COGS: A Compositional Generalization Challenge Based on Semantic Interpretation
Conference on Empirical Methods in Natural Language Processing(2020)
摘要
Natural language is characterized by compositionality: the meaning of a complex expression is constructed from the meanings of its constituent parts. To facilitate the evaluation of the compositional abilities of language processing architectures, we introduce COGS, a semantic parsing dataset based on a fragment of English. The evaluation portion of COGS contains multiple systematic gaps that can only be addressed by compositional generalization; these include new combinations of familiar syntactic structures, or new combinations of familiar words and familiar structures. In experiments with Transformers and LSTMs, we found that in-distribution accuracy on the COGS test set was near-perfect (96–99%), but generalization accuracy was substantially lower (16–35%) and showed high sensitivity to random seed (+-6–8%). These findings indicate that contemporary standard NLP models are limited in their compositional generalization capacity, and position COGS as a good way to measure progress.
更多查看译文
关键词
compositional generalization challenge,semantic
AI 理解论文
溯源树
样例
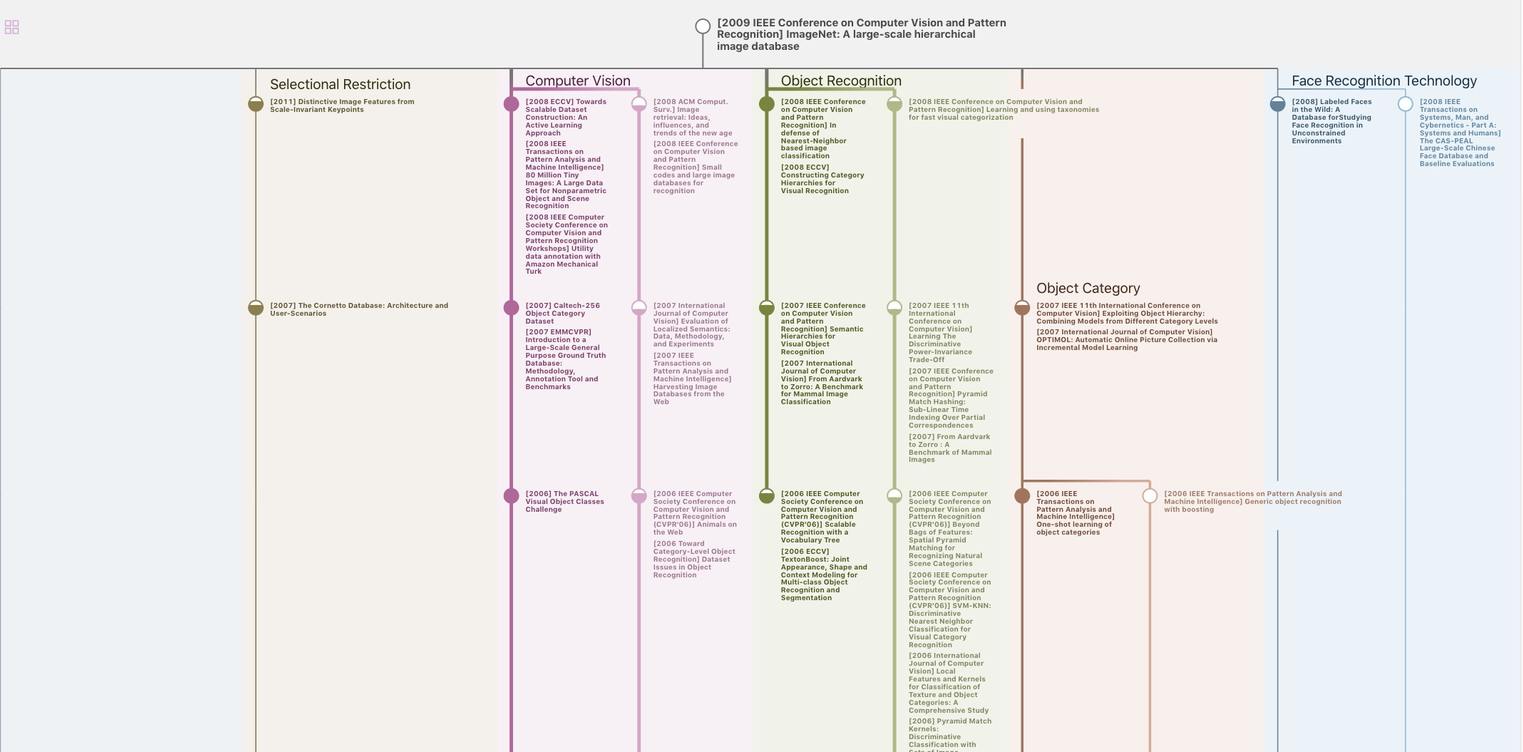
生成溯源树,研究论文发展脉络
Chat Paper
正在生成论文摘要