Data-centric framework for crystal structure identification in atomistic simulations using machine learning
PHYSICAL REVIEW MATERIALS(2022)
摘要
Atomic-level modeling performed at large scales enables the investigation of mesoscale materials properties with atom-by-atom resolution. The spatial complexity of such cross-scale simulations renders them unsuitable for simple human visual inspection. Instead, specialized structure characterization techniques are required to aid interpretation. These have historically been challenging to construct, requiring significant intuition and effort. Here we propose an alternative framework for a fundamental structural characterization task: classifying atoms according to the crystal structure to which they belong. Our approach is data-centric and favors the employment of Machine Learning over heuristic rules of classification. A group of data-science tools and simple local descriptors of atomic structure are employed together with an efficient synthetic training set. We also introduce the first standard and publicly available benchmark data set for evaluation of algorithms for crystal-structure classification. It is demonstrated that our data-centric framework outperforms all of the most popular heuristic methods-especially at high temperatures when lattices are the most distorted-while introducing a systematic route for generalization to new crystal structures. Moreover, through the use of outlier detection algorithms our approach is capable of discerning between amorphous atomic motifs (i.e., noncrystalline phases) and unknown crystal structures, making it uniquely suited for exploratory materials synthesis simulations.
更多查看译文
关键词
crystal structure identification,atomistic simulations,crystal structure,machine learning,data-centric
AI 理解论文
溯源树
样例
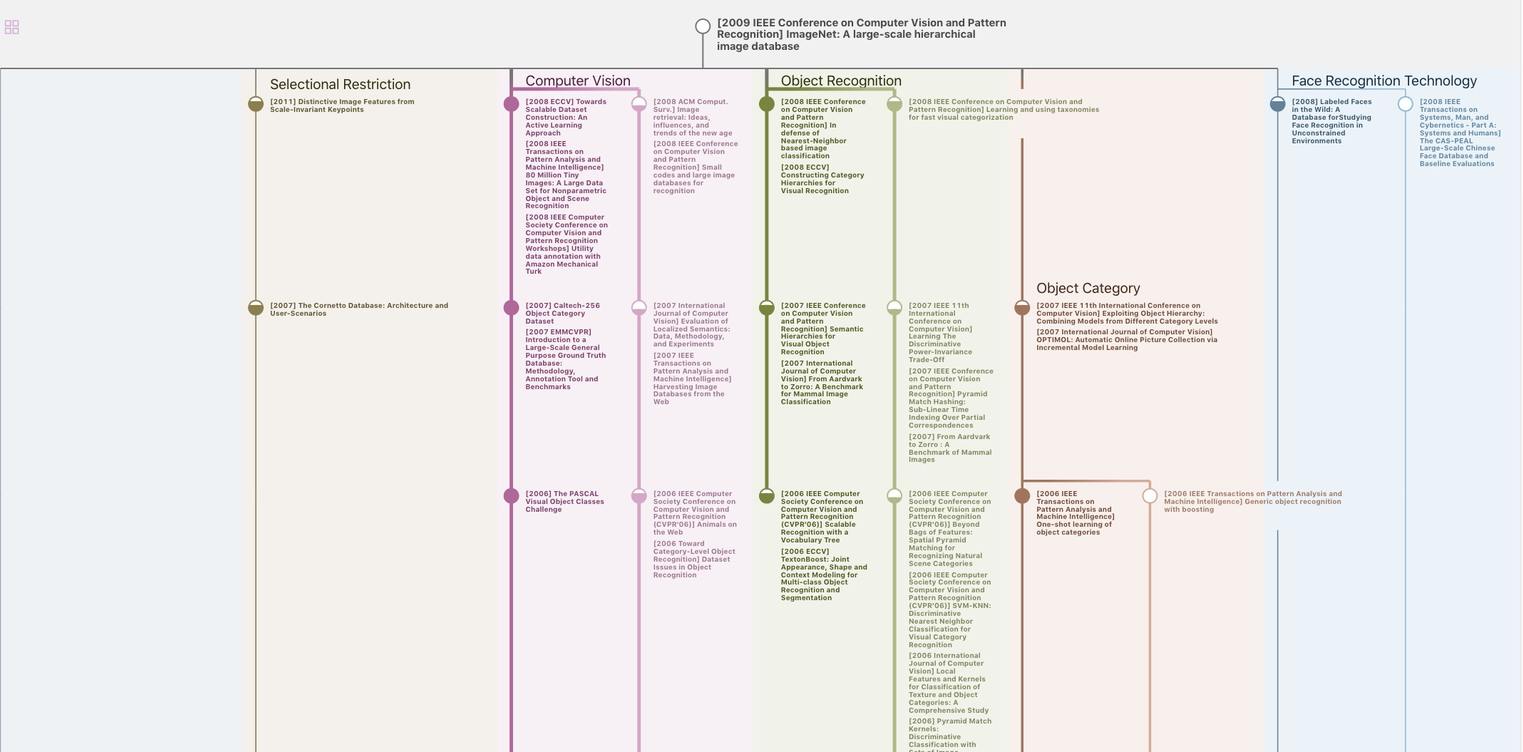
生成溯源树,研究论文发展脉络
Chat Paper
正在生成论文摘要