Deep Reinforcement Learning with Stacked Hierarchical Attention for Text-based Games
NIPS 2020(2020)
摘要
We study reinforcement learning (RL) for text-based games, which are interactive simulations in the context of natural language. While different methods have been developed to represent the environment information and language actions, existing RL agents are not empowered with any reasoning capabilities to deal with textual games. In this work, we aim to conduct explicit reasoning with knowledge graphs for decision making, so that the actions of an agent are generated and supported by an interpretable inference procedure. We propose a stacked hierarchical attention mechanism to construct an explicit representation of the reasoning process by exploiting the structure of the knowledge graph. We extensively evaluate our method on a number of man-made benchmark games, and the experimental results demonstrate that our method performs better than existing text-based agents.
更多查看译文
关键词
stacked hierarchical attention,games,learning,text-based
AI 理解论文
溯源树
样例
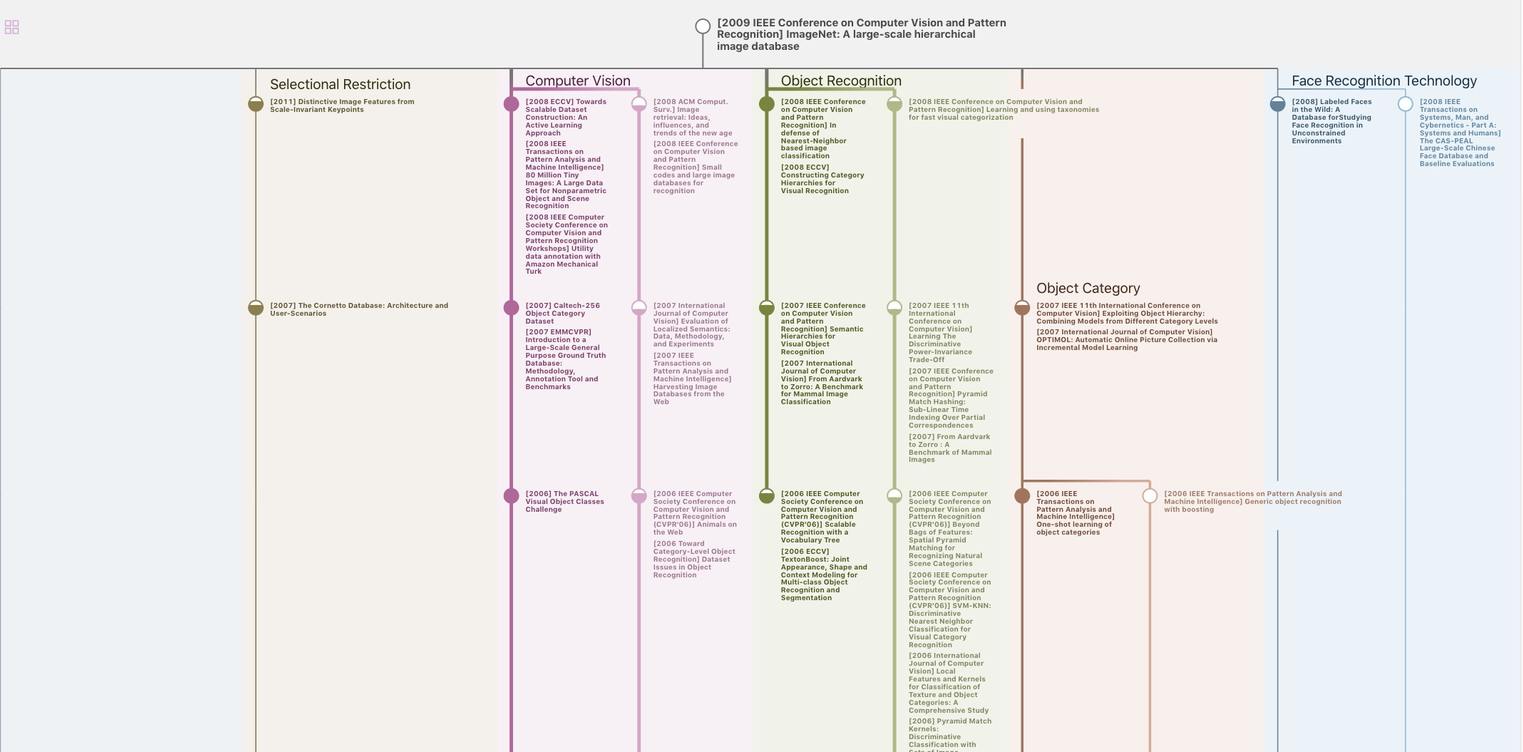
生成溯源树,研究论文发展脉络
Chat Paper
正在生成论文摘要