Deep Learning Superpixel Semantic Segmentation with Transparent Initialization and Sparse Encoder
arxiv(2020)
摘要
Even though deep learning greatly improves the performance of semantic segmentation, its success mainly lies on object central areas but without accurate edges. As superpixel is a popular and effective auxiliary to preserve object edges, in this paper, we jointly learn semantic segmentation with trainable superpixels. We achieve it by adding fully-connected layers with transparent initialization and an efficient logit uniformization with a sparse encoder. Specifically, the proposed transparent initialization reserves the effects of learned parameters from pretrained networks, one for semantic segmentation and the other for superpixel, by a linear data recovery. This avoids a significant loss increase by using the pretrained networks, which otherwise can be caused by an inappropriate parameter initialization on the added layers. Meanwhile, consistent assignments to all pixels in each superpixel can be guaranteed by the logit uniformization with a sparse encoder. This sparse encoder with sparse matrix operations substantially improves the training efficiency by reducing the large computational complexity arising from indexing pixels by superpixels. We demonstrate the effectiveness of our proposal by transparent initialization and sparse encoder on semantic segmentation on PASCAL VOC 2012 dataset with enhanced labeling on the object edges. Moreover, the proposed transparent initialization can also be used to jointly finetune multiple or a deeper pretrained network on other tasks.
更多查看译文
关键词
segmentation,deep learning,transparent initialization
AI 理解论文
溯源树
样例
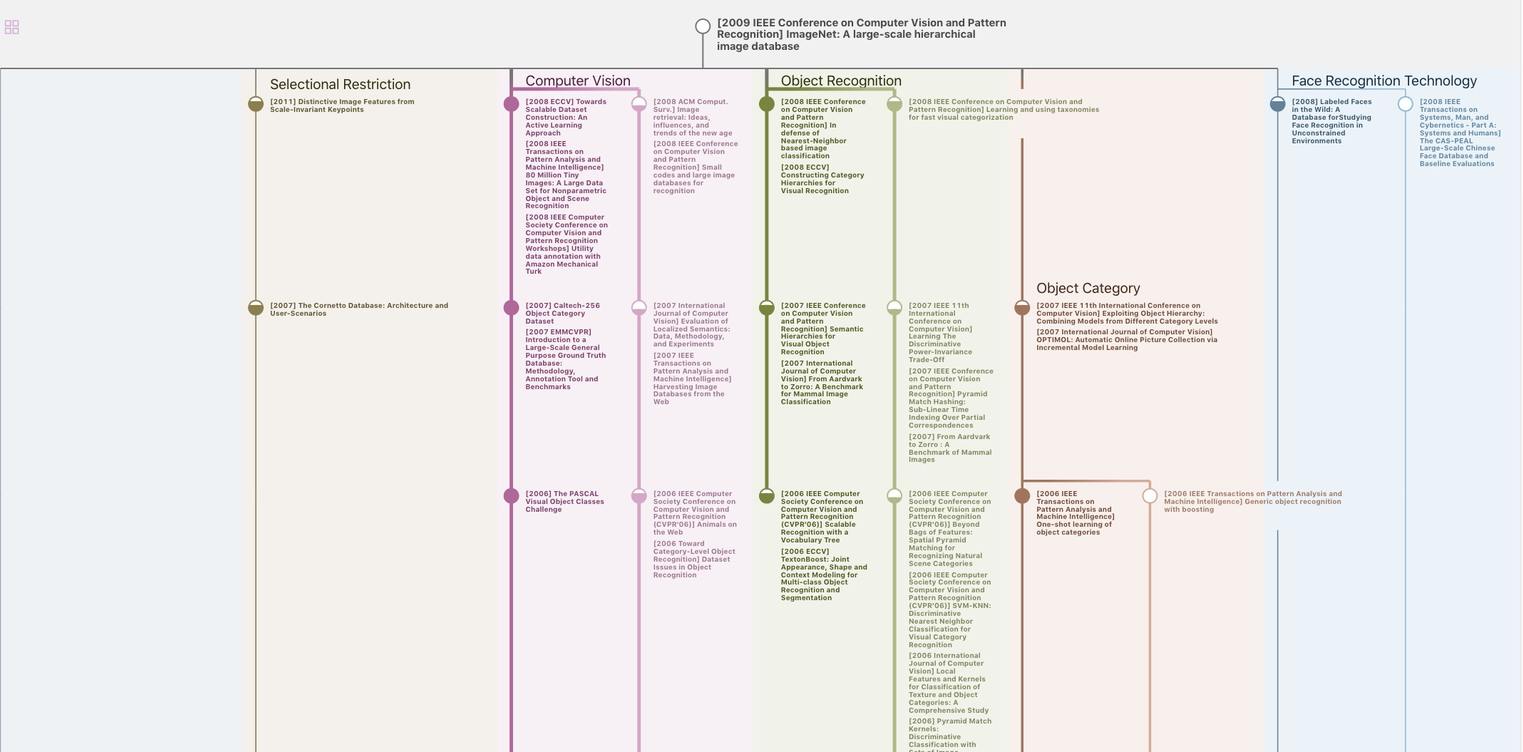
生成溯源树,研究论文发展脉络
Chat Paper
正在生成论文摘要