Improved Pomdp Tree Search Planning With Prioritized Action Branching
THIRTY-FIFTH AAAI CONFERENCE ON ARTIFICIAL INTELLIGENCE, THIRTY-THIRD CONFERENCE ON INNOVATIVE APPLICATIONS OF ARTIFICIAL INTELLIGENCE AND THE ELEVENTH SYMPOSIUM ON EDUCATIONAL ADVANCES IN ARTIFICIAL INTELLIGENCE(2021)
摘要
Online solvers for partially observable Markov decision processes have difficulty scaling to problems with large action spaces. This paper proposes a method called PA-POMCPOW to sample a subset of the action space for inclusion in a search tree. The proposed method first evaluates the action space according to a score function that is a linear combination of expected reward and expected information gain. The actions with the highest score are then added to the search tree during tree expansion. Using this score function, actions providing the highest expected mixture of exploration and exploitation are included in the tree. Experiments show that PA-POMCPOW is able to outperform existing state-of-theart solvers on problems with large discrete action spaces.
更多查看译文
关键词
planning,prioritized action,search
AI 理解论文
溯源树
样例
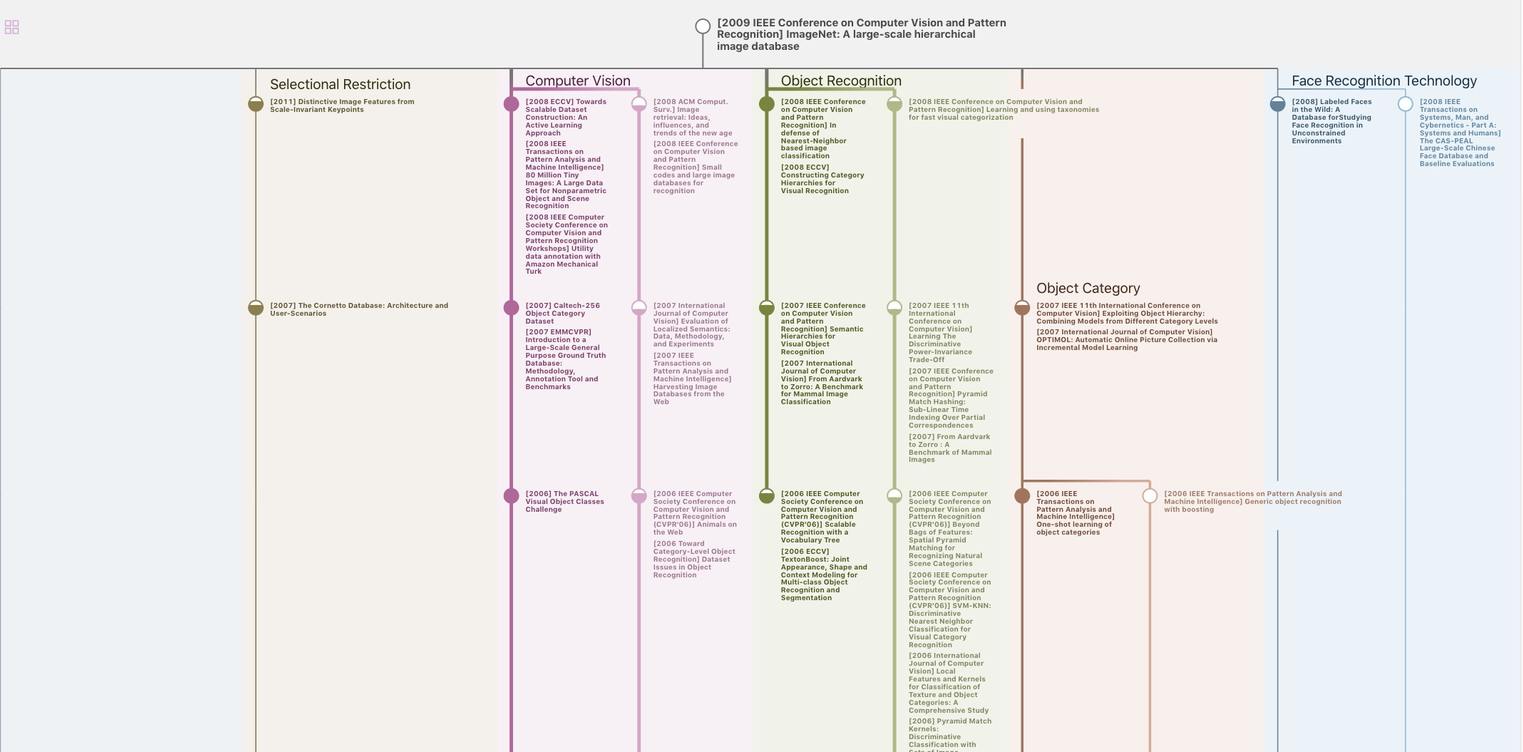
生成溯源树,研究论文发展脉络
Chat Paper
正在生成论文摘要