Few-Shot Complex Knowledge Base Question Answering via Meta Reinforcement Learning
Conference on Empirical Methods in Natural Language Processing(2020)
摘要
Complex question-answering (CQA) involves answering complex natural-language questions on a knowledge base (KB). However, the conventional neural program induction (NPI) approach exhibits uneven performance when the questions have different types, harboring inherently different characteristics, e.g., difficulty level. This paper proposes a meta-reinforcement learning approach to program induction in CQA to tackle the potential distributional bias in questions. Our method quickly and effectively adapts the meta-learned programmer to new questions based on the most similar questions retrieved from the training data. The meta-learned policy is then used to learn a good programming policy, utilizing the trial trajectories and their rewards for similar questions in the support set. Our method achieves state-of-the-art performance on the CQA dataset (Saha et al., 2018) while using only five trial trajectories for the top-5 retrieved questions in each support set, and meta-training on tasks constructed from only 1% of the training set. We have released our code at https://github.com/DevinJake/MRL-CQA.
更多查看译文
关键词
reinforcement learning,knowledge,complex,few-shot
AI 理解论文
溯源树
样例
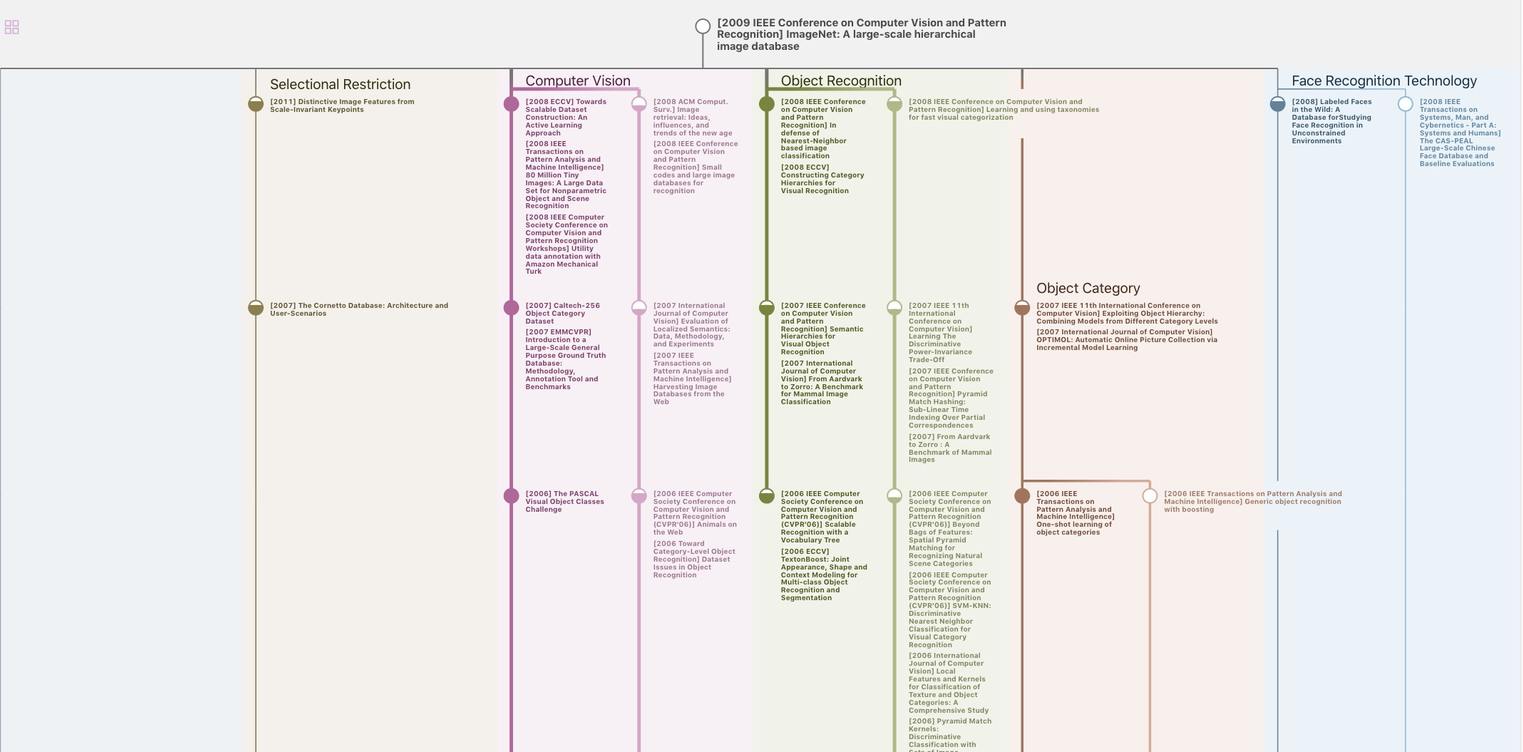
生成溯源树,研究论文发展脉络
Chat Paper
正在生成论文摘要