Pay Attention Selectively and Comprehensively: Pyramid Gating Network for Human Pose Estimation without Pre-training
MM '20: The 28th ACM International Conference on Multimedia Seattle WA USA October, 2020(2020)
摘要
Deep neural network with multi-scale feature fusion has achieved great success in human pose estimation. However, drawbacks still exist in these methods: 1) they consider multi-scale features equally, which may over-emphasize redundant features; 2) preferring deeper structures, they can learn features with the strong semantic representation, but tend to lose natural discriminative information; 3) to attain good performance, they rely heavily on pretraining, which is time-consuming, or even unavailable practically. To mitigate these problems, we propose a novel comprehensive recalibration model called Pyramid GAting Network (PGA-Net) that is capable of distillating, selecting, and fusing the discriminative and attention-aware features at different scales and different levels (i.e., both semantic and natural levels). Meanwhile, focusing on fusing features both selectively and comprehensively, PGA-Net can demonstrate remarkable stability and encouraging performance even without pre-training, making the model can be trained truly from scratch. We demonstrate the effectiveness of PGA-Net through validating on COCO and MPII benchmarks, attaining new state-of-the-art performance. https://github.com/ssr0512/PGA-Net
更多查看译文
关键词
pyramid gating system, stabilization, human pose estimation
AI 理解论文
溯源树
样例
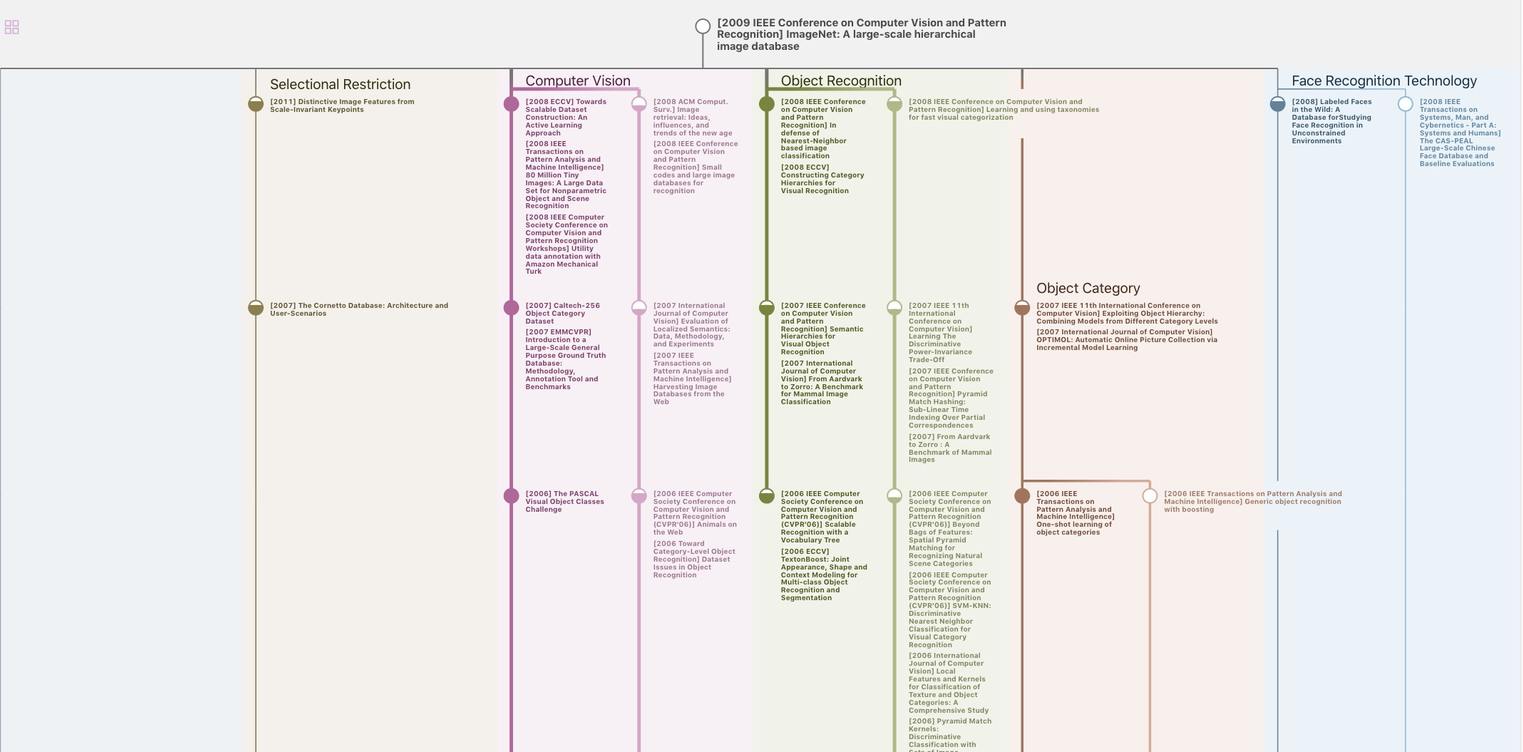
生成溯源树,研究论文发展脉络
Chat Paper
正在生成论文摘要