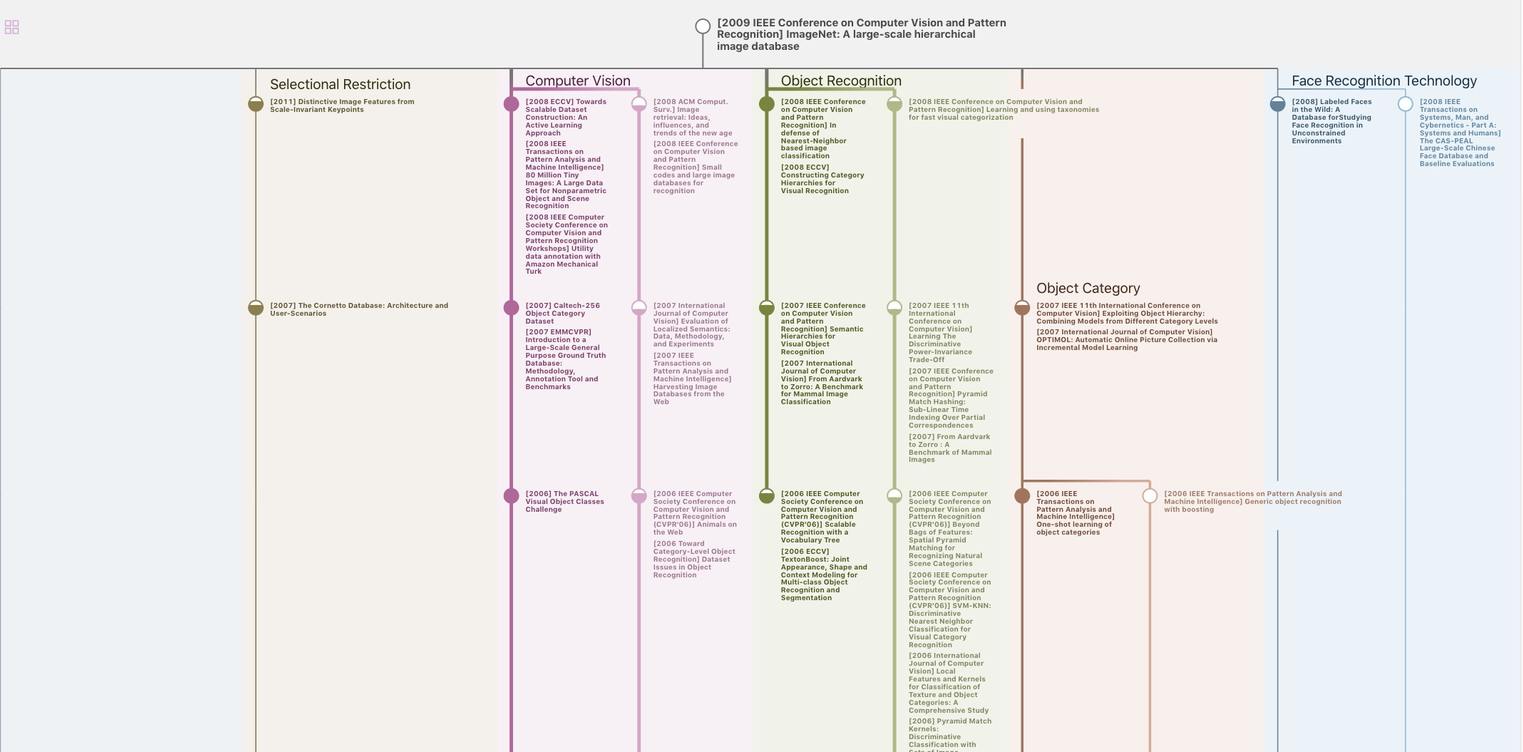
生成溯源树,研究论文发展脉络
Chat Paper
正在生成论文摘要