Meta Graph Attention on Heterogeneous Graph with Node-Edge Co-evolution
arxiv(2020)
摘要
Graph neural networks have become an important tool for modeling structured data. In many real-world systems, intricate hidden information may exist, e.g., heterogeneity in nodes/edges, static node/edge attributes, and spatiotemporal node/edge features. However, most existing methods only take part of the information into consideration. In this paper, we present the Co-evolved Meta Graph Neural Network (CoMGNN), which applies meta graph attention to heterogeneous graphs with co-evolution of node and edge states. We further propose a spatiotemporal adaption of CoMGNN (ST-CoMGNN) for modeling spatiotemporal patterns on nodes and edges. We conduct experiments on two large-scale real-world datasets. Experimental results show that our models significantly outperform the state-of-the-art methods, demonstrating the effectiveness of encoding diverse information from different aspects.
更多查看译文
关键词
heterogeneous graph,attention,meta,node-edge,co-evolution
AI 理解论文
溯源树
样例
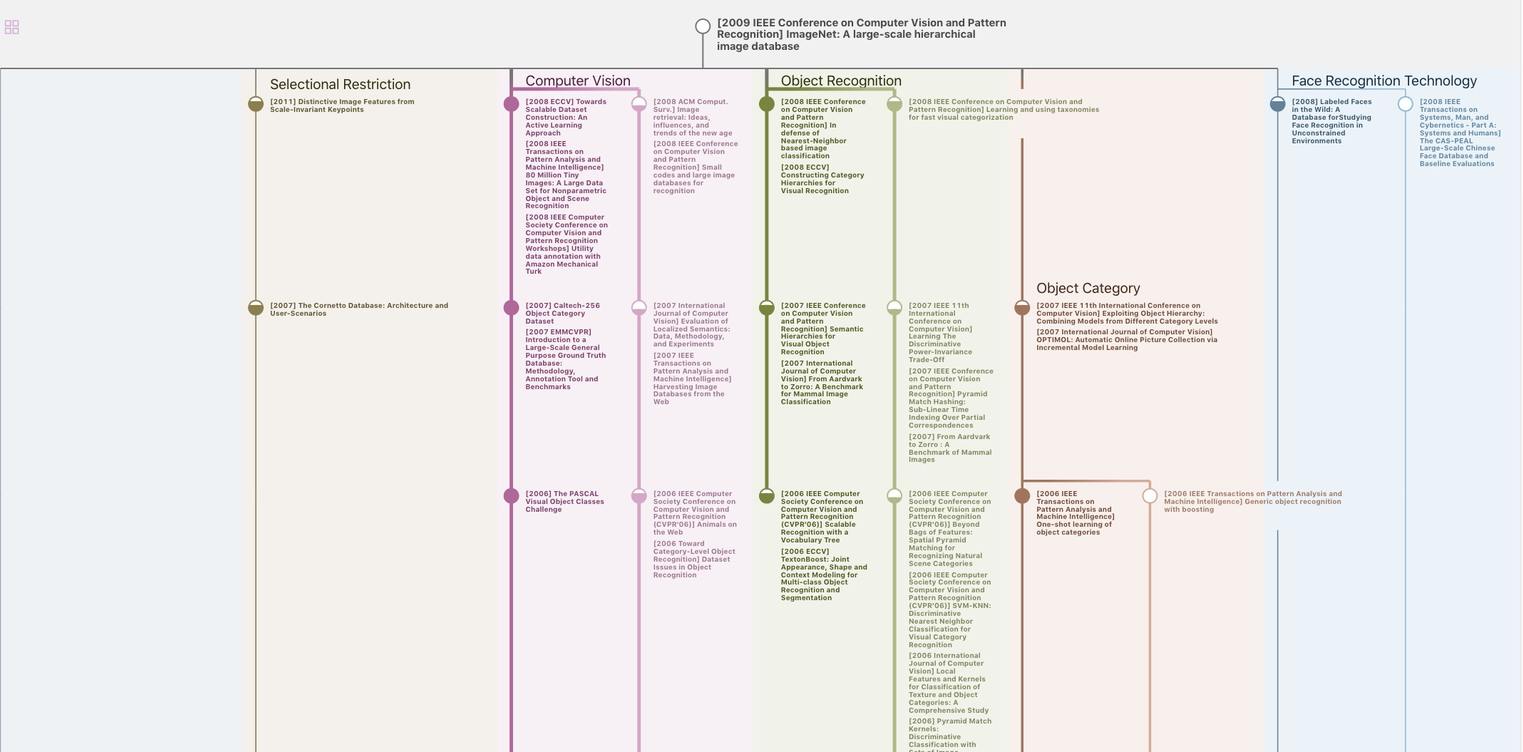
生成溯源树,研究论文发展脉络
Chat Paper
正在生成论文摘要