Better Set Representations For Relational Reasoning
NIPS 2020(2020)
摘要
Incorporating relational reasoning into neural networks has greatly expanded their capabilities and scope. One defining trait of relational reasoning is that it operates on a set of entities, as opposed to standard vector representations. Existing end-to-end approaches for relational reasoning typically extract entities from inputs by directly interpreting the latent feature representations as a set. We show that these approaches do not respect set permutational invariance and thus have fundamental representational limitations. To resolve this limitation, we propose a simple and general network module called Set Refiner Network (SRN). We first use synthetic image experiments to demonstrate how our approach effectively decomposes objects without explicit supervision. Then, we insert our module into existing relational reasoning models and show that respecting set invariance leads to substantial gains in prediction performance and robustness on several relational reasoning tasks. Code can be found at github.com/CUAI/BetterSetRepresentations.
更多查看译文
关键词
relational reasoning,representations
AI 理解论文
溯源树
样例
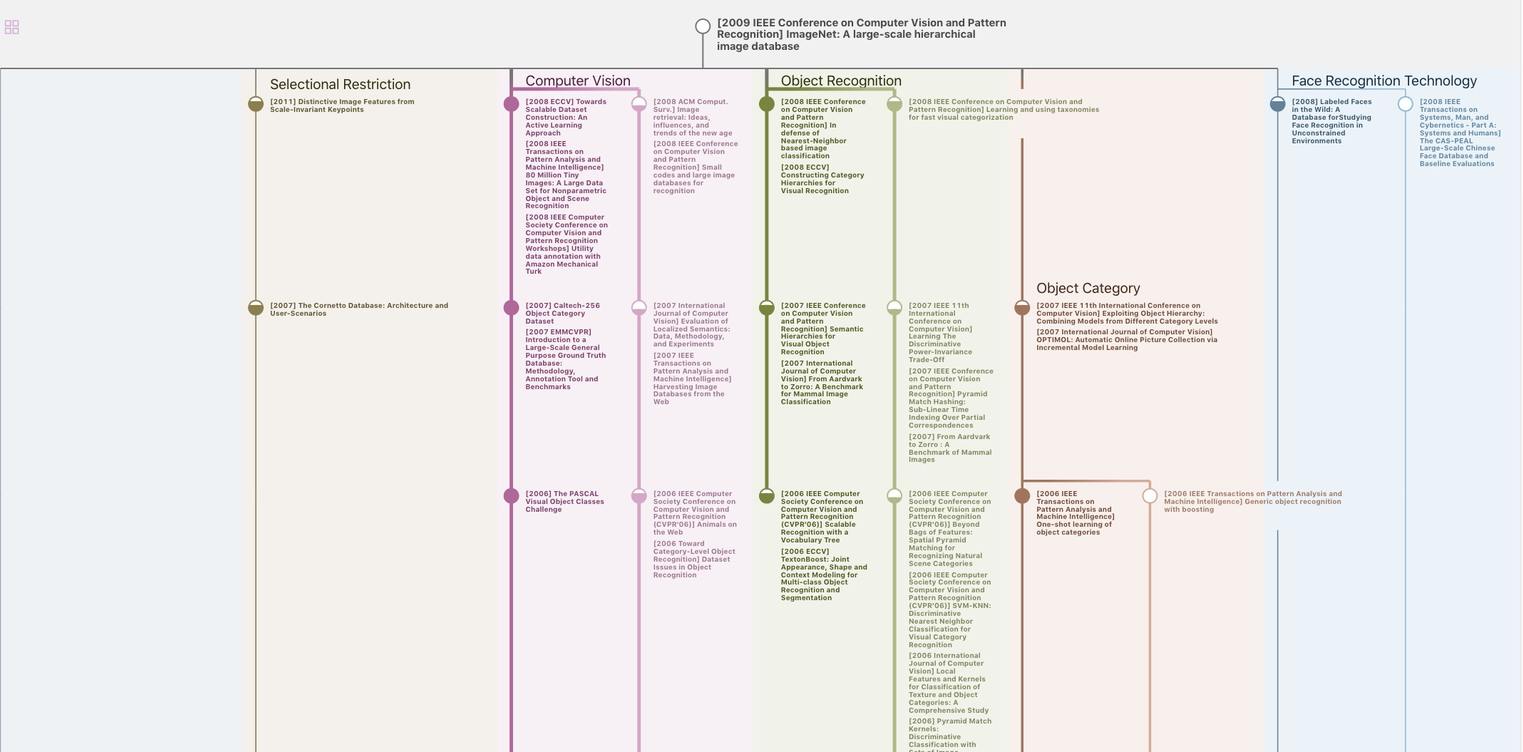
生成溯源树,研究论文发展脉络
Chat Paper
正在生成论文摘要