Diversity can be Transferred: Output Diversification for White- and Black-box Attacks
NIPS 2020(2020)
摘要
Adversarial attacks often involve random perturbations of the inputs drawn from uniform or Gaussian distributions, e.g. to initialize optimization-based white-box attacks or generate update directions in black-box attacks. These simple perturbations, however, could be sub-optimal as they are agnostic to the model being attacked. To improve the efficiency of these attacks, we propose Output Diversified Sampling (ODS), a novel sampling strategy that attempts to maximize diversity in the target model's outputs among the generated samples. While ODS is a gradient-based strategy, the diversity offered by ODS is transferable and can be helpful for both white-box and black-box attacks via surrogate models. Empirically, we demonstrate that ODS significantly improves the performance of existing white-box and black-box attacks. In particular, ODS reduces the number of queries needed for state-of-the-art black-box attacks on ImageNet by a factor of two.
更多查看译文
关键词
diversity,output diversification,black-box black-box
AI 理解论文
溯源树
样例
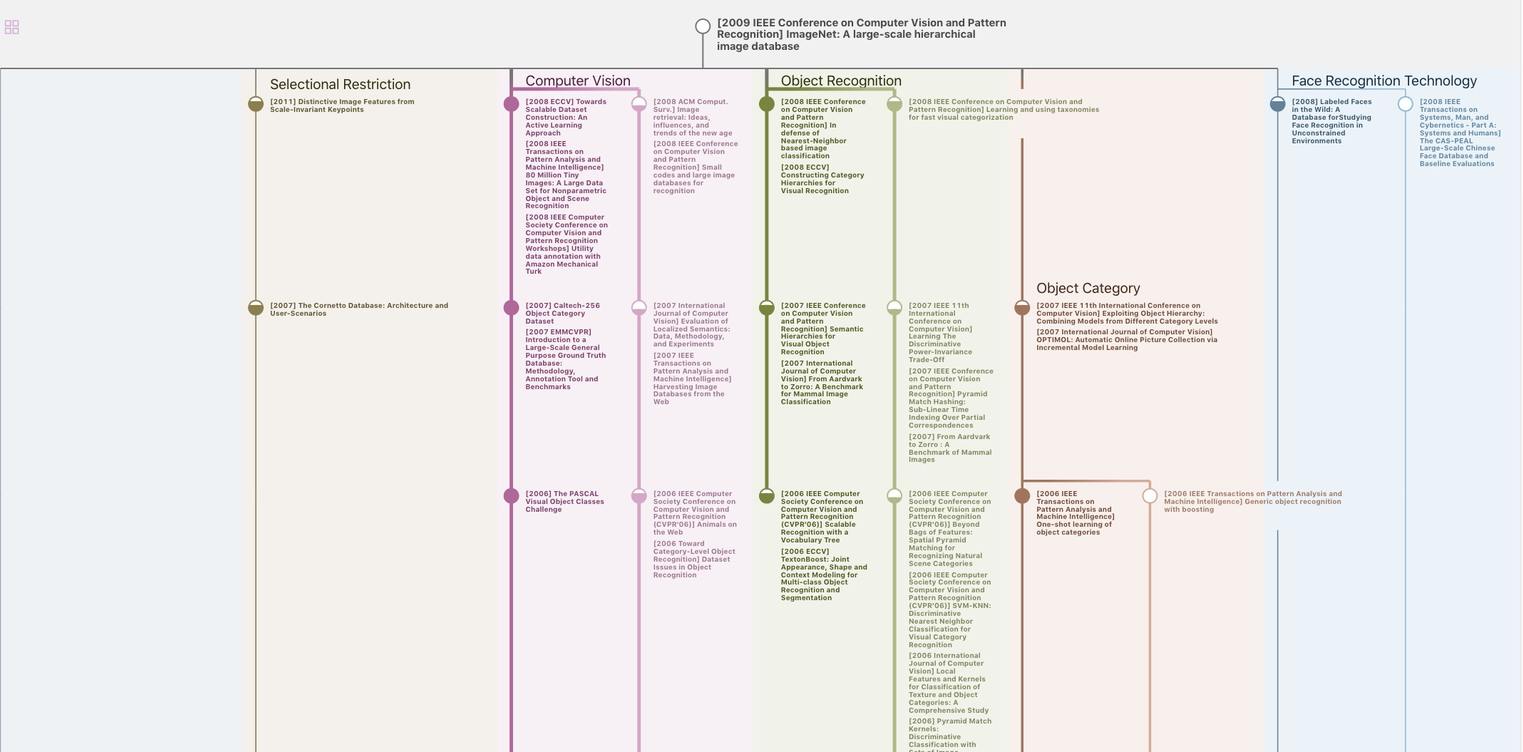
生成溯源树,研究论文发展脉络
Chat Paper
正在生成论文摘要