Discriminatively-Tuned Generative Classifiers for Robust Natural Language Inference
Conference on Empirical Methods in Natural Language Processing(2020)
摘要
While discriminative neural network classifiers are generally preferred, recent work has shown advantages of generative classifiers in term of data efficiency and robustness. In this paper, we focus on natural language inference (NLI). We propose GenNLI, a generative classifier for NLI tasks, and empirically characterize its performance by comparing it to five baselines, including discriminative models and large-scale pretrained language representation models like BERT. We explore training objectives for discriminative fine-tuning of our generative classifiers, showing improvements over log loss fine-tuning from prior work (Lewis and Fan, 2019). In particular, we find strong results with a simple unbounded modification to log loss, which we call the “infinilog loss”. Our experiments show that GenNLI outperforms both discriminative and pretrained baselines across several challenging NLI experimental settings, including small training sets, imbalanced label distributions, and label noise.
更多查看译文
关键词
generative classifiers,robust natural
AI 理解论文
溯源树
样例
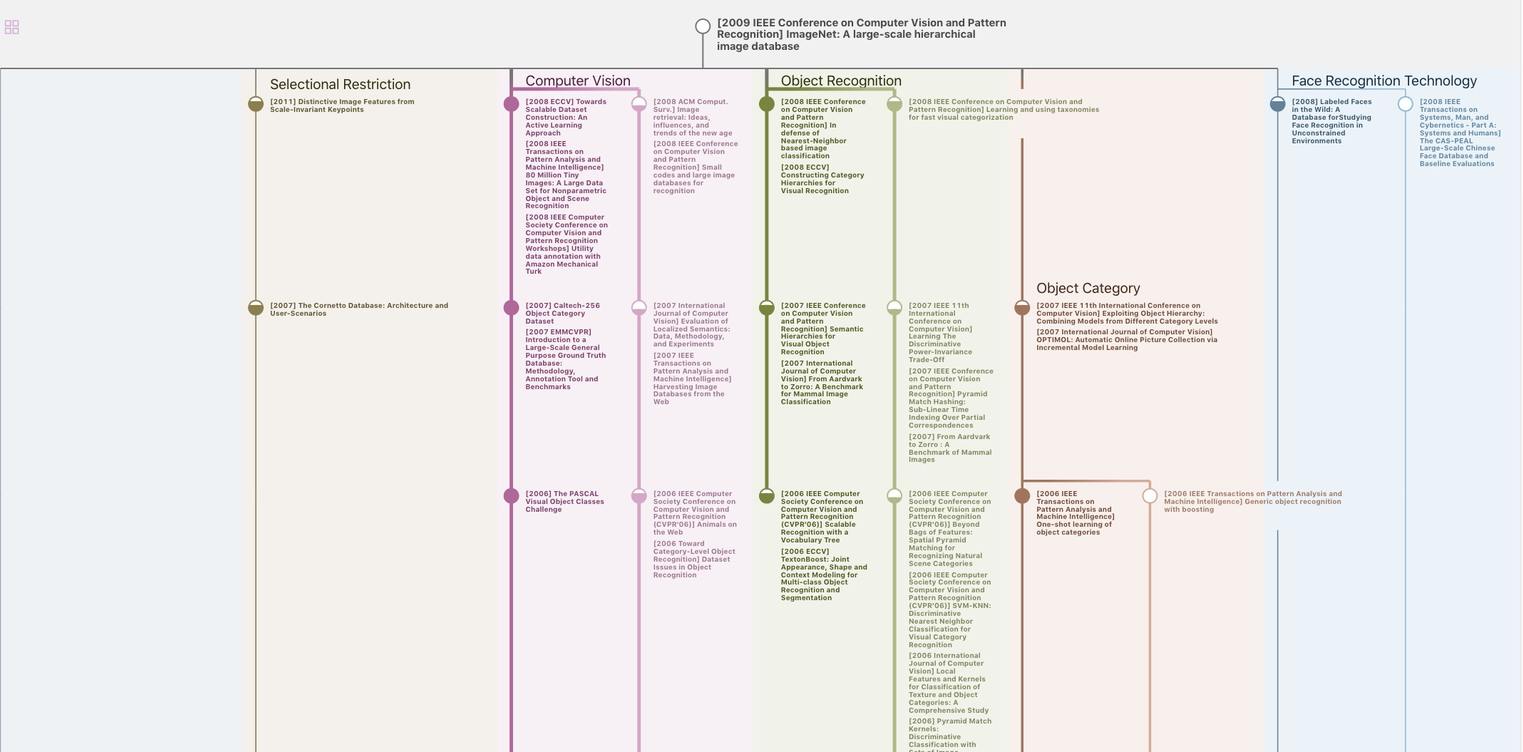
生成溯源树,研究论文发展脉络
Chat Paper
正在生成论文摘要