Regularizing linear inverse problems under unknown non-Gaussian white noise allowing repeated measurements
arxiv(2023)
摘要
We deal with the solution of a generic linear inverse problem in the Hilbert space setting. The exact right-hand side is unknown and only accessible through discretized measurements corrupted by white noise with unknown arbitrary distribution. The measuring process can be repeated, which allows to reduce and estimate the measurement error through averaging. We show convergence against the true solution of the infinite-dimensional problem for a priori and a posteriori regularization schemes as the number of measurements and the dimension of the discretization tend to infinity under natural and easily verifiable conditions for the discretization.
更多查看译文
关键词
statistical inverse problems,discretization,white noise,discrepancy principle
AI 理解论文
溯源树
样例
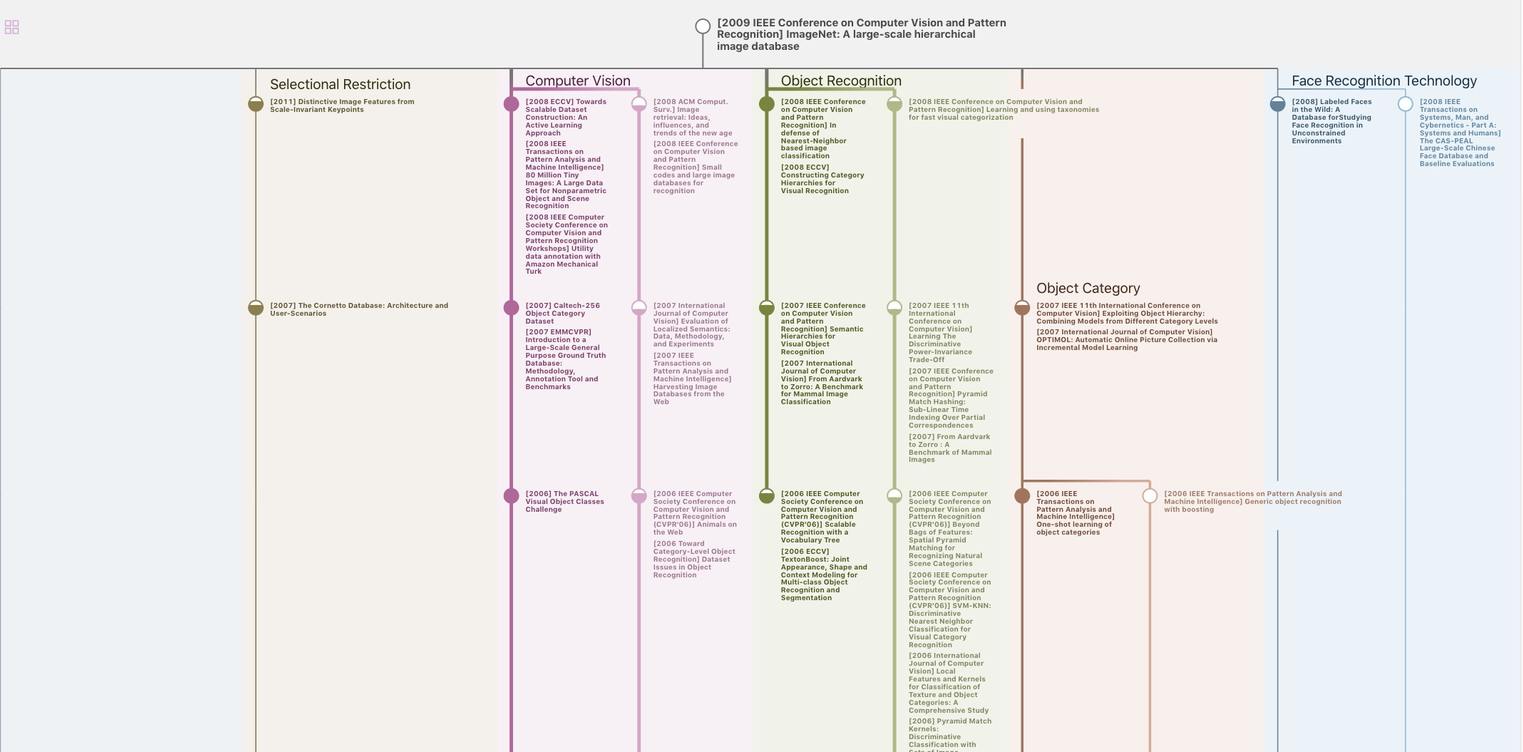
生成溯源树,研究论文发展脉络
Chat Paper
正在生成论文摘要