Off-Policy Interval Estimation with Lipschitz Value Iteration
NIPS 2020(2020)
摘要
Off-policy evaluation provides an essential tool for evaluating the effects of different policies or treatments using only observed data. When applied to high-stakes scenarios such as medical diagnosis or financial decision-making, it is crucial to provide provably correct upper and lower bounds of the expected reward, not just a classical single point estimate, to the end-users, as executing a poor policy can be very costly. In this work, we propose a provably correct method for obtaining interval bounds for off-policy evaluation in a general continuous setting. The idea is to search for the maximum and minimum values of the expected reward among all the Lipschitz Q-functions that are consistent with the observations, which amounts to solving a constrained optimization problem on a Lipschitz function space. We go on to introduce a Lipschitz value iteration method to monotonically tighten the interval, which is simple yet efficient and provably convergent. We demonstrate the practical efficiency of our method on a range of benchmarks.
更多查看译文
关键词
estimation,interval,off-policy
AI 理解论文
溯源树
样例
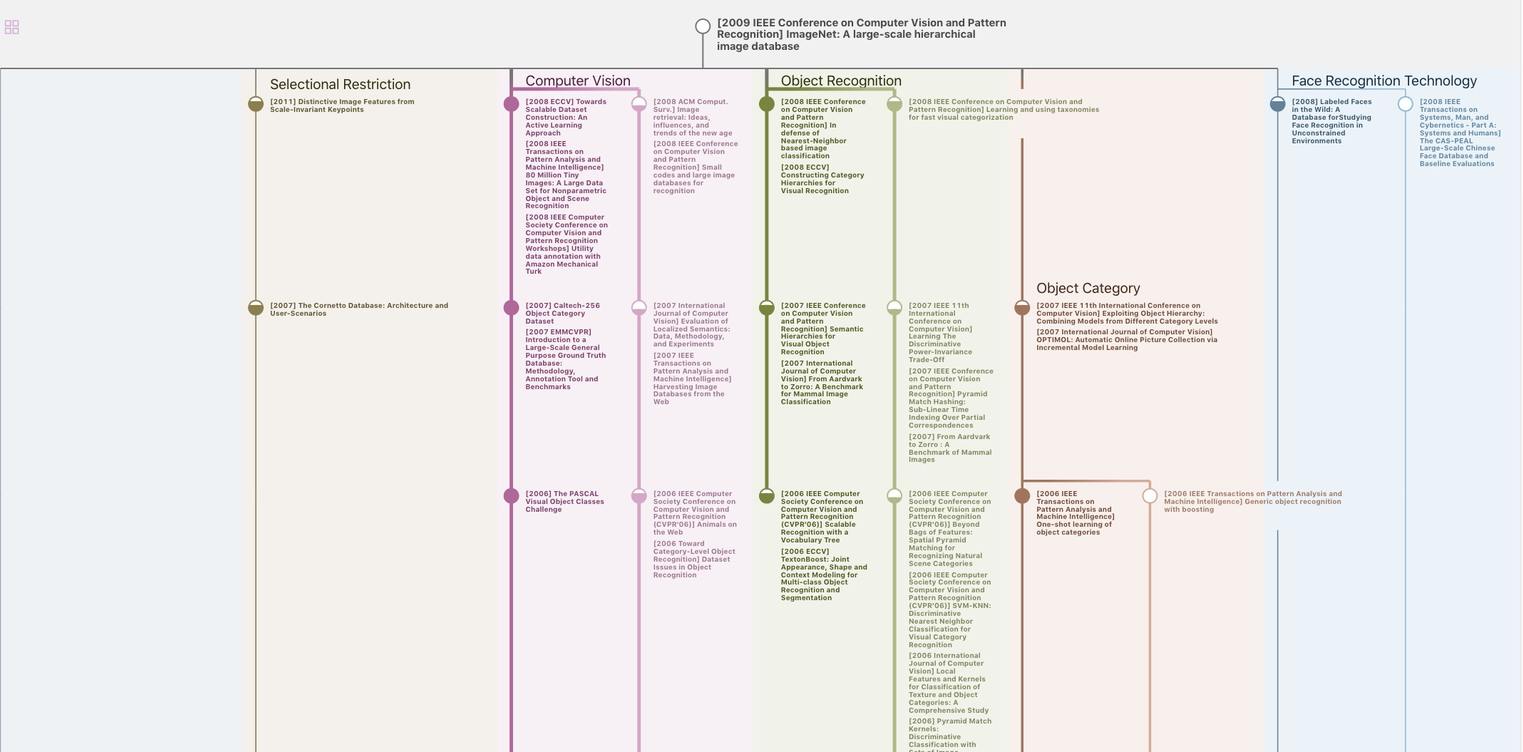
生成溯源树,研究论文发展脉络
Chat Paper
正在生成论文摘要