Incorporating dose–volume histogram parameters of swallowing organs at risk in a videofluoroscopy-based predictive model of radiation-induced dysphagia after head and neck cancer intensity-modulated radiation therapy
STRAHLENTHERAPIE UND ONKOLOGIE(2020)
摘要
Purpose To develop a videofluoroscopy-based predictive model of radiation-induced dysphagia (RID) by incorporating DVH parameters of swallowing organs at risk (SWOARs) in a machine learning analysis. Methods Videofluoroscopy (VF) was performed to assess the penetration-aspiration score (P/A) at baseline and at 6 and 12 months after RT. An RID predictive model was developed using dose to nine SWOARs and P/A-VF data at 6 and 12 months after treatment. A total of 72 dosimetric features for each patient were extracted from DVH and analyzed with linear support vector machine classification (SVC), logistic regression classification (LRC), and random forest classification (RFC). Results 38 patients were evaluable. The relevance of SWOARs DVH features emerged both at 6 months (AUC 0.82 with SVC; 0.80 with LRC; and 0.83 with RFC) and at 12 months (AUC 0.85 with SVC; 0.82 with LRC; and 0.94 with RFC). The SWOARs and the corresponding features with the highest relevance at 6 months resulted as the base of tongue (V65 and D mean ), the superior (D mean ) and medium constrictor muscle (V45, V55; V65; D mp ; D mean ; D max and D min ), and the parotid glands (D mean and D mp ). On the contrary, the features with the highest relevance at 12 months were the medium (V55; D min and D mean ) and inferior constrictor muscles (V55, V65 D min and D max ), the glottis (V55 and D max ), the cricopharyngeal muscle (D max ), and the cervical esophagus (D max ). Conclusion We trained and cross-validated an RID predictive model with high discriminative ability at both 6 and 12 months after RT. We expect to improve the predictive power of this model by enlarging the number of training datasets.
更多查看译文
关键词
Machine learning machines,Normal tissue complication probability,Aspiration,Deglutition,Radiotherapy
AI 理解论文
溯源树
样例
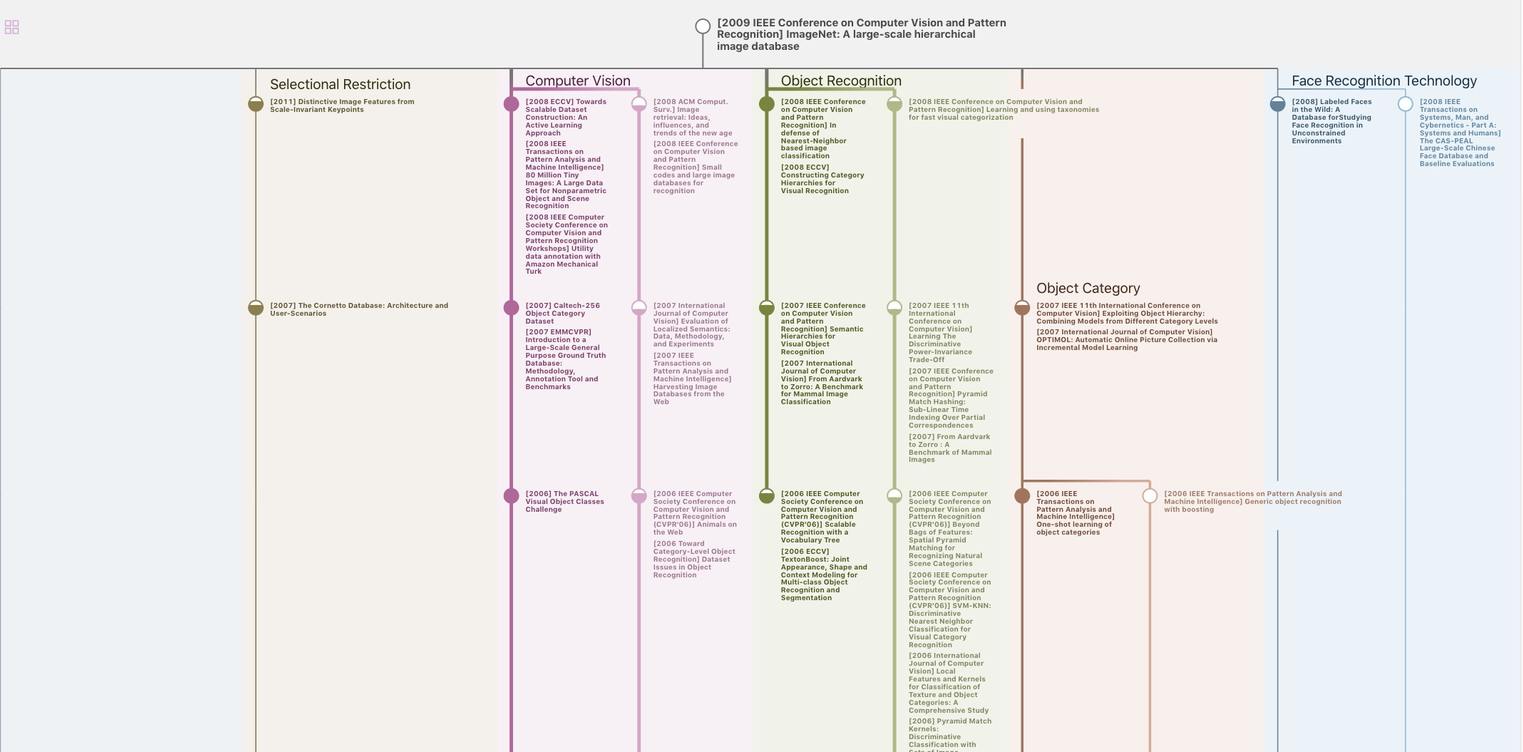
生成溯源树,研究论文发展脉络
Chat Paper
正在生成论文摘要