A Knowledge-Aware Sequence-to-Tree Network for Math Word Problem Solving
Conference on Empirical Methods in Natural Language Processing(2020)
摘要
With the advancements in natural language processing tasks, math word problem solving has received increasing attention. Previous methods have achieved promising results but ignore background common-sense knowledge not directly provided by the problem. In addition, during generation, they focus on local features while neglecting global information. To incorporate external knowledge and global expression information, we propose a novel knowledge-aware sequence-to-tree (KA-S2T) network in which the entities in the problem sequences and their categories are modeled as an entity graph. Based on this entity graph, a graph attention network is used to capture knowledge-aware problem representations. Further, we use a tree-structured decoder with a state aggregation mechanism to capture the long-distance dependency and global expression information. Experimental results on the Math23K dataset revealed that the KA-S2T model can achieve better performance than previously reported best results.
更多查看译文
关键词
math word problem solving,knowledge-aware,sequence-to-tree
AI 理解论文
溯源树
样例
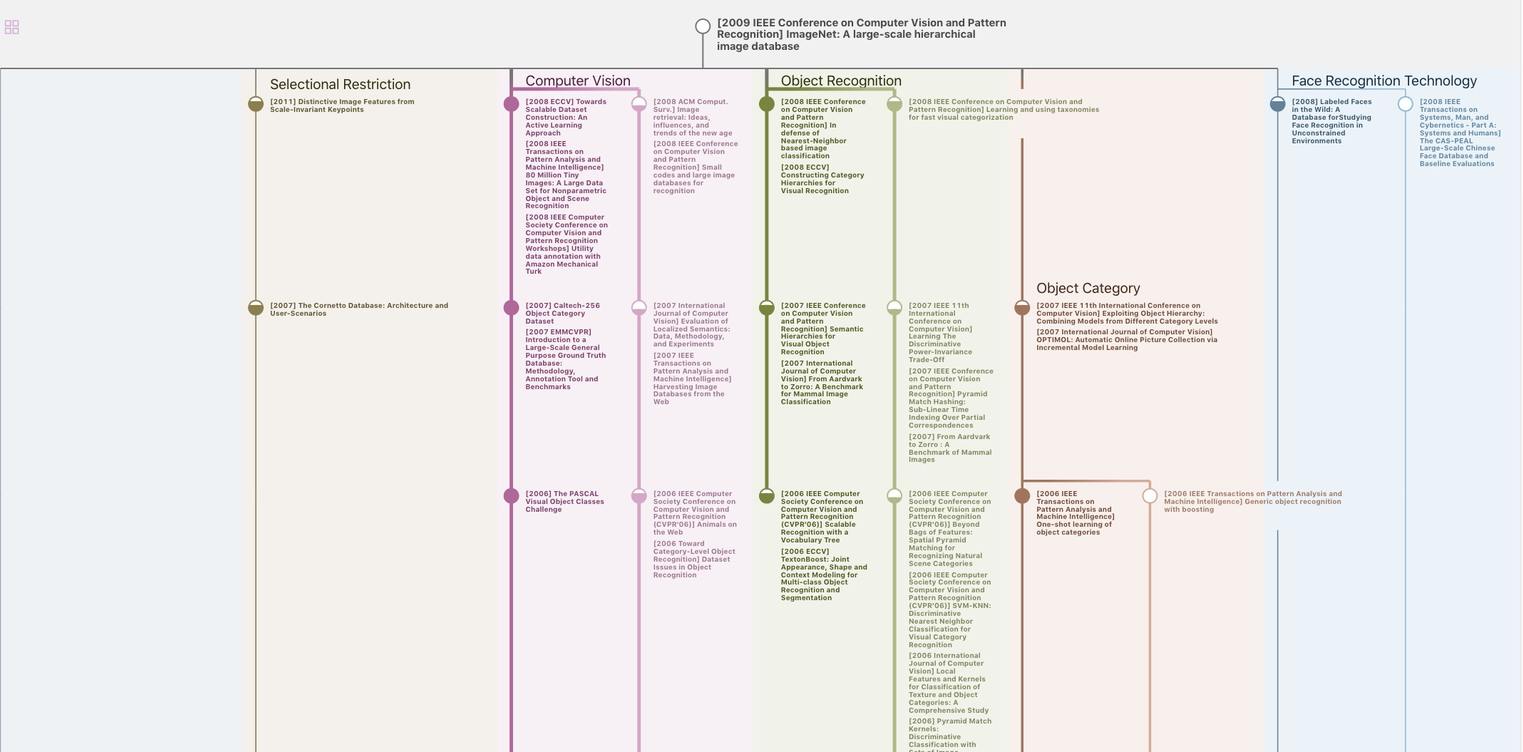
生成溯源树,研究论文发展脉络
Chat Paper
正在生成论文摘要