No MCMC for me: Amortized sampling for fast and stable training of energy-based models
ICLR(2020)
摘要
Energy-Based Models (EBMs) present a flexible and appealing way to representuncertainty. Despite recent advances, training EBMs on high-dimensional dataremains a challenging problem as the state-of-the-art approaches are costly, unstable, and require considerable tuning and domain expertise to apply successfully. In this work we present a simple method for training EBMs at scale which uses an entropy-regularized generator to amortize the MCMC sampling typically usedin EBM training. We improve upon prior MCMC-based entropy regularization methods with a fast variational approximation. We demonstrate the effectiveness of our approach by using it to train tractable likelihood models. Next, we apply our estimator to the recently proposed Joint Energy Model (JEM), where we matchthe original performance with faster and stable training. This allows us to extend JEM models to semi-supervised classification on tabular data from a variety of continuous domains.
更多查看译文
AI 理解论文
溯源树
样例
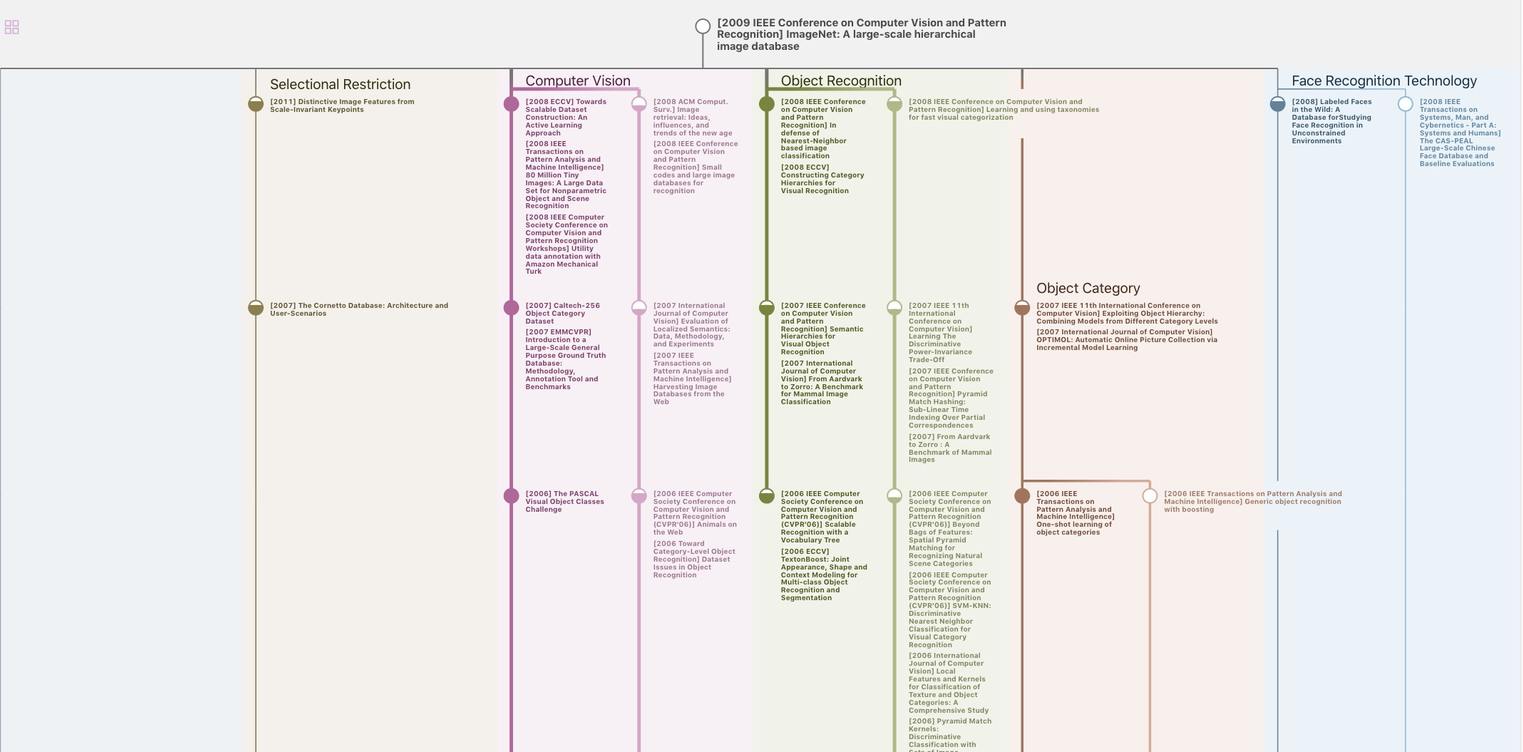
生成溯源树,研究论文发展脉络
Chat Paper
正在生成论文摘要