Siamese Attentive Graph Tracking
MM '20: The 28th ACM International Conference on Multimedia Seattle WA USA October, 2020(2020)
摘要
Recently, deep Siamese matching networks have attracted increasing attention for visual tracking. Despite the demonstrated successes, Siamese trackers do not take full advantage of the structural information of target objects. They tend to drift in the presence of non-rigid deformation or partly occlusion. In this paper, we propose to advance Siamese trackers with graph convolutional networks, which pay more attention to the structural layout of target objects, to learn features robust to large appearance changes over time. Specifically, we divide the target object into several sub-parts and design an attentive graph convolutional network to model the relationship between parts. We incrementally update the attention coefficients of the graph with the attention scheme at each frame in an end-to-end manner. To further improve localization accuracy, we propose a learnable cascade regression algorithm based on deep reinforcement learning to refine the predicted bounding boxes. Extensive experiments on seven challenging benchmark datasets, i.e., OTB-100, TC-128, VOT2018, VOT2019, TrackingNet, GOT-10k and LaSOT, demonstrate that the proposed tracking method performs favorably against state-of-the-art approaches.
更多查看译文
关键词
Siamese networks, visual tracking, attention, reinforcement learning
AI 理解论文
溯源树
样例
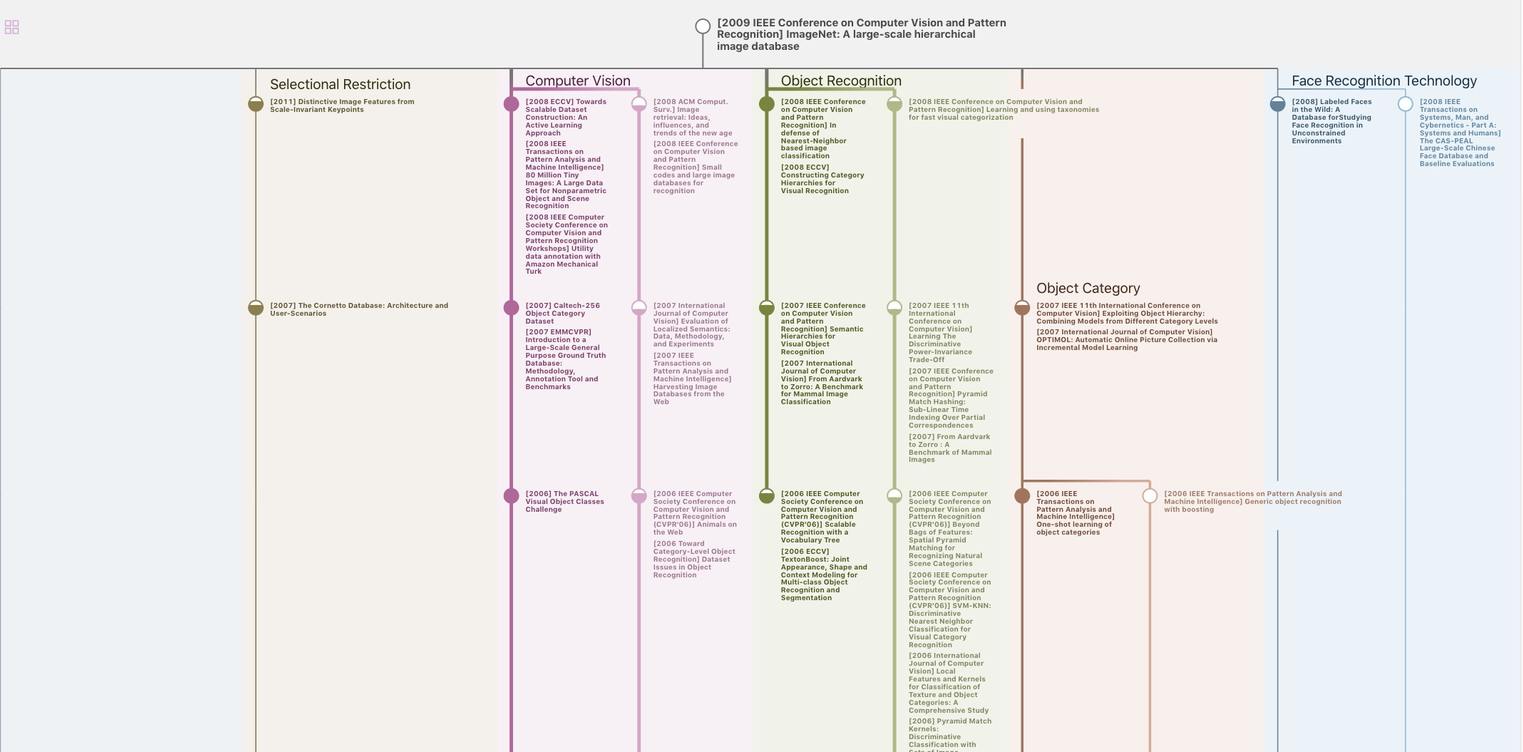
生成溯源树,研究论文发展脉络
Chat Paper
正在生成论文摘要