A Theoretical Analysis Of Catastrophic Forgetting Through The Ntk Overlap Matrix
24TH INTERNATIONAL CONFERENCE ON ARTIFICIAL INTELLIGENCE AND STATISTICS (AISTATS)(2021)
摘要
Continual learning (CL) is a setting in which an agent has to learn from an incoming stream of data during its entire lifetime. Although major advances have been made in the field, one recurring problem which remains unsolved is that of Catastrophic Forgetting (CF). While the issue has been extensively studied empirically, little attention has been paid from a theoretical angle. In this paper, we show that the impact of CF increases as two tasks increasingly align. We introduce a measure of task similarity called the NTK overlap matrix which is at the core of CF. We analyze common projected gradient algorithms and demonstrate how they mitigate forgetting. Then, we propose a variant of Orthogonal Gradient Descent (OGD) which leverages structure of the data through Principal Component Analysis (PCA). Experiments support our theoretical findings and show how our method can help reduce CF on classical CL datasets.
更多查看译文
关键词
catastrophic forgetting
AI 理解论文
溯源树
样例
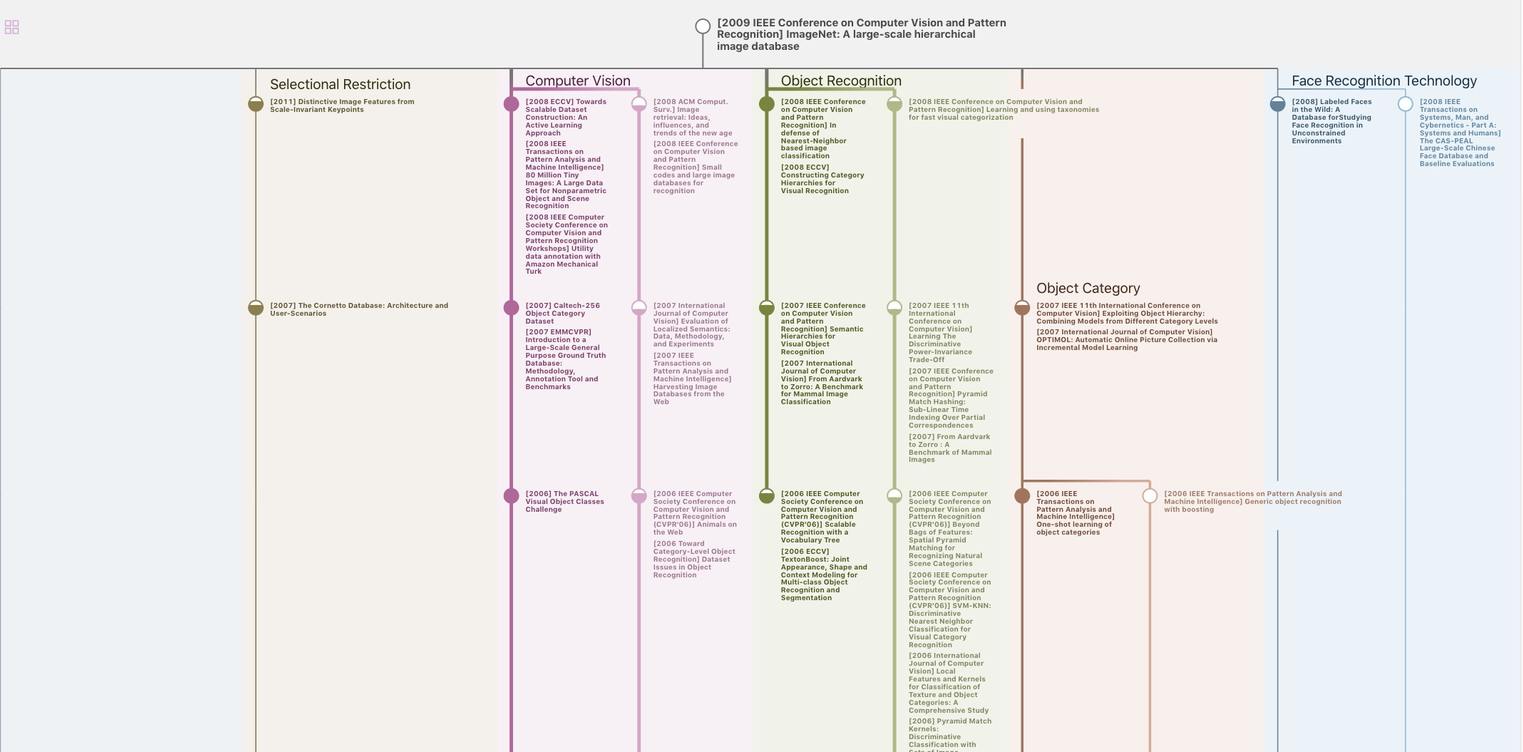
生成溯源树,研究论文发展脉络
Chat Paper
正在生成论文摘要