Theoretical Analysis of Self-Training with Deep Networks on Unlabeled Data
ICLR(2021)
摘要
Self-training algorithms, which train a model to fit pseudolabels predicted by another previously-learned model, have been very successful for learning with unlabeled data using neural networks. However, the current theoretical understanding of self-training only applies to linear models. This work provides a unified theoretical analysis of self-training with deep networks for semi-supervised learning, unsupervised domain adaptation, and unsupervised learning. At the core of our analysis is a simple but realistic "expansion" assumption, which states that a low-probability subset of the data must expand to a neighborhood with large probability relative to the subset. We also assume that neighborhoods of examples in different classes have minimal overlap. We prove that under these assumptions, the minimizers of population objectives based on self-training and input-consistency regularization will achieve high accuracy with respect to ground-truth labels. By using off-the-shelf generalization bounds, we immediately convert this result to sample complexity guarantees for neural nets that are polynomial in the margin and Lipschitzness. Our results help explain the empirical successes of recently proposed self-training algorithms which use input consistency regularization.
更多查看译文
关键词
unlabeled
AI 理解论文
溯源树
样例
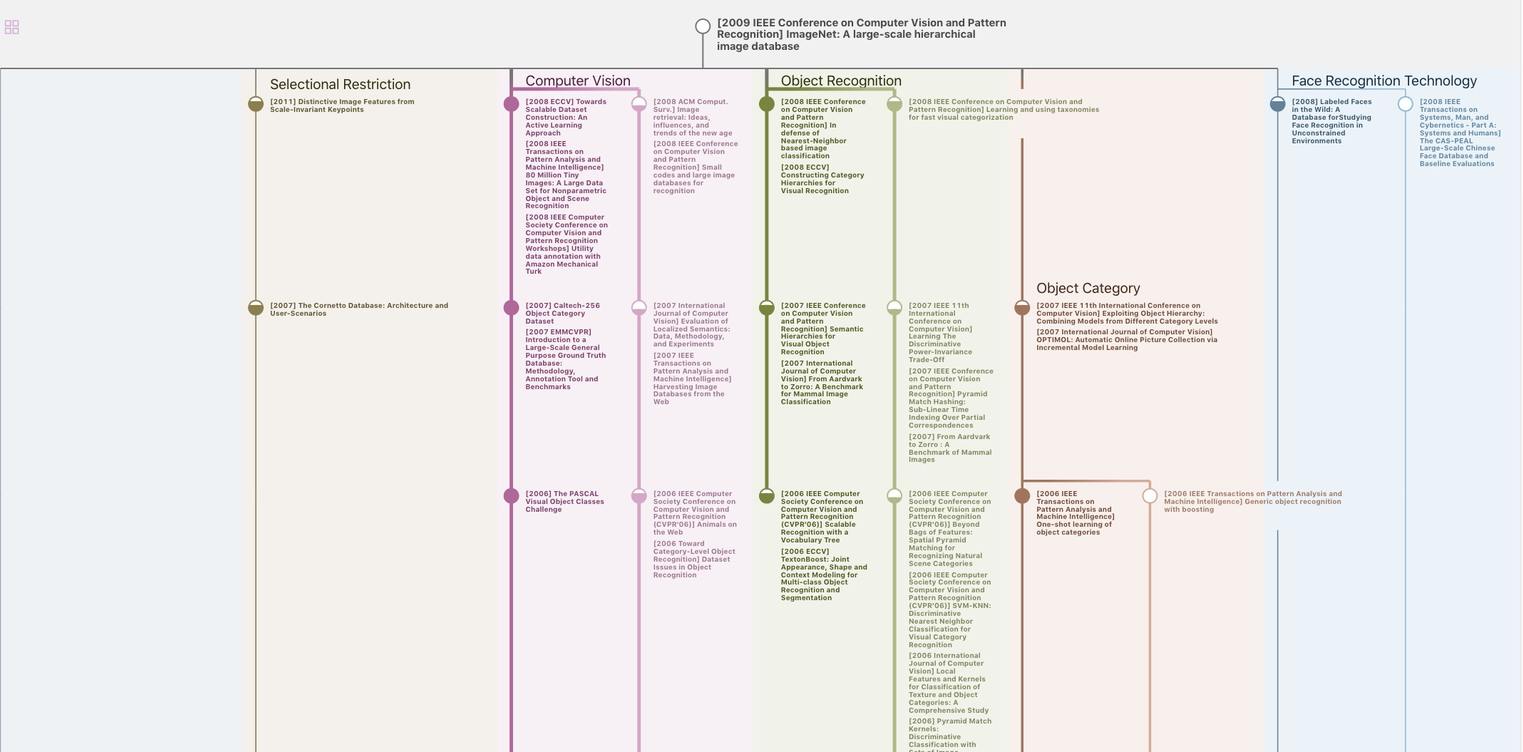
生成溯源树,研究论文发展脉络
Chat Paper
正在生成论文摘要