Deep Heterogeneous Multi-Task Metric Learning for Visual Recognition and Retrieval
MM '20: The 28th ACM International Conference on Multimedia Seattle WA USA October, 2020(2020)
摘要
How to estimate the distance between data instances is a fundamental problem in many artificial intelligence algorithms, and critical in diverse multimedia applications. A major challenge in the estimation is how to find an appropriate distance function when labeled data are insufficient for a certain task. Multi-task metric learning (MTML) is able to alleviate such data deficiency issue by learning distance metrics for multiple tasks together and sharing information between the different tasks. Recently, heterogeneous MTML (HMTML) has attracted much attention since it can handle multiple tasks with varied data representations. A major drawback of the current HMTML approaches is that only linear transformations are learned to connect different domains. This is suboptimal since the correlations between different domains may be very complex and highly nonlinear. To overcome this drawback, we propose a deep heterogeneous MTML (DHMTML) method, in which a nonlinear mapping is learned for each task by using a deep neural network. The correlations of different domains are exploited by sharing some parameters at the top layers of different networks. More importantly, the auto-encoder scheme and the adversarial learning mechanism are integrated and incorporated to help exploit the feature correlations in and between different tasks and the specific properties are preserved by learning additional task-specific layers together with the common layers. Experiments demonstrated that the proposed method outperforms single-task deep metric learning algorithms and other HMTML approaches consistently on several benchmark datasets.
更多查看译文
关键词
metric learning, multi-task, heterogeneous, deep neural networks, visual applications
AI 理解论文
溯源树
样例
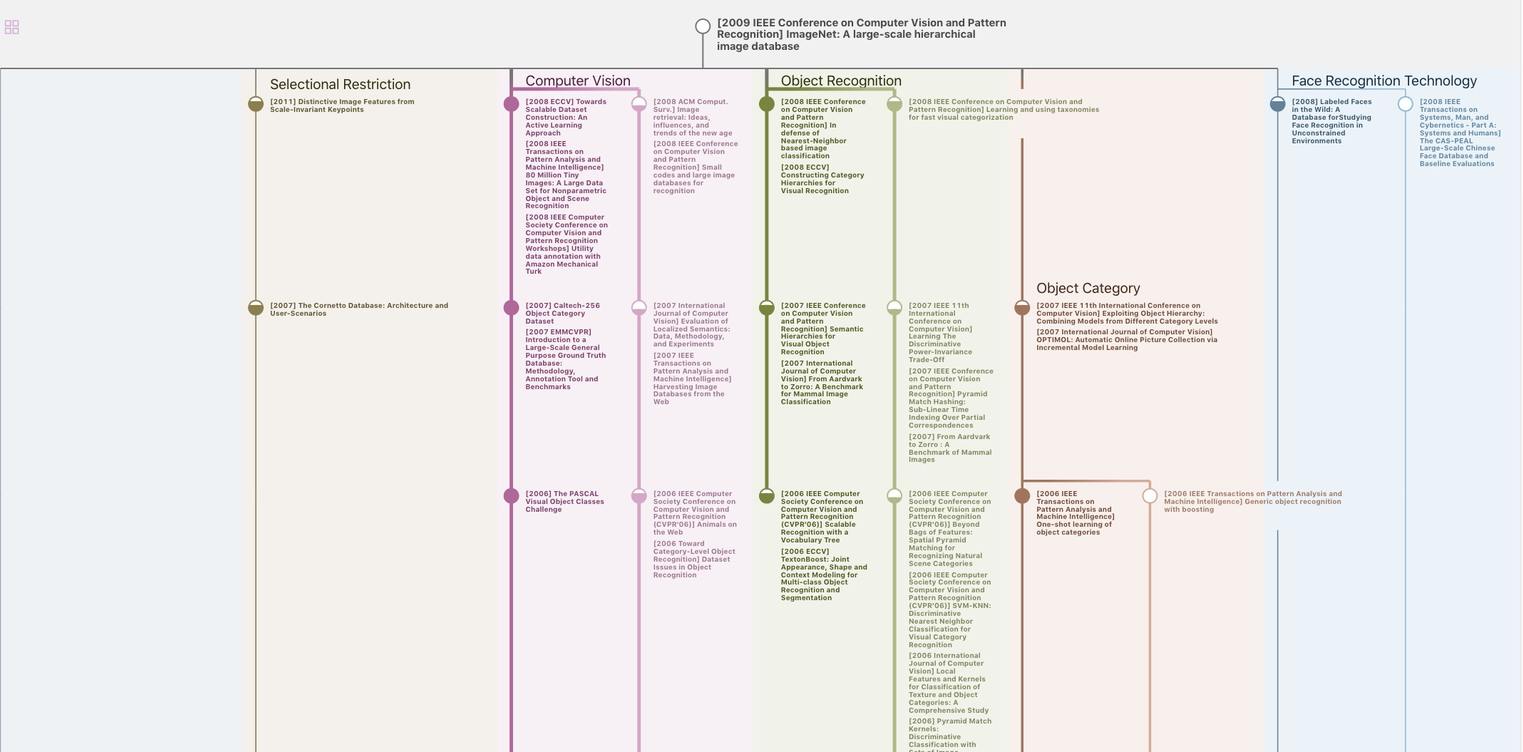
生成溯源树,研究论文发展脉络
Chat Paper
正在生成论文摘要