When Hearst Is not Enough: Improving Hypernymy Detection from Corpus with Distributional Models
Conference on Empirical Methods in Natural Language Processing(2020)
摘要
We address hypernymy detection, i.e., whether an is-a relationship exists between words (x ,y), with the help of large textual corpora. Most conventional approaches to this task have been categorized to be either pattern-based or distributional. Recent studies suggest that pattern-based ones are superior, if large-scale Hearst pairs are extracted and fed, with the sparsity of unseen (x ,y) pairs relieved. However, they become invalid in some specific sparsity cases, where x or y is not involved in any pattern. For the first time, this paper quantifies the non-negligible existence of those specific cases. We also demonstrate that distributional methods are ideal to make up for pattern-based ones in such cases. We devise a complementary framework, under which a pattern-based and a distributional model collaborate seamlessly in cases which they each prefer. On several benchmark datasets, our framework demonstrates improvements that are both competitive and explainable.
更多查看译文
关键词
hypernymy detection,corpus
AI 理解论文
溯源树
样例
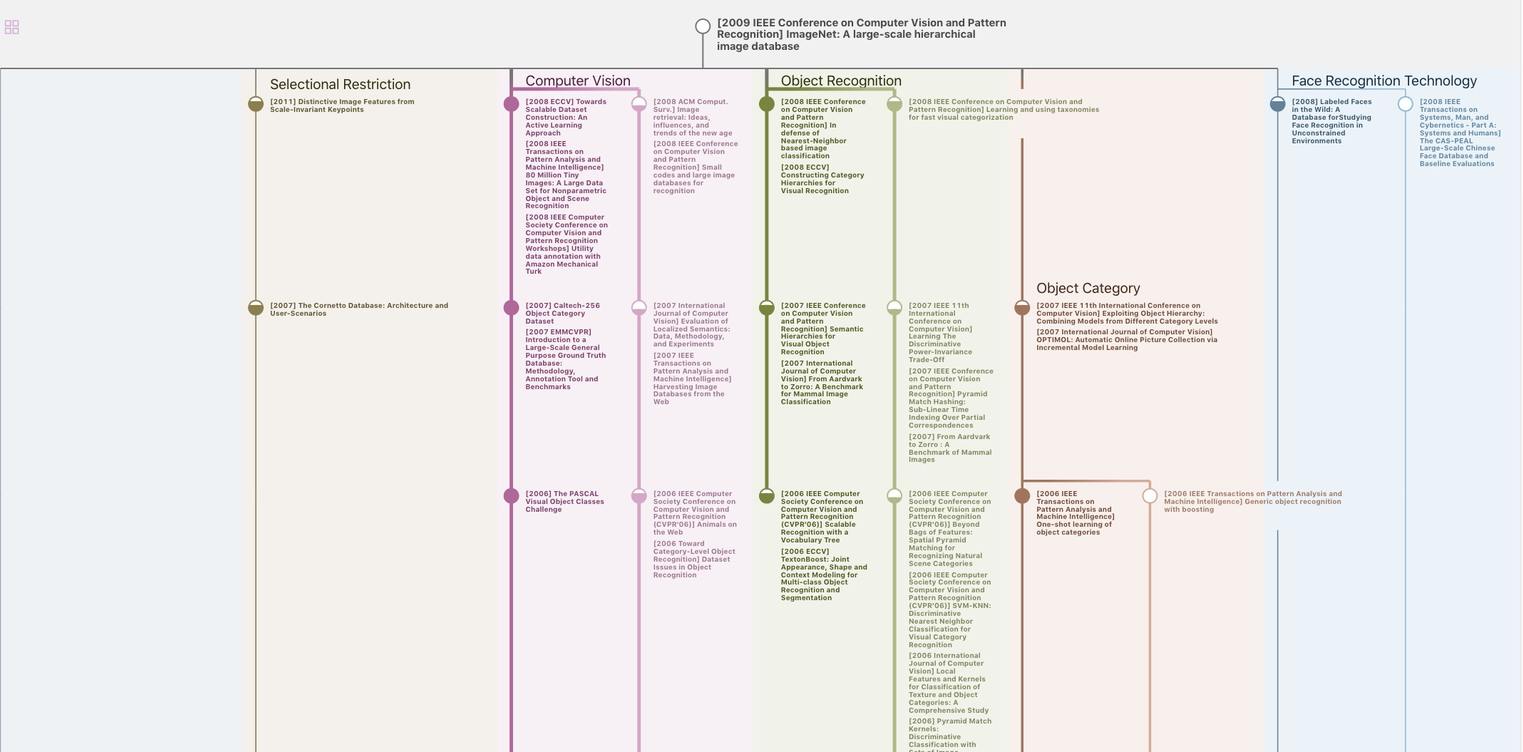
生成溯源树,研究论文发展脉络
Chat Paper
正在生成论文摘要