Evidential Sparsification of Multimodal Latent Spaces in Conditional Variational Autoencoders
NIPS 2020(2020)
摘要
Discrete latent spaces in variational autoencoders have been shown to effectively capture the data distribution for many real-world problems such as natural language understanding, human intent prediction, and visual scene representation. However, discrete latent spaces need to be sufficiently large to capture the complexities of real-world data, rendering downstream tasks computationally challenging. For instance, performing motion planning in a high-dimensional latent representation of the environment could be intractable. We consider the problem of sparsifying the discrete latent space of a trained conditional variational autoencoder, while preserving its learned multimodality. As a post hoc latent space reduction technique, we use evidential theory to identify the latent classes that receive direct evidence from a particular input condition and filter out those that do not. Experiments on diverse tasks, such as image generation and human behavior prediction, demonstrate the effectiveness of our proposed technique at reducing the discrete latent sample space size of a model while maintaining its learned multimodality.
更多查看译文
关键词
conditional variational autoencoders,multimodal latent spaces,evidential sparsification
AI 理解论文
溯源树
样例
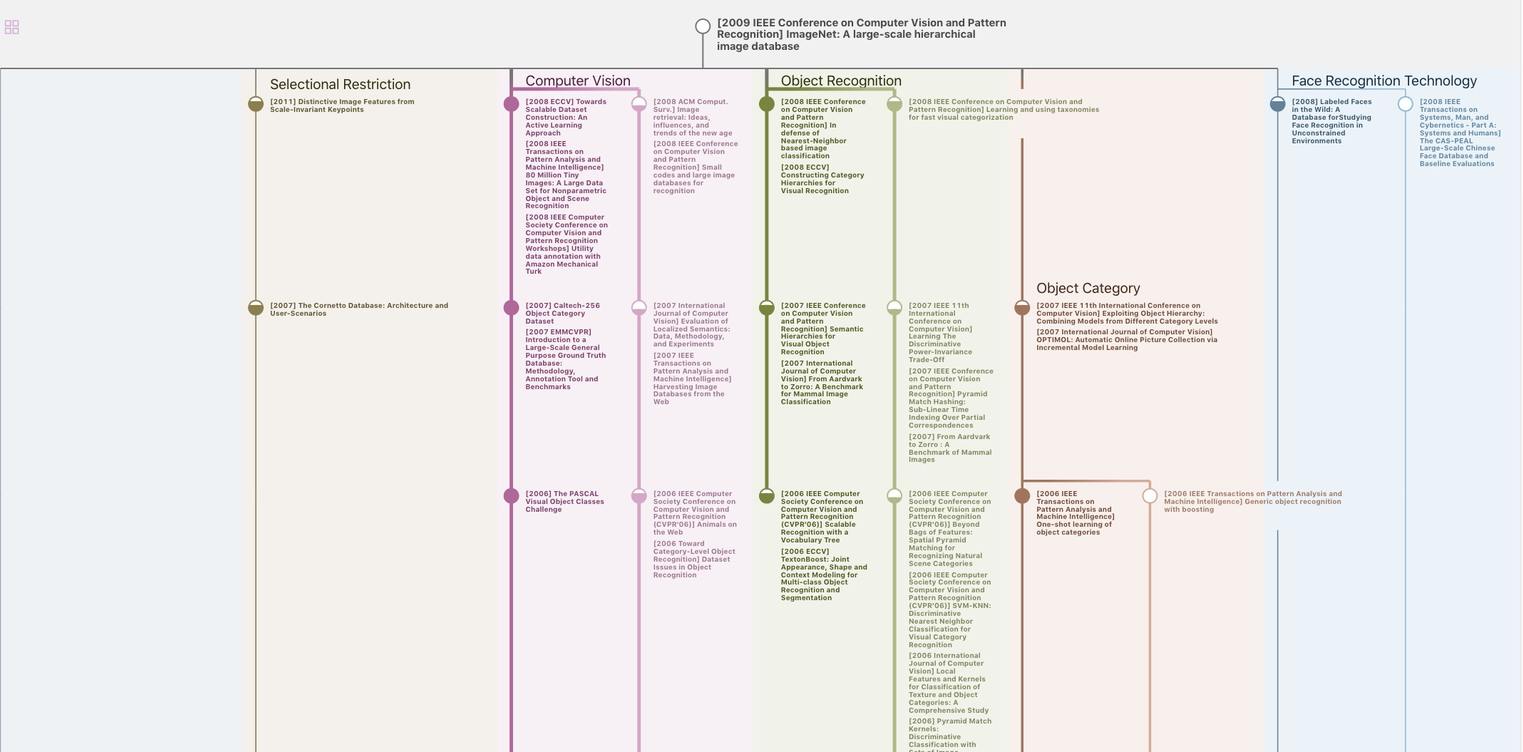
生成溯源树,研究论文发展脉络
Chat Paper
正在生成论文摘要