MAPPING CROP CONDITION USING QUICKBIRD-2 AND WORLDVIEW-1 SATELLITE IMAGES AND DERIVED PRODUCTS. A PRECISION AGRICULTURE CASE STUDY FOR PART OF ZHITEN TEST SITE IN NORTHEAST BULGARIA
AEROSPACE RESEARCH IN BULGARIA(2015)
摘要
The purpose of this article is to investigate the possibilities of mapping crop condition using high resolution (HR) satellite images for Zhiten test site situated in Northeast Bulgaria. The chosen satellite images are acquired from multispectral QuickBird-2 and panchromatic WorldView-1 sensors on 31/05/2009 and 30/11/2011, respectively. The methodology of this article includes the following working stages: 1) applying arable mask from CORINE 2006 land-cover database; 2) conducting per-pixel supervised classification using the Maximum Likelihood Classifier (MLC) algorithm for crop identification; 3) applying accuracy assessment tool in ERDAS Imagine and deriving accuracy totals and Kappa statistics; 4) mapping crop condition using Normalized Difference Vegetation Index (NDVI), Renormalized Difference Vegetation Index (RDVI) and Soil Adjusted Vegetation Index (SAVI) indices. The overall classification accuracy for the QuickBird-2 image is 90.86 % and overall Kappa statistics is 0.8538, while for the WorldView-1 image is 86.71 % and overall Kappa statistics is 0.7721. The SAVI shows better sensitivity for the spring crops cultivars with less than 40 % vegetation cover. Meanwhile, the NDVI gives good results for winter crops, but saturates at high vegetation dencity and gives generalized results for values less than 0.40. Overall RDVI gives better crop condition results for winter crops at flowering and grain filling phenophases and spring crops at vegetative phase compared to NDVI and SAVI.
更多查看译文
AI 理解论文
溯源树
样例
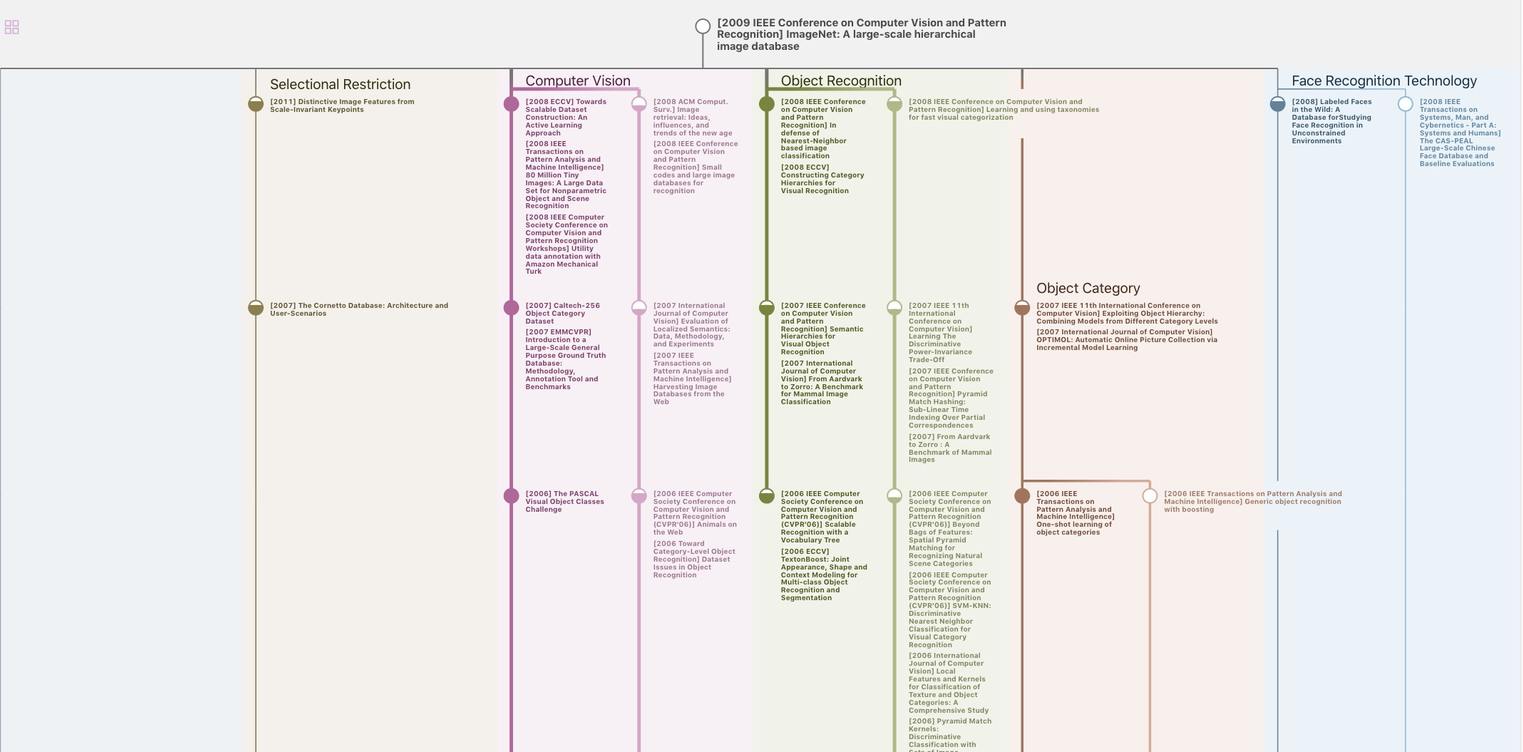
生成溯源树,研究论文发展脉络
Chat Paper
正在生成论文摘要