Meta-RCNN: Meta Learning for Few-Shot Object Detection
MM '20: The 28th ACM International Conference on Multimedia Seattle WA USA October, 2020(2020)
摘要
Despite significant advances in deep learning based object detection in recent years, training effective detectors in a small data regime remains an open challenge. This is very important since labelling training data for object detection is often very expensive and time-consuming. In this paper, we investigate the problem of few-shot object detection, where a detector has access to only limited amounts of annotated data. Based on the meta-learning principle, we propose a new meta-learning framework for object detection named "Meta-RCNN", which learns the ability to perform few-shot detection via meta-learning. Specifically, Meta-RCNN learns an object detector in an episodic learning paradigm on the (meta) training data. This learning scheme helps acquire a prior which enables Meta-RCNN to do few-shot detection on novel tasks. Built on top of the popular Faster RCNN detector, in Meta-RCNN, both the Region Proposal Network (RPN) and the object classification branch are meta-learned. The meta-trained RPN learns to provide class-specific proposals, while the object classifier learns to do few-shot classification. The novel loss objectives and learning strategy of Meta-RCNN can be trained in an end-to-end manner. We demonstrate the effectiveness of Meta-RCNN in few-shot detection on three datasets (Pascal-VOC, ImageNet-LOC and MSCOCO) with promising results.
更多查看译文
关键词
Object Detection, Deep Learning, Meta Learning
AI 理解论文
溯源树
样例
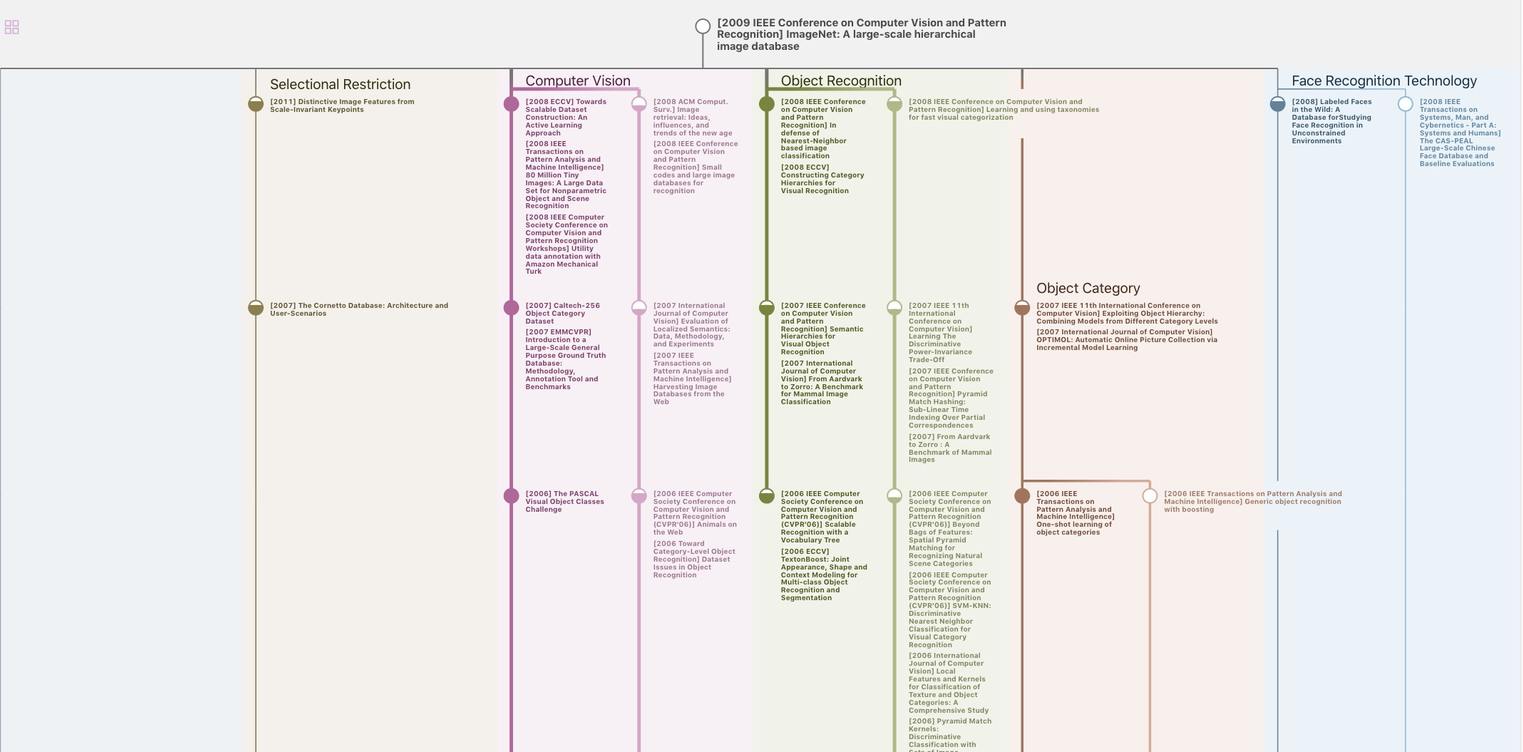
生成溯源树,研究论文发展脉络
Chat Paper
正在生成论文摘要